Transforming AI Interpretability Through Improved Training Techniques
In the rapidly evolving field of artificial intelligence, the ability to reason through complex visual and linguistic tasks is paramount. Recent advancements in vision-language models (VLMs) have highlighted the significance of chain-of-thought (CoT) reasoning in enhancing model interpretability and trustworthiness.
- Addressing Data Limitations: Current training datasets for VLMs predominantly feature short answers with minimal rationales, which restricts the models’ ability to generalize to complex reasoning tasks.
- Innovative Training Approaches: The proposed methodology introduces distilled rationales from the GPT-4o model and utilizes reinforcement learning to refine VLM reasoning capabilities, leading to substantial performance improvements.
- Empirical Success: Experimental results demonstrate significant enhancements in CoT reasoning on benchmark datasets, suggesting that enriched training data and reinforcement learning can markedly improve VLM performance in reasoning and prediction tasks.
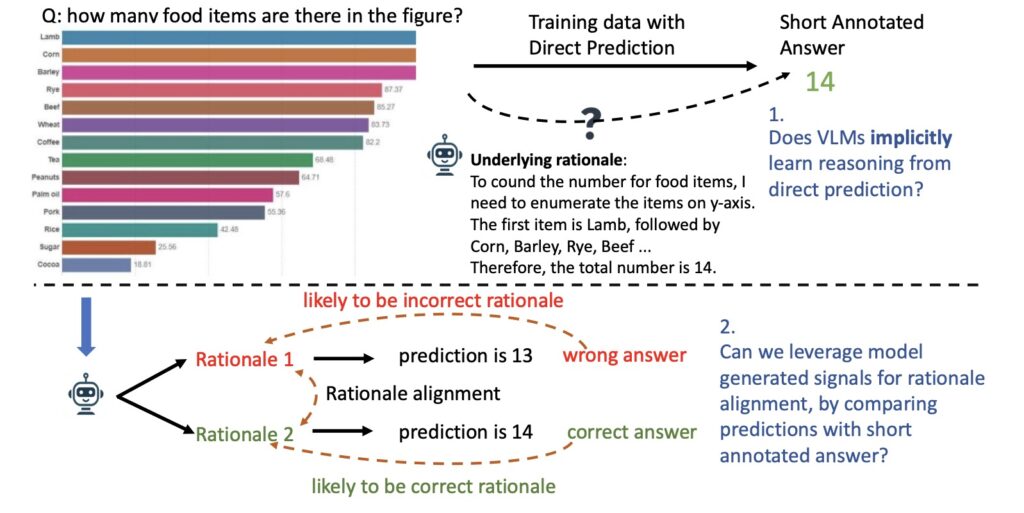
Chain-of-thought reasoning is essential for enabling vision-language models to navigate intricate tasks, providing a pathway to enhanced interpretability and trustworthiness. As VLMs are increasingly deployed for complex applications, the need for models to exhibit strong reasoning capabilities becomes vital. However, the existing training practices often rely on datasets that prioritize short answers with limited rationales, creating a gap in the models’ ability to generalize and perform well in reasoning tasks.
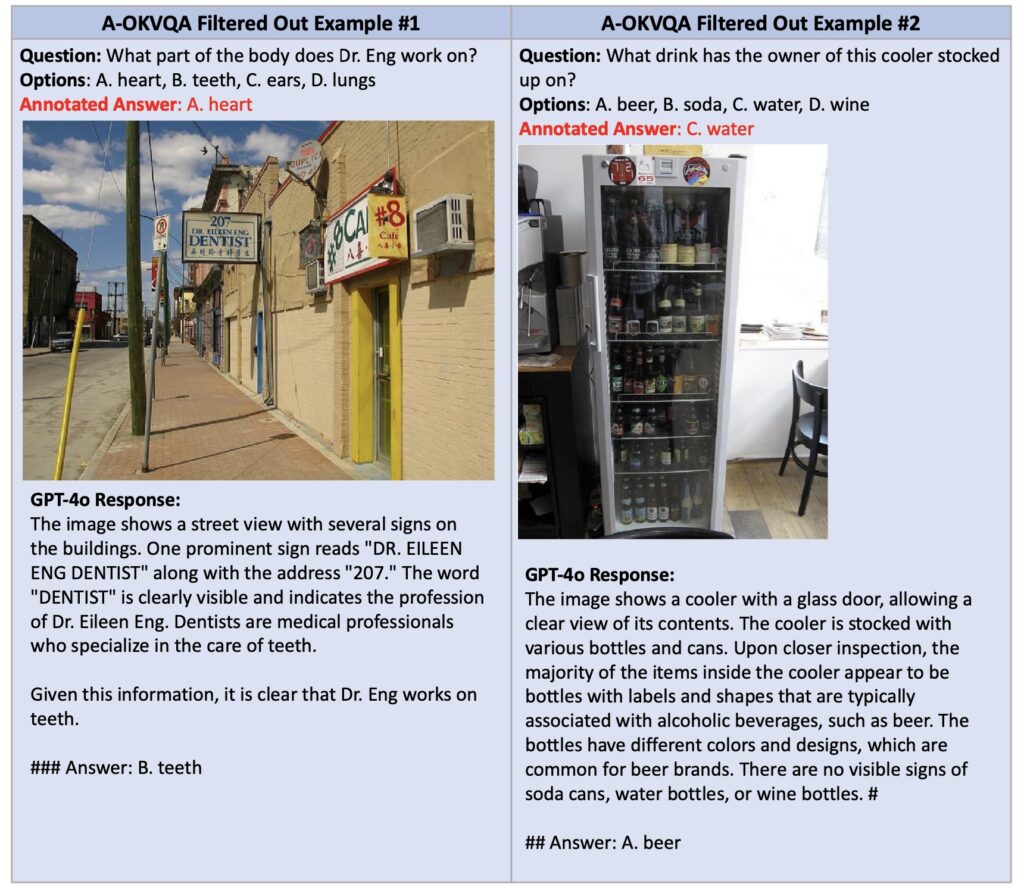
For instance, consider a scenario where a VLM must analyze a bar graph to determine the number of food items present. A human would naturally enumerate the bars and perform calculations to arrive at the answer. Yet, the annotated training data for such tasks typically consists of succinct responses, which do not capture the detailed reasoning process. This raises an important question: does training on straightforward predictions adequately prepare the model for more complex chain-of-thought reasoning?
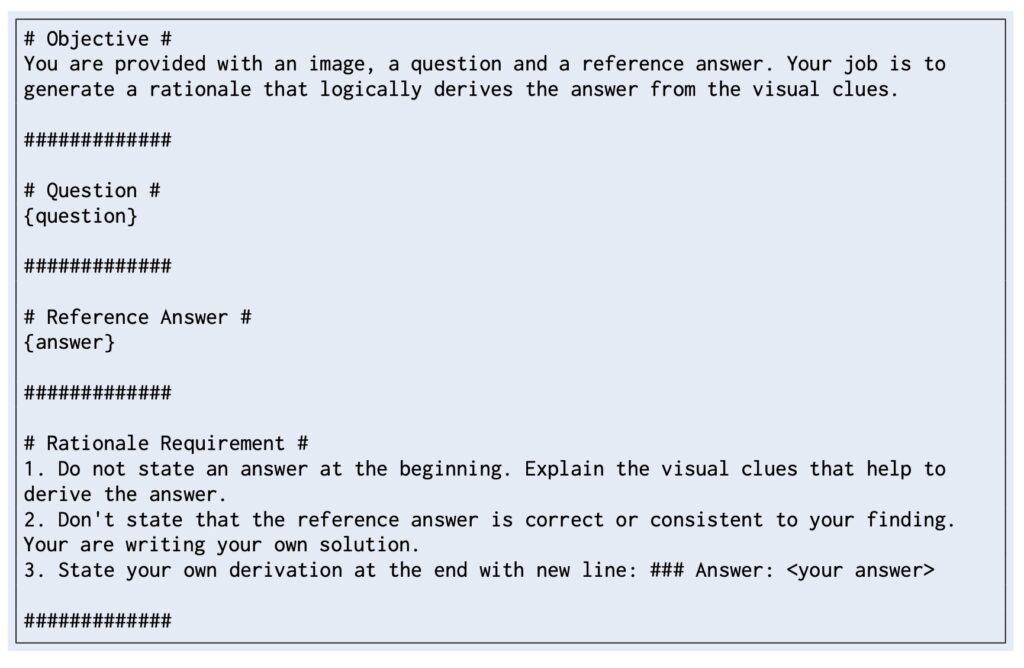
Innovative Solutions to Enhance VLM Performance
To address the limitations of current training methodologies, this research proposes a two-fold approach aimed at enriching the training data for vision-language models. The first strategy involves distilling detailed rationales from the GPT-4o model, which serves to provide a more comprehensive dataset for training. This enriched dataset, referred to as SHAREGPT-4O-REASONING, encompasses a wide range of visual question-answering (VQA) tasks, significantly enhancing the models’ reasoning capabilities.
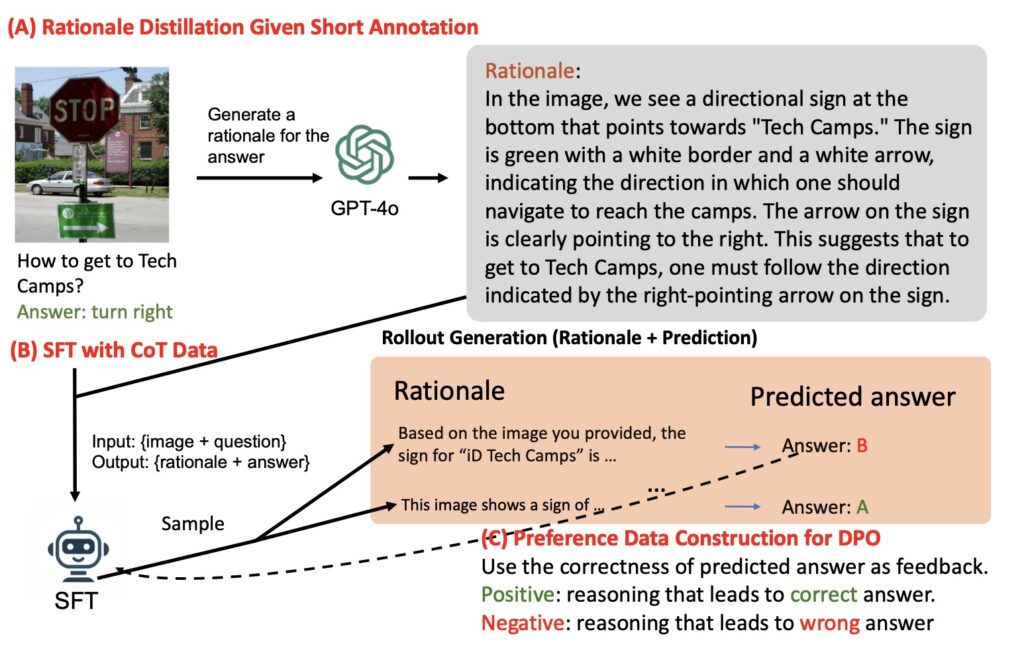
The second aspect of this approach employs reinforcement learning (RL) techniques to further refine the reasoning quality of VLMs. By creating positive (correct) and negative (incorrect) pairs of model-generated reasoning chains and comparing them to annotated short answers, the study implements Direct Preference Optimization (DPO) to calibrate the models’ reasoning abilities more effectively.
Empirical Validation of Enhanced Training Techniques
Experimental results from this research indicate substantial improvements in chain-of-thought reasoning after the implementation of these novel training methods. When fine-tuning VLMs on the enriched SHAREGPT-4O-REASONING dataset, researchers observed marked enhancements in reasoning performance across various benchmark datasets. Moreover, the application of reinforcement learning techniques further strengthened the models’ capabilities to generalize to direct answer prediction tasks.
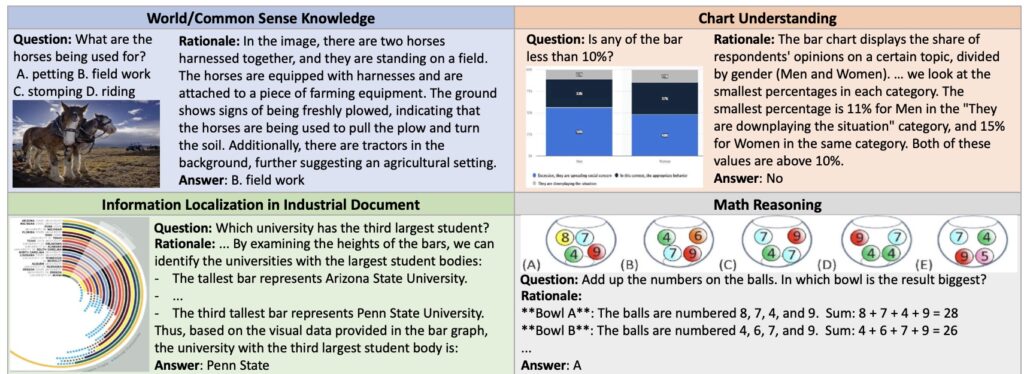
For instance, prior training on 26,000 direct predictions from ChartQA improved accuracy for direct answers by 2.9 points, while the improvement for CoT reasoning was minimal. This disparity underscores the need for enriched datasets and robust training methodologies to foster better reasoning capabilities in VLMs.
Implications for the Future of Vision-Language Models
The findings of this research highlight the critical importance of incorporating detailed rationales into the training of vision-language models. By leveraging both enriched datasets and advanced reinforcement learning techniques, researchers are paving the way for more robust and interpretable multimodal models. This not only enhances the performance of VLMs but also contributes to the broader goal of making AI systems more transparent and trustworthy.
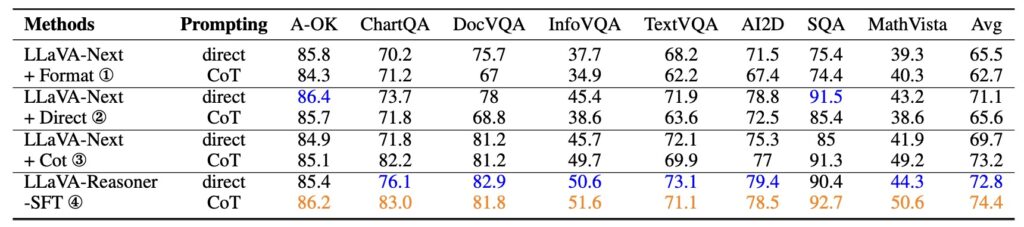
As the field of AI continues to evolve, the emphasis on improving interpretability and reasoning in models will be crucial for their successful application in real-world scenarios. By addressing the current limitations in training practices, researchers are making significant strides toward developing VLMs that can reason effectively across a variety of tasks, ultimately benefiting both the technology and its users.
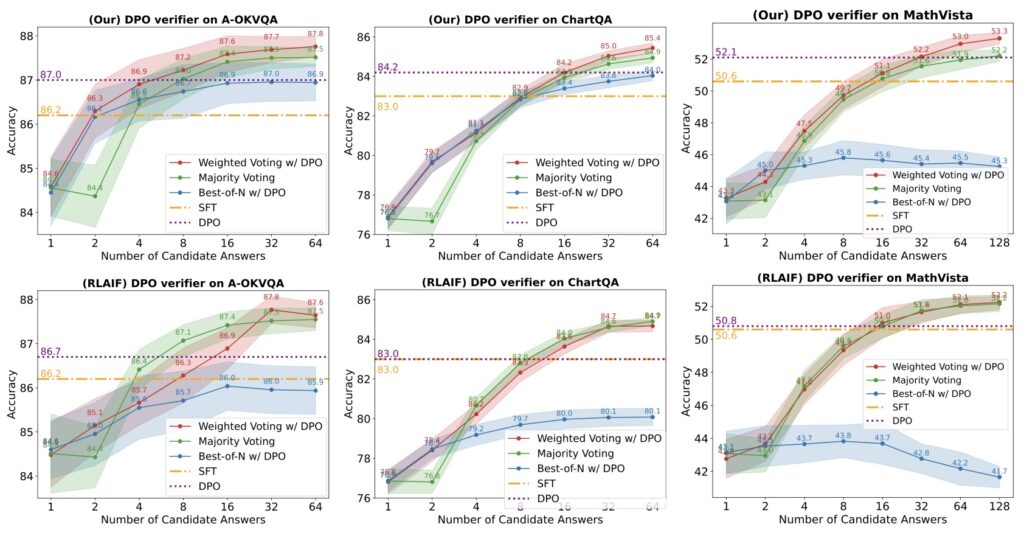
Enhancing chain-of-thought reasoning in vision-language models is not just a technical challenge; it is a vital step toward fostering trust and interpretability in AI systems. The proposed methods of enriching training data and applying reinforcement learning represent promising advancements in the field. As AI continues to integrate more deeply into our lives, improving the reasoning capabilities of VLMs will be essential for ensuring that these technologies serve humanity effectively and ethically. The future of AI hinges on our ability to create models that not only deliver answers but also articulate the reasoning behind those answers, paving the way for a more intelligent and trustworthy technological landscape.