Redefining AI with scalable pre-training for images and text integration.
- Apple introduces AIMV2, a family of large-scale vision encoders excelling in multimodal tasks.
- AIMV2 leverages autoregressive pre-training to reconstruct image patches and text tokens, achieving state-of-the-art results.
- Its scalability and superior performance highlight AIMV2’s potential to redefine multimodal AI applications.
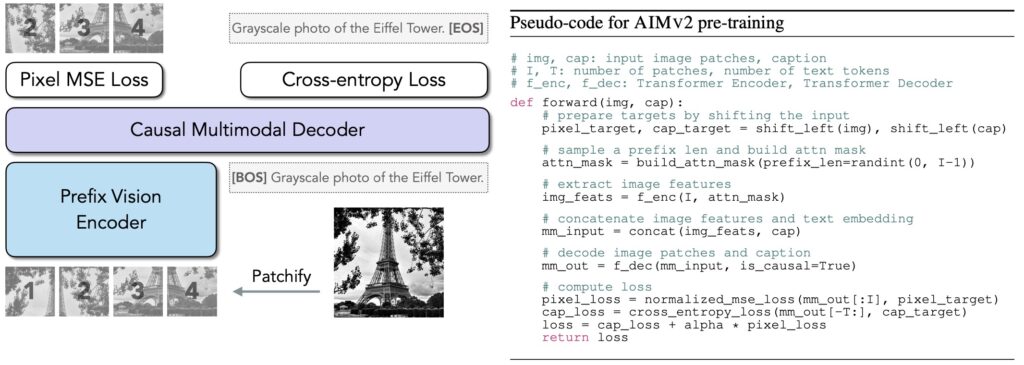
Apple’s AIMV2 marks a significant leap in the field of artificial intelligence, blending vision and language understanding through a multimodal autoregressive framework. Unlike traditional models, AIMV2 bridges the gap between text and images by training vision encoders to reconstruct raw image patches and text tokens. This innovative approach allows AIMV2 to excel in a variety of downstream tasks, from image classification to multimodal understanding.
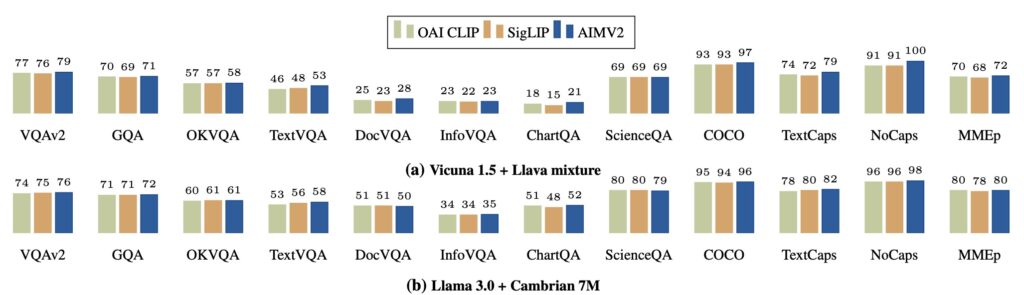
By integrating vision encoders with multimodal decoders, AIMV2 achieves exceptional accuracy across benchmarks. For example, the AIMV2-3B model records an impressive 89.5% accuracy on the ImageNet-1k dataset, outpacing many state-of-the-art models.
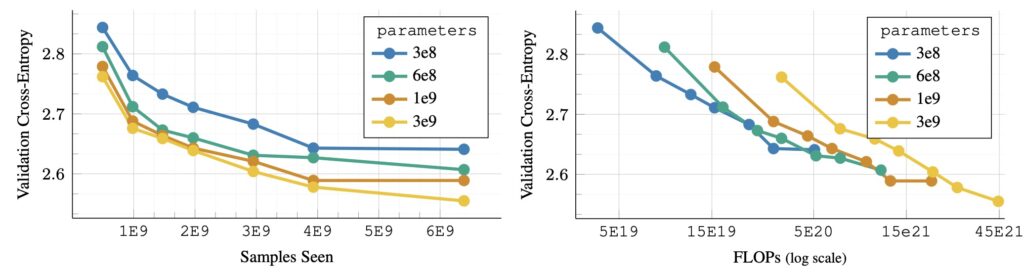
Breaking Boundaries in Multimodal AI
AIMV2 shines in its ability to perform across diverse tasks. It surpasses leading models like CLIP and SigLIP in multimodal image understanding and offers remarkable results in vision-specific tasks such as grounding and classification. The model’s strength lies in its unified objective—reconstructing input patches and tokens—that optimally utilizes available data for efficient training.
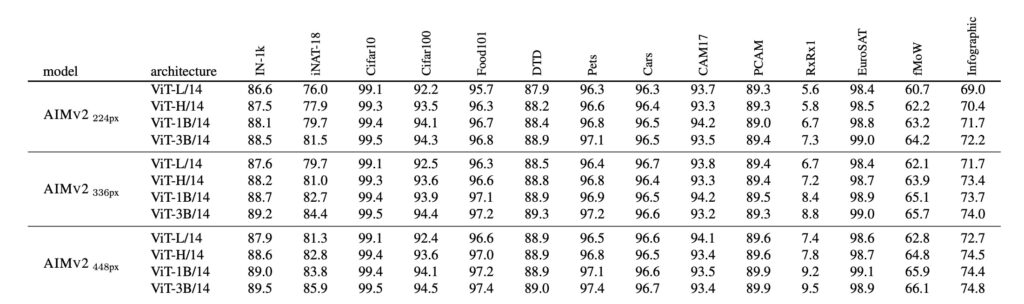
What sets AIMV2 apart is its adaptability. Unlike contrastive learning models that require extensive datasets, AIMV2’s autoregressive approach ensures high-quality training with fewer samples. This efficiency opens new doors for scaling vision encoders while maintaining exceptional performance.
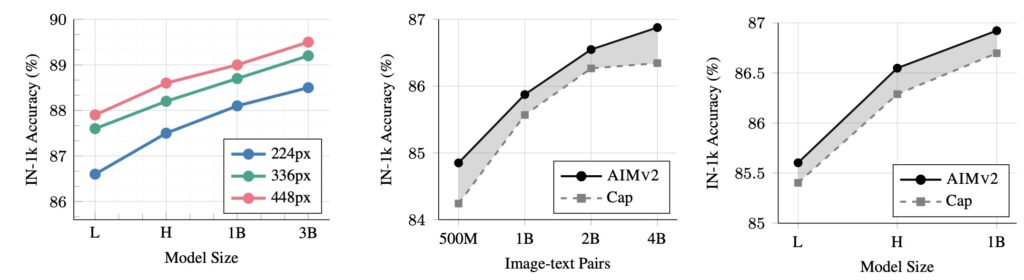
AIMV2’s Performance Highlights
- ImageNet-1k Classification: The AIMV2-3B model attains 89.5% accuracy with a frozen encoder.
- Multimodal Understanding: Outperforms models like CLIP in complex tasks involving text-image integration.
- Efficiency and Scalability: Simplified pre-training ensures AIMV2 remains scalable for future advancements.
These accomplishments underscore AIMV2’s potential as a game-changer in AI-driven vision and language applications.
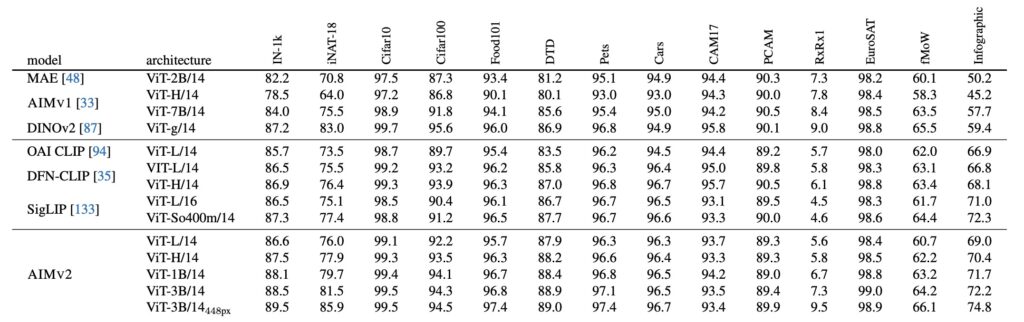
Paving the Way for Scalable Vision Models
Apple’s AIMV2 doesn’t just represent a new model—it’s a rethinking of how AI approaches vision and multimodal learning. Its autoregressive framework, paired with its scalability, positions it as a powerful tool for researchers and developers. As Apple continues to refine this technology, AIMV2 paves the way for robust applications in AI, from autonomous systems to next-generation user experiences.
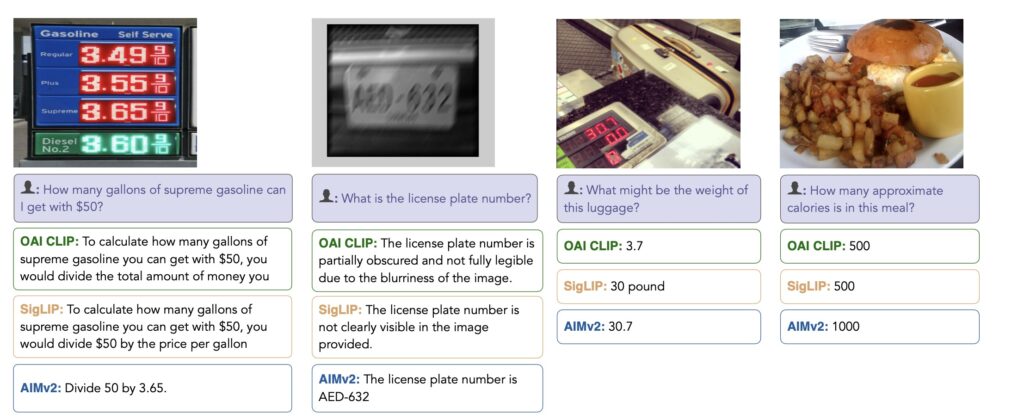
By advancing the boundaries of multimodal AI, AIMV2 stands as a testament to the potential of combining simplicity, efficiency, and innovation in one groundbreaking framework.
