Revolutionizing Audio Creation with Speed and Diversity
- Text-to-audio systems, despite their impressive performance, suffer from slow inference times, rendering them impractical for many creative applications, but a new method promises to change that.
- Adversarial Relativistic-Contrastive (ARC) post-training, a novel adversarial acceleration technique for diffusion and flow models, achieves unprecedented speeds without relying on costly distillation methods.
- By enhancing runtime to milliseconds on consumer-grade GPUs and maintaining quality and diversity, ARC post-training paves the way for broader creative use of text-to-audio models.
The world of generative audio technology has seen remarkable advancements in recent years, with text-to-audio models capable of producing high-quality soundscapes, music, and voiceovers from simple text prompts. However, a significant barrier has persisted: the slow inference times of these systems, often taking seconds to minutes per generation, which severely limits their practicality for real-time or iterative creative workflows. Imagine a musician or sound designer waiting minutes for a single audio clip to generate—such delays stifle spontaneity and innovation. Addressing this critical issue, we introduce a groundbreaking approach called Adversarial Relativistic-Contrastive (ARC) post-training, a method that dramatically accelerates text-to-audio generation without sacrificing quality or diversity.
Traditional text-to-audio models, particularly those based on gaussian flow frameworks like diffusion models or the more recent rectified flows, rely on iterative sampling processes that are computationally expensive at inference time. Over the years, researchers have poured considerable effort into speeding up these models, with most solutions centering on distillation techniques. Distillation involves training a “student” model to mimic a more complex “teacher” model, condensing multiple inference steps into fewer, faster ones. However, distillation comes with significant drawbacks. Online distillation methods require holding multiple full models in memory simultaneously, making them resource-intensive and costly to train. Offline methods, on the other hand, demand substantial resources to generate and store trajectory-output pairs for later training. Moreover, many distillation approaches incorporate Classifier-Free Guidance (CFG), which boosts quality and prompt adherence but often at the expense of diversity and with the risk of over-saturation artifacts in the generated audio.
Enter ARC post-training, the first adversarial acceleration algorithm for diffusion and flow models that sidesteps the pitfalls of distillation and CFG entirely. This innovative method extends a recent relativistic adversarial formulation to the realm of gaussian flow post-training, pairing it with a novel contrastive discriminator objective. The result is a simple yet powerful procedure that not only accelerates inference but also enhances prompt adherence—ensuring the generated audio closely matches the input text description. Unlike distillation, ARC post-training does not require multiple models in memory or extensive offline data generation, making it a more accessible and efficient solution for accelerating text-to-audio systems.
The performance gains achieved with ARC post-training are nothing short of remarkable. When paired with a series of optimizations applied to Stable Audio Open, this method enables the generation of approximately 12 seconds of 44.1kHz stereo audio in just 75 milliseconds on an H100 GPU. Even on a mobile edge-device, it produces around 7 seconds of audio in a comparably short time, marking it as the fastest text-to-audio model known to date. Such speeds transform the usability of these systems, bringing them into the realm of real-time or near-real-time applications. For creators, this means the ability to iterate quickly, experiment freely, and integrate audio generation seamlessly into their workflows—whether they’re designing sound effects for a game, composing music, or crafting podcasts.
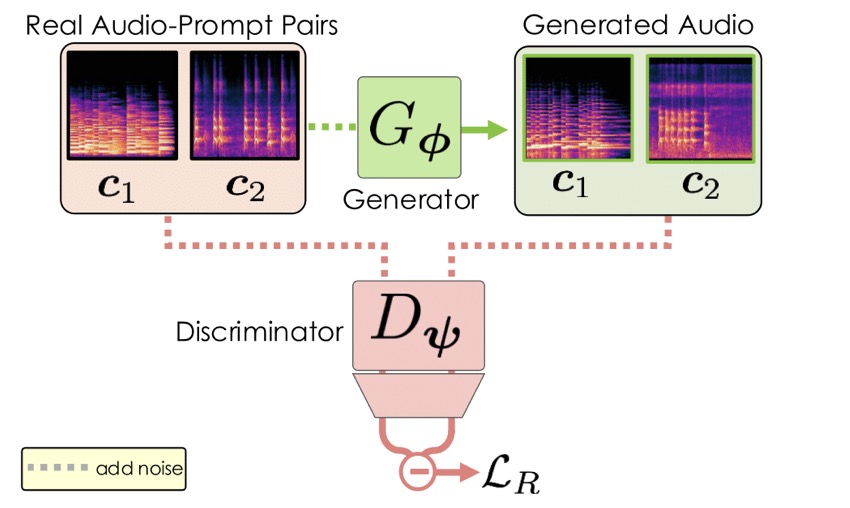
Beyond speed, ARC post-training addresses another critical aspect of generative audio: diversity. Many existing models, particularly those using CFG, tend to produce outputs that lack variety or exhibit repetitive patterns. ARC post-training counters this by incorporating mechanisms that encourage a broader range of outputs, as measured by a newly proposed metric called CCDS (Contrastive Creative Diversity Score). This metric aligns closely with human perceptual assessments of diversity, ensuring that the generated audio feels fresh and varied. The increased diversity, combined with maintained quality, means that users can expect outputs that are not only fast but also creatively inspiring, opening doors to applications previously hindered by the limitations of slower, less varied systems.
Looking at the broader implications, the efficiency and diversity brought by ARC post-training could democratize access to high-quality audio generation tools. Musicians, filmmakers, game developers, and even hobbyists can now envision using text-to-audio models in ways that were once impractical. Imagine a live performance where a DJ generates unique soundscapes on the fly based on audience prompts, or a mobile app that lets users create custom ringtones in seconds. These scenarios are no longer distant dreams but tangible possibilities with the speed and flexibility of ARC-enhanced models. Furthermore, the reduced computational demands of this approach make it feasible to deploy such technology on consumer-grade GPUs or even edge devices, broadening its reach to users without access to high-end hardware.
As we explore the creative potential of these accelerated models, preliminary experiments with audio-to-audio generation hint at even more exciting possibilities. While the primary focus of ARC post-training has been on text-to-audio, adapting it for audio-to-audio tasks—where an input sound is transformed or used as a prompt for new audio—could revolutionize sound design. Future efforts may involve fine-tuning with targeted datasets to achieve more precise control over specific audio characteristics, such as timbre, rhythm, or mood. Such advancements would empower creators to craft highly tailored audio content with minimal effort, further bridging the gap between imagination and realization.
ARC post-training represents a significant leap forward in the field of text-to-audio generation. By achieving unprecedented inference speeds, maintaining high quality, and enhancing output diversity, it addresses the core limitations that have long hindered the practical application of these models. This method not only outperforms traditional distillation-based approaches but also does so with a simplicity and efficiency that make it accessible to a wider audience. As we continue to refine and expand upon this technology, the hope is that text-to-audio systems will soon support an even broader range of creative applications, empowering artists and innovators to bring their sonic visions to life with unparalleled ease and speed.