Combining SAM with Optical Flow to Redefine Motion Analysis
- Innovative Model Integration: FlowSAM integrates the Segment Anything Model (SAM) with optical flow technology to enhance the detection and segmentation of moving objects in videos.
- Superior Benchmark Performance: The approach surpasses existing methods significantly in both single and multi-object motion segmentation benchmarks, thanks to its innovative use of flow data.
- Extended Applications and Efficiency: FlowSAM not only provides state-of-the-art frame-level segmentation but also extends these capabilities to sequence-level segmentation, maintaining object identity throughout video sequences.
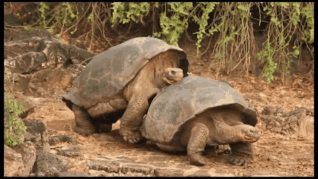
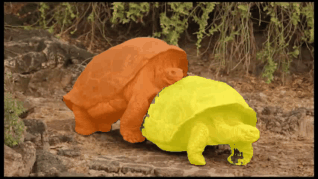
The recent development of FlowSAM marks a significant advancement in the field of video motion segmentation. This new method, developed through the integration of the Segment Anything Model (SAM) with optical flow techniques, has set new standards in the detection and segmentation of moving objects across various video benchmarks.
Technical Breakthroughs and Methodology
FlowSAM presents two distinct models that harness the capabilities of SAM for video segmentation. The first model, FlowI-SAM, directly inputs optical flow into SAM, allowing for precise motion detection without the need for traditional RGB data. The second model, FlowP-SAM, uses RGB inputs alongside flow-generated prompts to guide the segmentation process. These methods significantly simplify the segmentation workflow while enhancing the accuracy and effectiveness of the model.
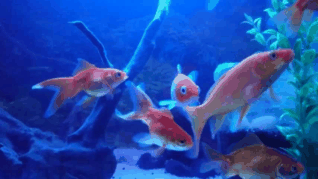
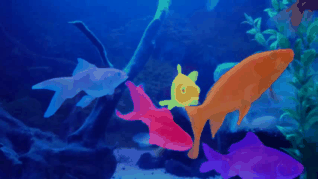
Performance and Benchmarking
FlowSAM’s innovative approach has led to unparalleled performance in motion segmentation tasks. In both single and multi-object benchmarks, such as DAVIS, YTVOS, and MoCA, FlowSAM has outperformed all previous approaches. This high level of accuracy is maintained not only in frame-level segmentation but also in sequence-level segmentation, where the model effectively maintains consistent object identities across frames.
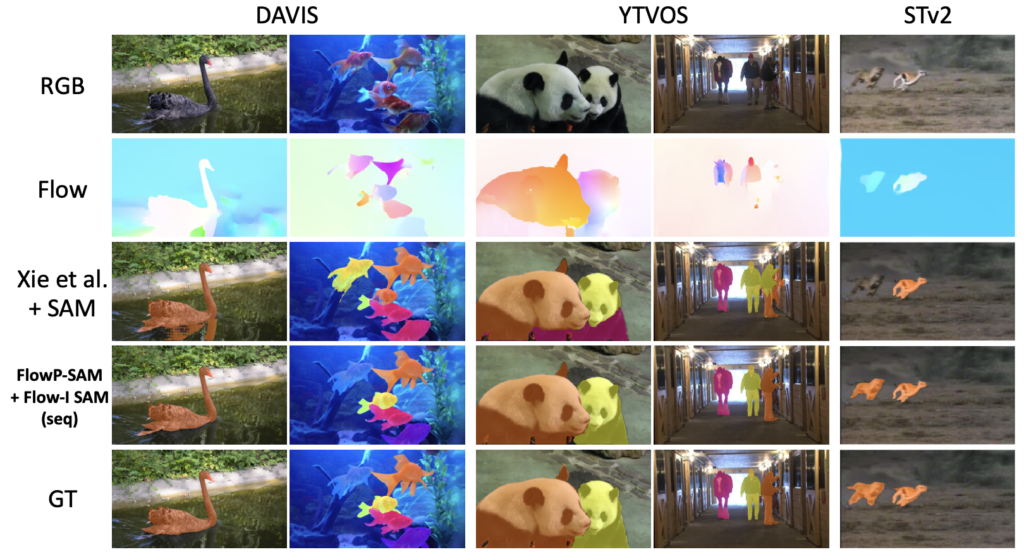
Challenges and Future Directions
Despite its successes, FlowSAM faces challenges, notably the extended processing times due to the computationally intensive image encoder used in the vanilla SAM. However, ongoing developments and optimizations in SAM technology promise to mitigate these issues shortly, potentially reducing inference times significantly.
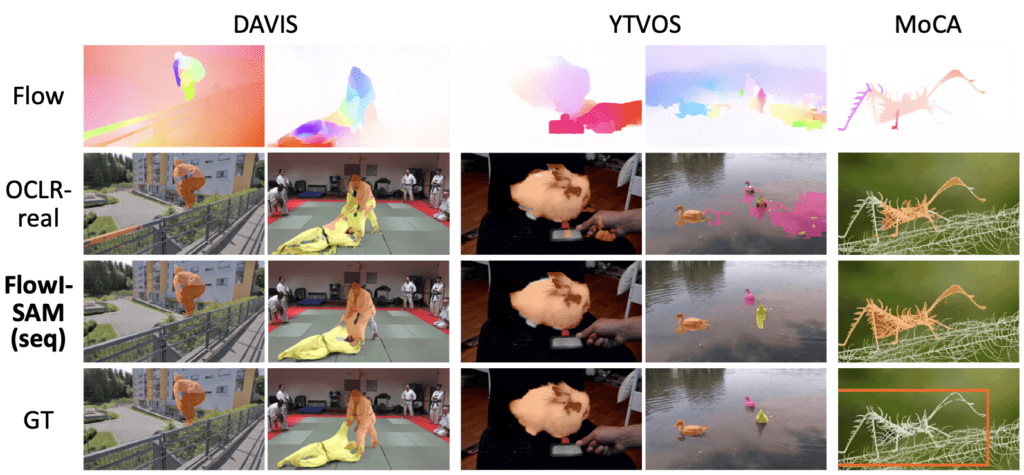
Additionally, the research team has provided comprehensive appendices in their paper, offering deeper insights into the architectural designs, experimental settings, and detailed ablation studies that support the robustness of their findings. They also discuss potential improvements and iterations that could enhance FlowSAM’s efficiency and applicability.
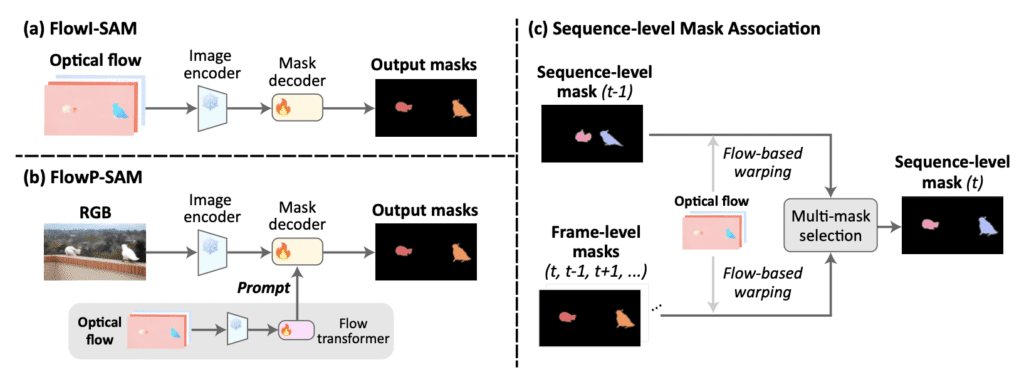
FlowSAM represents a transformative approach to video analysis, offering a more robust and efficient method for segmenting motion in real-world videos. As this technology evolves, it is poised to become a fundamental tool in areas ranging from autonomous driving and surveillance to interactive media and sports analytics, where understanding dynamic environments is crucial.