Exploring the Role of Synthetic Captions and AltTexts in Pre-Training Multimodal Foundation Models
- Hybrid Captioning Approach: A combination of synthetic captions and original AltTexts is shown to improve model performance and alignment, addressing the limitations of relying solely on synthetic captions.
- Model-Specific Preferences: Different multimodal foundation models, such as CLIP and multimodal LLMs, exhibit unique preferences for caption formats, suggesting that a one-size-fits-all approach may not be effective.
- Controllable Captioning Pipeline: The development of a scalable captioning pipeline allows for the generation of diverse caption formats, enhancing the training process for various models.
In the rapidly evolving landscape of artificial intelligence, the intersection of image and text data has become crucial for training effective multimodal foundation models. Recent studies have pointed to the potential benefits of synthetic captions in enhancing image-text alignment, but questions remain about their relationship with traditional web-crawled AltTexts. This research seeks to bridge that gap by exploring the interactions between synthetic captions and AltTexts, providing valuable insights for optimizing pre-training strategies in multimodal models.
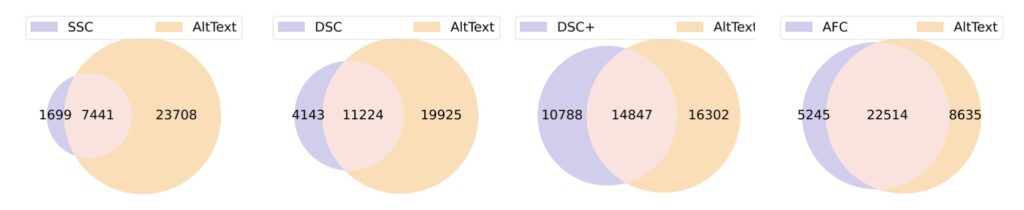
The study introduces a novel, controllable, and scalable captioning pipeline designed to generate a diverse range of caption formats tailored to the needs of various multimodal models. By examining Short Synthetic Captions (SSC) and Dense Synthetic Captions (DSC+) as case studies, the researchers systematically investigate how these caption types interact with original AltTexts across different models, including CLIP and multimodal LLMs. The findings underscore the importance of a hybrid approach, where both synthetic captions and AltTexts are utilized, leading to improved model performance and alignment.
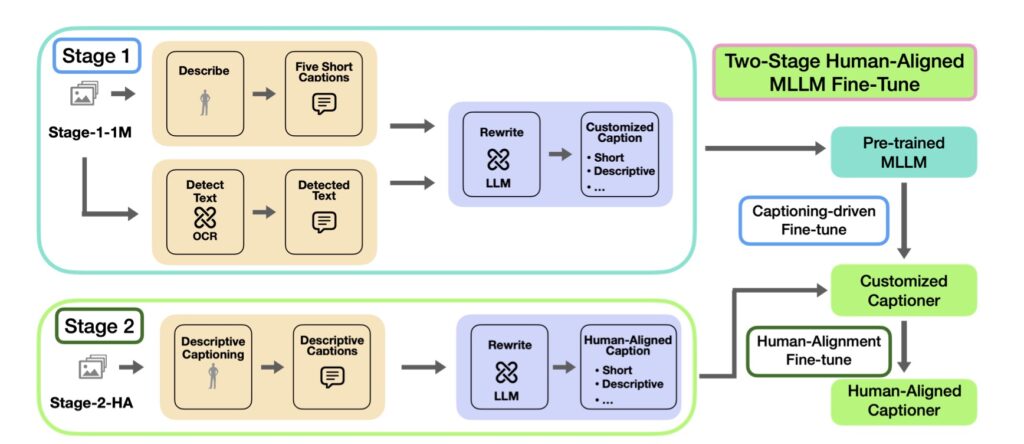
One of the key insights from this research is the distinct preferences exhibited by different multimodal foundation models when it comes to caption types. For instance, CLIP demonstrates a tendency to favor short synthetic captions, while multimodal LLMs show better performance with more descriptive captions. This variation suggests that optimizing caption formats for specific models can significantly impact training outcomes. Moreover, the research highlights that the benchmarks used in the pre-training and fine-tuning stages of multimodal LLMs may have different preferences for captioning, indicating a need for tailored strategies.
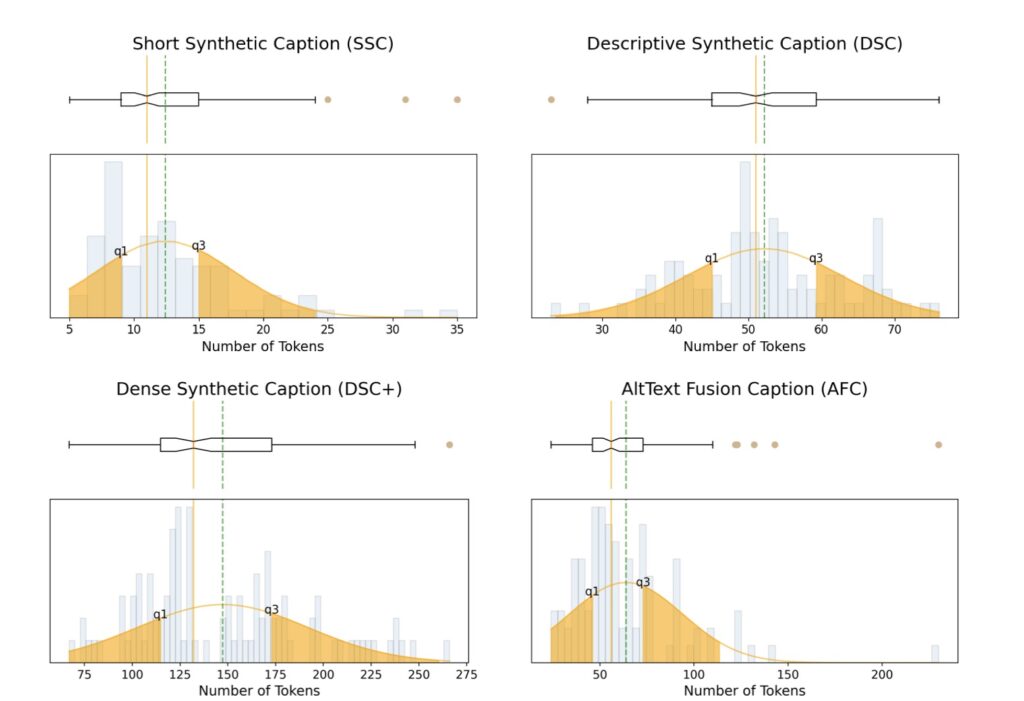
Additionally, the study reaffirms observations from previous models, such as DALLE-3, regarding the advantages of synthetic captions in text-to-image generation tasks. By leveraging a comprehensive set of benchmarks, the researchers provide evidence that integrating synthetic captions into the training process enhances overall performance. This further solidifies the case for a hybrid captioning strategy that balances the strengths of both synthetic and original AltTexts.
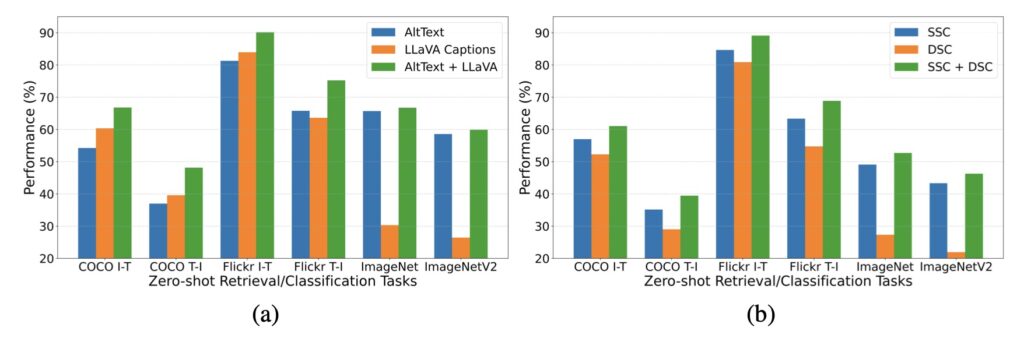
As the research community continues to explore the complexities of multimodal models, the insights gained from this study pave the way for future advancements. The authors express their intention to refine the captioning pipeline further, aiming to enhance its ability to generate task-specific captions applicable across a broader range of multimodal applications. This ongoing evolution is vital for maximizing the effectiveness of multimodal models, ultimately contributing to more robust and versatile AI systems.
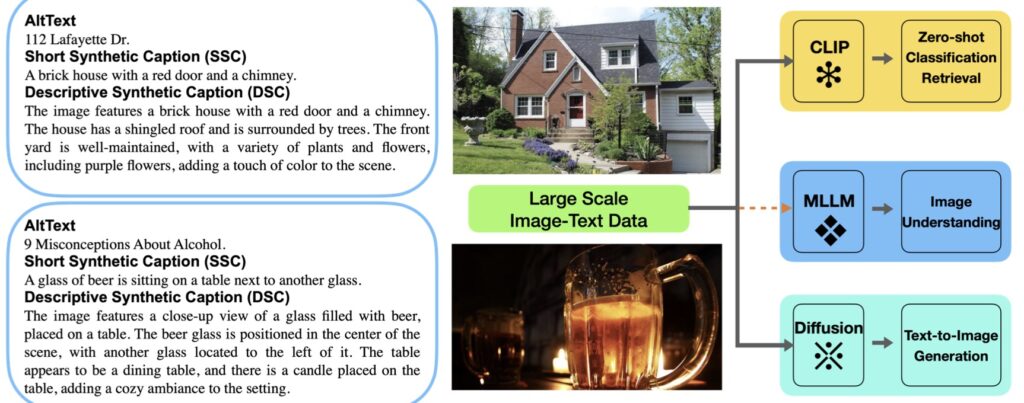
The study on prompt-adaptive captioning in multimodal foundation models emphasizes the critical role that both synthetic captions and AltTexts play in training effective AI systems. By adopting a hybrid approach that tailors caption formats to model preferences, developers and researchers can optimize the performance of their models, leading to improved image-text alignment and overall outcomes. As advancements in multimodal AI continue, the findings from this research will serve as a valuable guide for future endeavors in the field.