Cutting-edge AI models from Saama simplify radiology report generation, addressing critical bottlenecks in medical imaging.
- Innovative Integration: OpenBioLLM-8B, OpenBioLLM-70B, and DINOv2 vision encoders work together to generate accurate radiology reports from chest X-rays.
- Efficiency in Action: The new framework automates the “Findings” and “Impression” sections of reports, reducing the workload on radiologists while improving consistency.
- Future Potential: This approach lays the groundwork for scalable, AI-driven solutions in personalized medicine and clinical trials.
The field of radiology is witnessing a seismic shift with the advent of AI technologies capable of generating comprehensive reports from medical imaging. Saama’s latest models, OpenBioLLM-8B and OpenBioLLM-70B, coupled with advanced vision encoders like DINOv2, present a unified framework for automating the creation of critical radiology report sections. This breakthrough not only accelerates clinical workflows but also ensures consistency and accuracy in diagnostic interpretations.
The integration of visual and language models addresses a longstanding challenge in healthcare: the growing volume of medical imaging data versus the limited number of radiologists. This imbalance necessitates innovative solutions like AI-driven tools to assist radiologists in managing their workload efficiently.
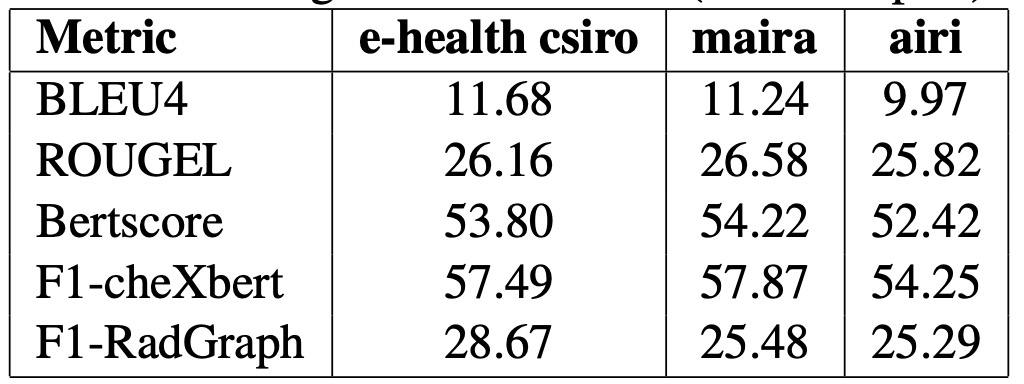
How OpenBioLLM and DINOv2 Work Together
At the heart of this innovation lies the combination of DINOv2 vision encoders and OpenBioLLM language models. The DINOv2 encoder analyzes X-ray images to extract meaningful visual features, while the OpenBioLLM text generation framework translates these features into detailed and clinically relevant narratives.
This approach employs the LLaVA framework to seamlessly integrate image processing and text generation. By training on large-scale datasets like MIMIC-CXR, the system ensures its outputs are grounded in real-world medical data. Prompts such as “Write findings for this X-ray” guide the AI to produce precise and actionable sections for radiology reports.
Key Achievements and Challenges
The results are impressive. OpenBioLLM-8B demonstrated superior performance compared to previous models, offering detailed and coherent report sections. Using a mix of supervised training and PEFT (parameter-efficient fine-tuning) methods like LoRA, the model overcame challenges like overfitting, ensuring robust outputs across diverse imaging scenarios.
However, the system isn’t without limitations. Current implementations don’t support personalized or dynamic backgrounds in sequential image generation, and occasional “copy-paste” effects in outputs highlight areas for further refinement. Future iterations aim to address these issues by enhancing data augmentation techniques and incorporating more advanced generative frameworks.
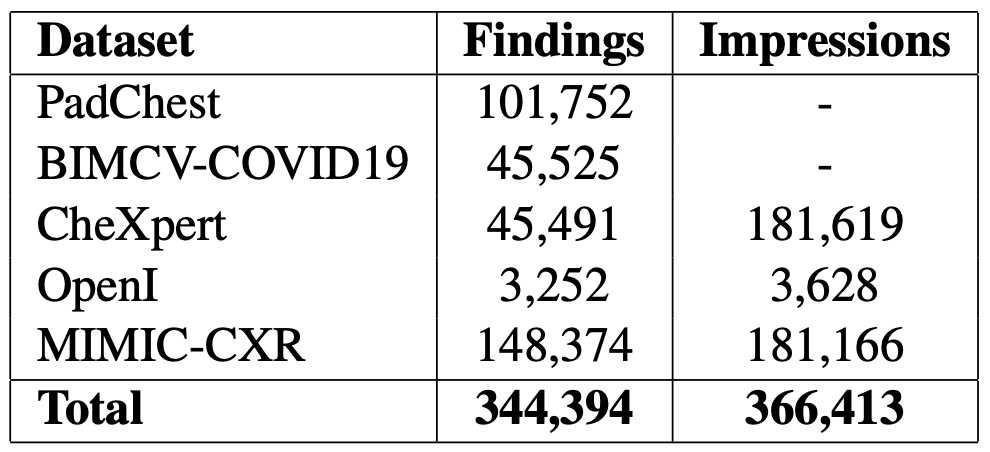
A Vision for the Future of AI in Medicine
The integration of vision and language AI models like OpenBioLLM is poised to revolutionize personalized medicine and clinical research. By automating tedious yet critical tasks, these tools allow radiologists and clinicians to focus on more nuanced aspects of patient care.
This breakthrough also serves as a blueprint for other applications of AI in healthcare, from streamlining clinical trials to improving diagnostic accuracy across medical domains. With further development, OpenBioLLM and similar frameworks could redefine the role of AI in medicine, bridging the gap between innovation and practical clinical utility.
In sum, the collaboration between advanced AI models and medical data represents a new frontier in healthcare — one where technology and medicine work hand-in-hand to improve outcomes and streamline care.