Integrating pixel-level understanding with powerful reasoning for advanced multimodal interactions
- Unified Model Architecture: OMG-LLaVA combines image-level, object-level, and pixel-level reasoning within a single framework, enhancing both visual understanding and reasoning capabilities.
- Perception Prior Embedding: The model integrates perception priors with image features for improved pixel-level segmentation and understanding.
- Flexible User Interaction: OMG-LLaVA accepts diverse visual and text prompts, offering flexible and interactive user experiences across multiple tasks.
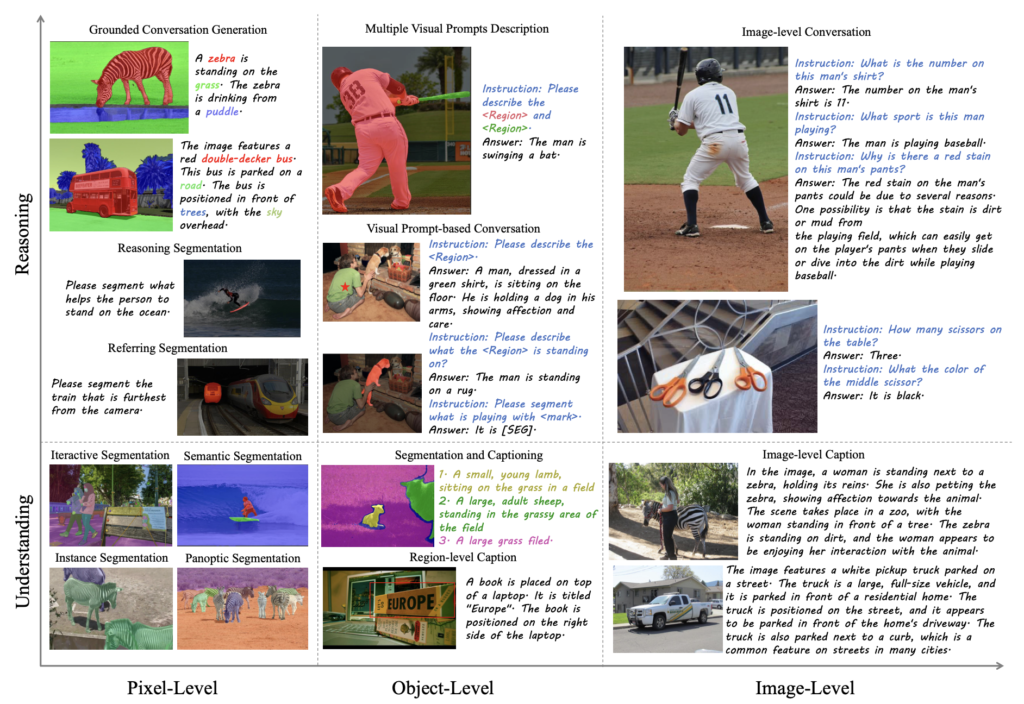
In a significant leap for artificial intelligence, researchers have introduced OMG-LLaVA, a novel framework that bridges the capabilities of pixel-level image understanding and vision-based reasoning within a single model. This innovative approach seeks to overcome the limitations of current universal segmentation methods and large vision-language multimodal models, which traditionally excel in their respective areas but lack comprehensive integration.
The Challenge
Current universal segmentation methods are adept at pixel-level image and video understanding, yet they fall short in reasoning capabilities and lack flexibility in text-based control. On the other hand, large vision-language multimodal models, while powerful in vision-based conversation and reasoning, struggle with pixel-level understanding and are not designed to handle visual prompts effectively for user interaction.
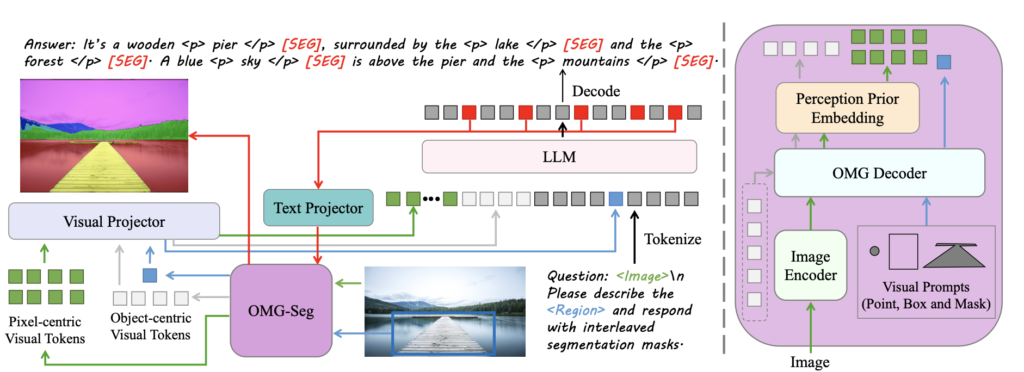
The Solution: OMG-LLaVA
OMG-LLaVA addresses these challenges by unifying the strengths of both approaches. This framework employs a universal segmentation method as its visual encoder, effectively integrating image information, perception priors, and visual prompts into visual tokens that are processed by a large language model (LLM). The LLM then interprets the user’s text instructions and generates both text responses and pixel-level segmentation results based on the visual input.
Key Innovations
- Unified Architecture: OMG-LLaVA simplifies the model architecture by using one image encoder, one LLM, and one decoder. This end-to-end training approach minimizes the need for multiple specialized models, reducing the overall complexity and computational cost.
- Perception Prior Embedding: The model incorporates perception prior embedding, a technique designed to integrate perception priors with image features. This enhancement allows for more accurate and contextually aware pixel-level segmentation and understanding.
- Versatile User Interaction: By accepting a variety of visual and text prompts, OMG-LLaVA offers flexible and interactive user experiences. Users can engage with the model through different types of input, making it a versatile tool for various applications.
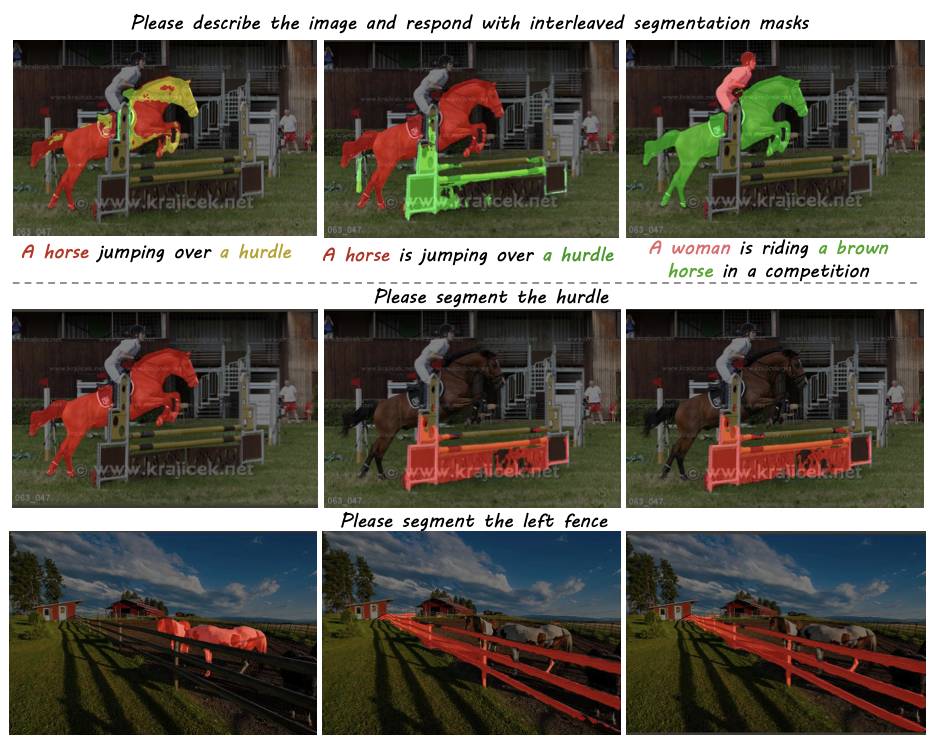
Performance and Capabilities
OMG-LLaVA excels in performing image-level, object-level, and pixel-level reasoning and understanding, matching or surpassing the performance of specialized methods across multiple benchmarks. The model demonstrates the ability to handle over eight different multimodal learning tasks while preserving the visual perception capabilities of its predecessor, OMG-Seg.
Implications for AI Development
The development of OMG-LLaVA represents a significant step forward in the design of multimodal language models (MLLMs). By unifying various levels of understanding and reasoning within a single framework, the model paves the way for more efficient and capable AI systems. The research team hopes that this work will inspire the AI community to rethink the design of MLLM architectures, aiming to maximize functionality while minimizing the number of model components.
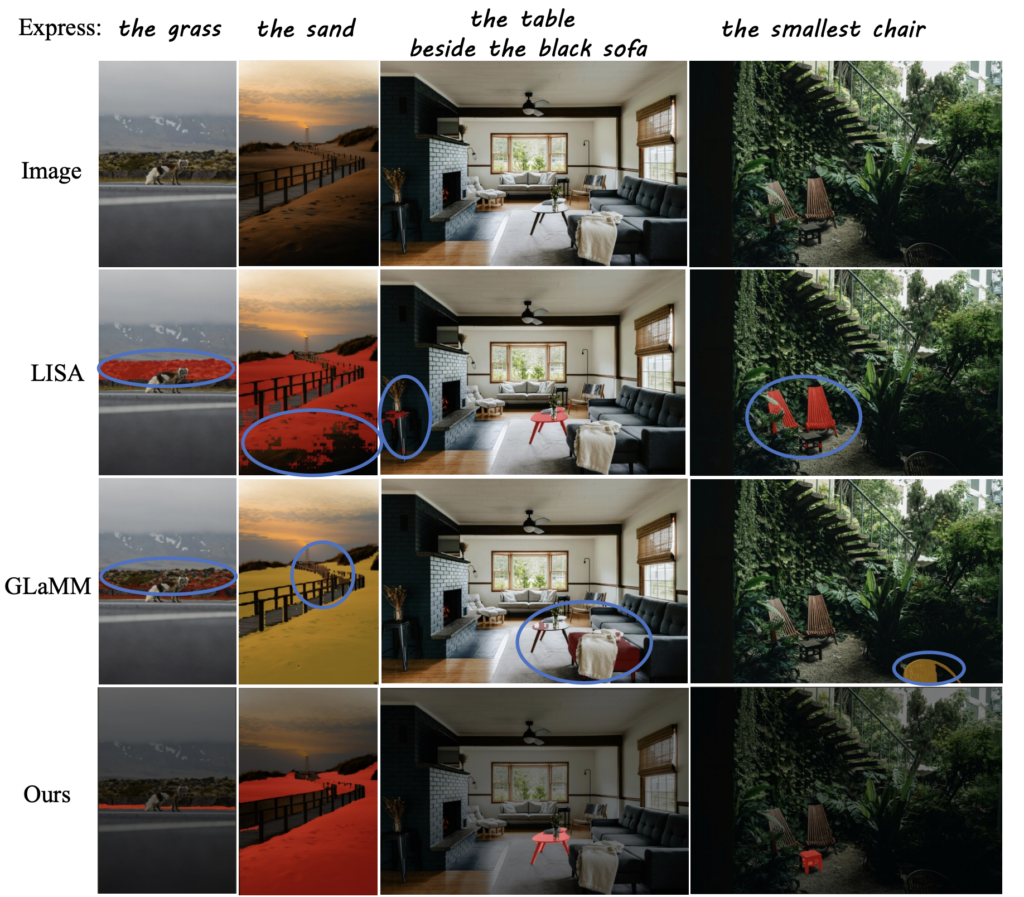
Future Directions
The code and model for OMG-LLaVA have been released to the public, encouraging further research and development. As the AI community continues to explore and refine this approach, the potential applications for such advanced multimodal models are vast, ranging from enhanced interactive systems to more sophisticated image and video analysis tools.
OMG-LLaVA stands as a testament to the evolving capabilities of AI in integrating comprehensive visual understanding with advanced reasoning. By bridging the gap between pixel-level accuracy and vision-based reasoning, this innovative framework offers a glimpse into the future of AI, where flexible, interactive, and highly capable systems can seamlessly interpret and respond to both visual and textual inputs.