A family of large-scale language models pushing the boundaries of efficiency and performance
The study introduces Cerebras-GPT, a groundbreaking family of open compute-optimal language models, scaling from 111 million to 13 billion parameters. These models are trained on the Eleuther Pile dataset, following DeepMind Chinchilla scaling rules to ensure efficient pre-training and high accuracy within a given compute budget.
When compared to other open-source models, Cerebras-GPT demonstrates state-of-the-art pre-training efficiency on both pre-training and downstream objectives. This research is the first open effort of its kind, providing detailed instructions for reproducing the results and releasing pre-trained model checkpoints.
The study also incorporates Maximal Update Parameterization (μP), a technique that improves large model stability and enhances scaling results. The researchers document their experience in training these models on the Andromeda AI Cluster, which consists of 16 Cerebras CS-2 systems, showcasing the simplicity of scaling models and performance.
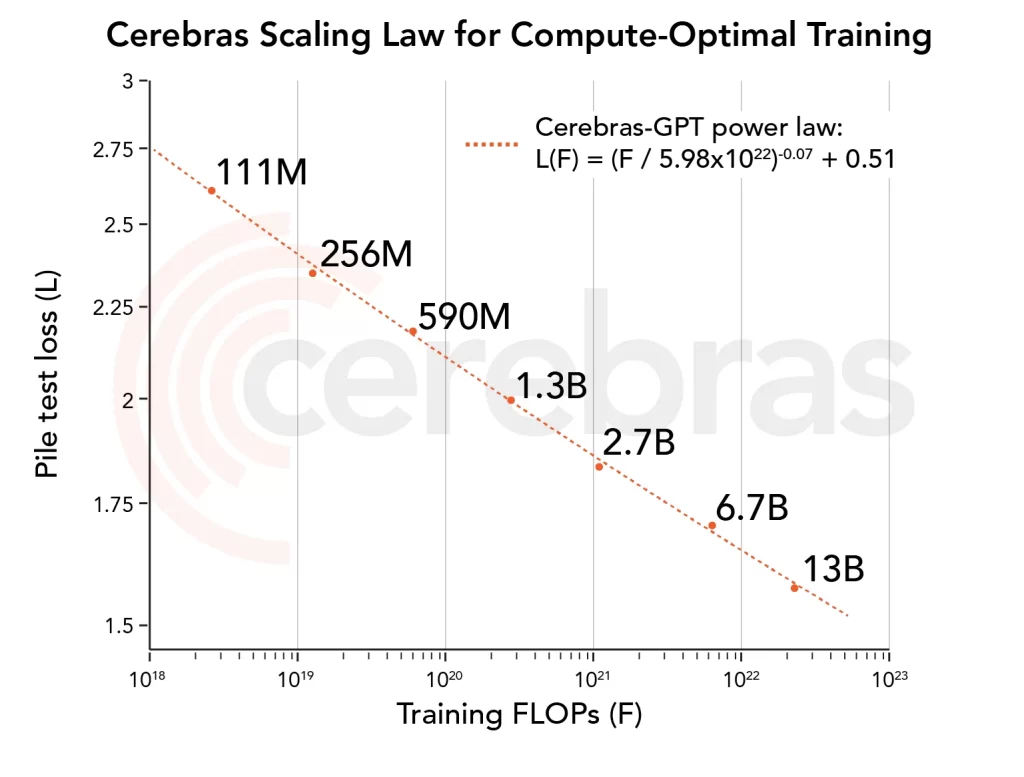
Overall, Cerebras-GPT represents a significant advancement in the development of open compute-optimal language models, pushing the boundaries of efficiency and performance in the field of artificial intelligence.