Innovating Object Addition in Images with Text Guidance Alone
- Diffree enables seamless text-guided object addition without compromising background consistency.
- The model leverages the OABench dataset, enhancing training and performance in real-world scenarios.
- Diffree eliminates the need for cumbersome human intervention, using a diffusion model with an object mask predictor.
Object addition in images, driven solely by text guidance, has long posed a significant challenge in the field of computer vision. Existing methods often struggle with maintaining the consistency of the visual context, such as lighting, texture, and spatial location, and typically require extensive human intervention. Addressing these challenges, researchers have introduced Diffree, a novel Text-to-Image (T2I) model that seamlessly integrates new objects into images using only text input.
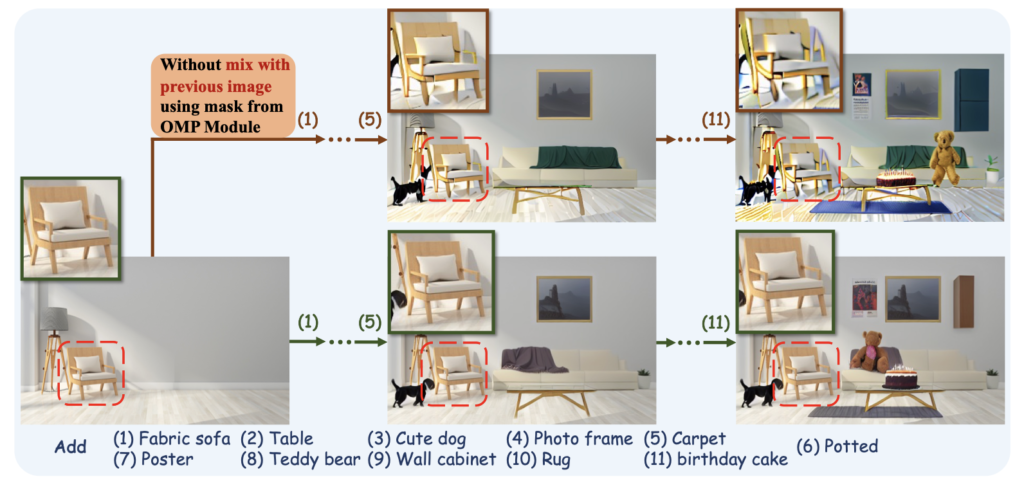
The Challenge of Text-Guided Object Addition
Traditional text-guided image inpainting methods can add objects to images but often at the expense of background consistency. These methods may require users to manually specify bounding boxes or scribble masks, which is not only cumbersome but also prone to errors. The main challenge lies in ensuring that the newly added object blends perfectly with the existing image in terms of lighting, texture, and spatial arrangement.
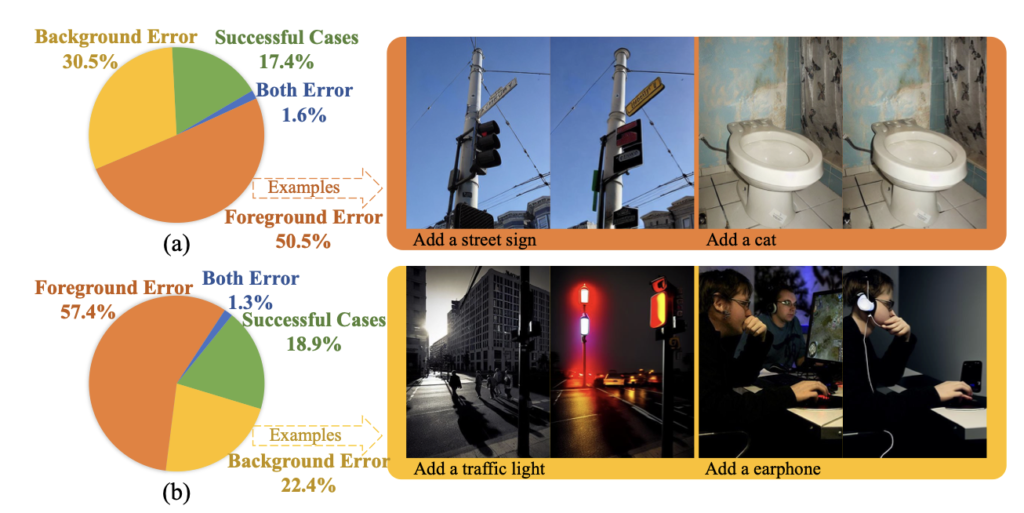
Introducing Diffree
Diffree addresses these challenges by leveraging a diffusion model coupled with an object mask predictor. This innovative approach allows for the seamless addition of objects into images with guidance from text alone. The model’s training is bolstered by OABench, a meticulously curated synthetic dataset designed specifically for this task.
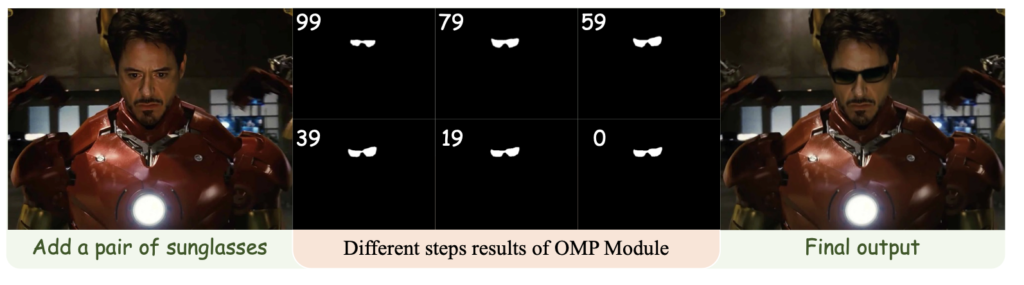
OABench Dataset:
- Composition: OABench consists of 74,000 real-world tuples, each containing an original image, an inpainted image with an object removed, an object mask, and detailed object descriptions.
- Creation: The dataset is created using advanced image inpainting techniques to remove objects, ensuring a high-quality foundation for training the Diffree model.
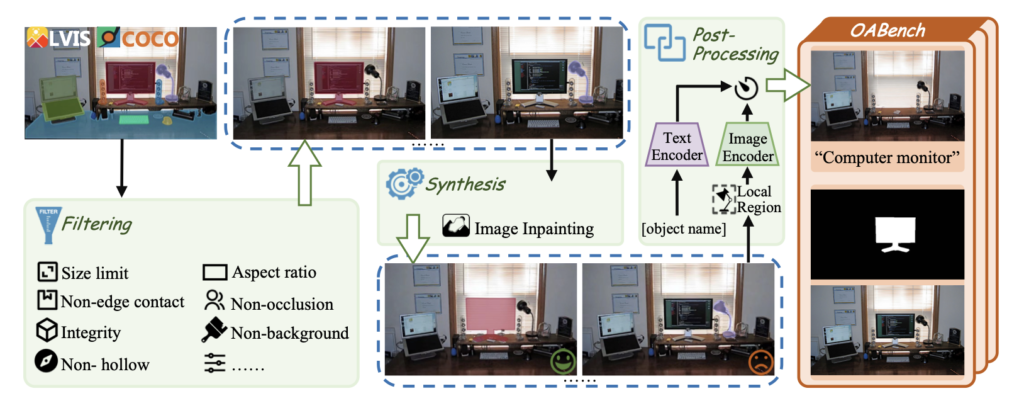
Training and Functionality:
- Stable Diffusion Model: Diffree is trained on the Stable Diffusion model, integrated with an additional mask prediction module.
- Text Guidance: The model predicts the position and integrates new objects into images purely based on textual descriptions provided by the user.
- Background Consistency: One of Diffree’s standout features is its ability to maintain background consistency without the need for external masks or manual input.
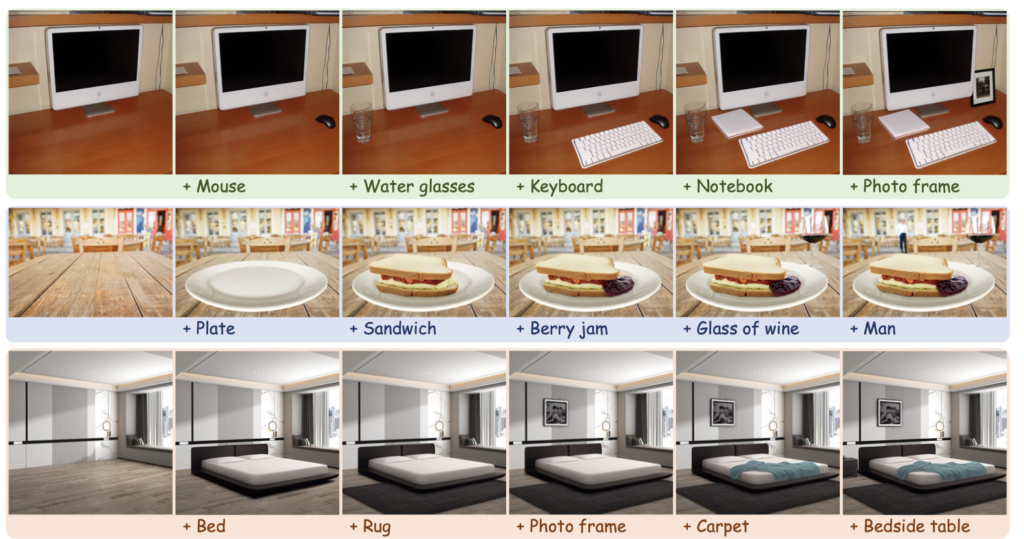
Performance and Applications
Extensive experiments have demonstrated Diffree’s ability to add new objects into images with a high success rate. The model excels in maintaining background consistency, spatial appropriateness, and object relevance and quality, setting a new benchmark in the field of text-guided image inpainting.
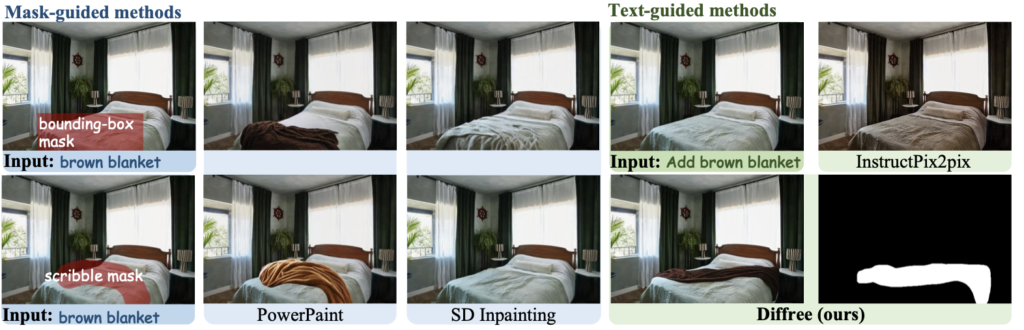
Quantitative and Qualitative Results:
- High Success Rate: Diffree consistently achieves successful object addition, validated through both quantitative metrics and qualitative assessments.
- Background Consistency: The model ensures that the background remains intact and visually coherent, a common shortcoming in previous methods.
- Object Quality: Added objects are of high quality, seamlessly integrated into the scene with appropriate lighting and texture.
Eliminating Human Intervention
One of the most significant advantages of Diffree is the elimination of cumbersome human intervention. Unlike traditional methods that require users to specify bounding boxes or scribble masks, Diffree operates solely on text input. This advancement not only simplifies the user experience but also enhances the model’s practicality and scalability.
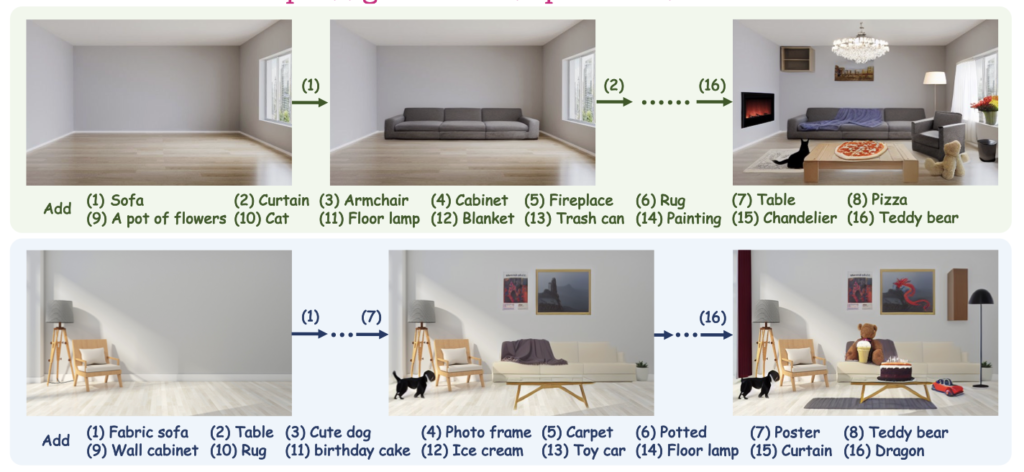
User Experience:
- Text Input: Users provide textual descriptions of the objects they wish to add, and Diffree handles the rest.
- Automation: The model automates the process of object addition, making it accessible to users without technical expertise.
Future Directions
While Diffree marks a significant advancement, there are areas for future exploration and improvement. Enhancing the model’s ability to handle more complex scenes and integrating additional features such as multi-object addition and real-time processing could further elevate its capabilities.
Potential Improvements:
- Complex Scenes: Extending the model’s functionality to handle more intricate scenes with multiple objects and dynamic backgrounds.
- Real-Time Processing: Developing real-time capabilities for instant object addition based on text input.
Diffree represents a groundbreaking approach to text-guided object addition in images, offering a seamless and automated solution that maintains visual consistency and quality. By leveraging the comprehensive OABench dataset and eliminating the need for manual input, Diffree sets a new standard for image inpainting, making it accessible and practical for a wide range of applications. As the model continues to evolve, it holds the potential to revolutionize how we interact with and manipulate visual content, paving the way for more intuitive and efficient image editing tools.