A Breakthrough in AI-Powered Scene Recovery Combines Spatial Intelligence and Physics for Unprecedented Realism
- Scene Understanding Redefined: CAST combines 2D segmentation, depth analysis, and GPT-powered spatial reasoning to decode complex object relationships.
- Occlusion-Resistant Generation: Advanced 3D models reconstruct hidden geometry using MAE and point cloud conditioning, ensuring pixel-perfect alignment.
- Physics-Driven Perfection: A constraint graph optimizes poses using Signed Distance Fields (SDFs), eliminating floating objects and unnatural collisions.
Humans perceive the world not as isolated objects but as interconnected networks shaped by physics, function, and design. A chair leans against a table; a lamp illuminates a book; shadows define spatial boundaries. Replicating this intricate dance of objects and relationships in 3D requires more than just geometry—it demands an understanding of context, physics, and intent. Traditional single-image 3D reconstruction methods often falter here, producing disjointed scenes with misaligned objects or unrealistic interactions. Enter CAST (Component-Aligned 3D Scene Reconstruction), a novel AI framework that bridges this gap by merging spatial intelligence with physics-aware optimization.
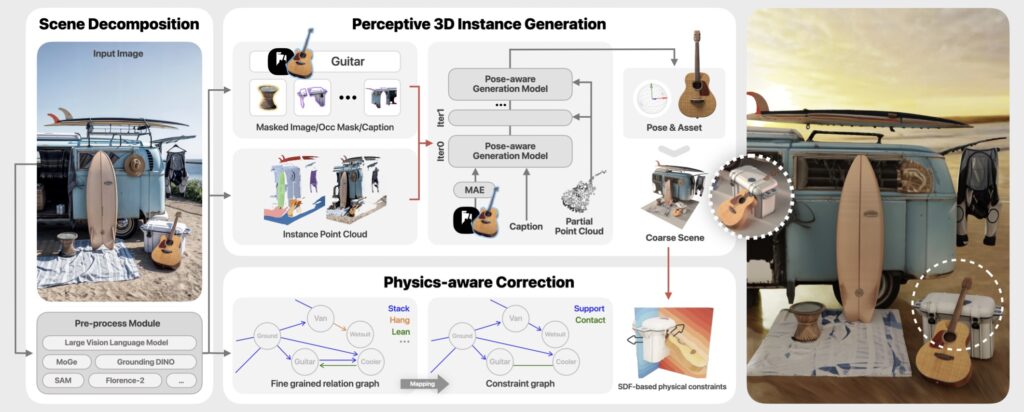
Breaking Down the Scene: Perception Meets Context
CAST begins by dissecting an input RGB image into its core components. Using state-of-the-art 2D segmentation, it identifies objects and estimates their relative depths. But depth alone isn’t enough—where objects are matters less than how they relate. Here, CAST’s GPT-based spatial analyzer shines. By interpreting inter-object relationships (e.g., “a cup rests on a table, not under it”), the system builds a relational map of the scene. This step mirrors human intuition, ensuring that reconstructed scenes obey real-world logic.
In a café scene, CAST recognizes that chairs surround tables, mugs sit near laptops, and lighting casts softer shadows on fabric than metal.
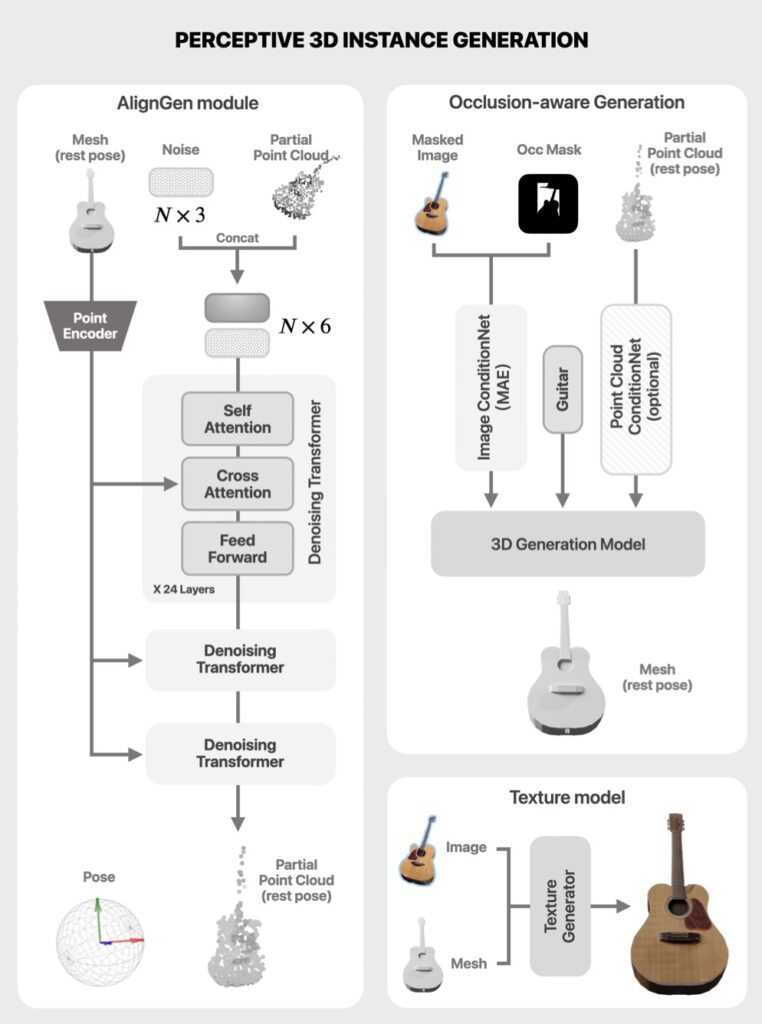
Reconstructing the Unseen: Defying Occlusions
A key hurdle in 3D generation is occluded objects. How do you reconstruct a sofa partially hidden by a table? CAST tackles this with an occlusion-aware 3D generator. Using Masked Autoencoders (MAE) and point cloud conditioning, the model infers hidden geometry by cross-referencing visible textures and learned object priors. This approach ensures that even partially visible objects, like a lamp obscured by a curtain, are accurately rebuilt in full 3D.
By aligning generated meshes with the source image’s geometry and texture, CAST minimizes “floaters” or misaligned edges that plague other methods.
Physics Takes the Wheel: Ensuring Real-World Coherence
Reconstructed objects are nothing without physical plausibility. CAST’s final step applies a physics-aware correction layer. A fine-grained relation graph—informed by material properties, object roles, and spatial norms—generates a constraint graph. This graph guides optimization via Signed Distance Fields (SDFs), which mathematically enforce rules like “objects cannot intersect” or “plates must lie flat.” The result? No floating books, no chairs clipping through walls, and shadows that obey light sources.
Scenes aren’t just visually accurate—they’re physically interactable, critical for robotics and VR.
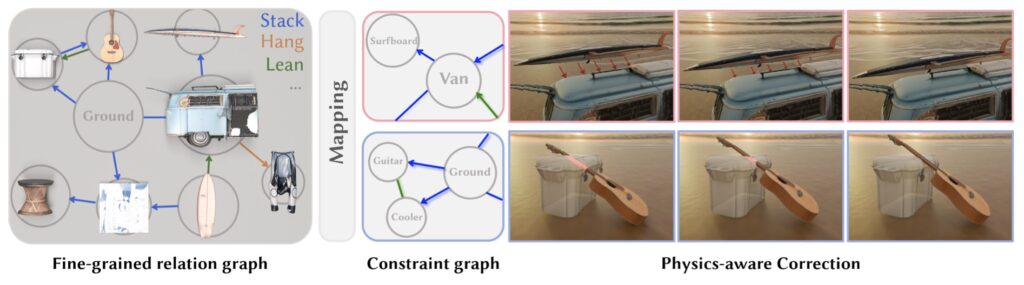
Beyond Pixels to Practical Innovation
CAST’s implications span industries:
- Entertainment: Filmmakers can rapidly convert real-world sets into detailed 3D environments, reducing post-production costs.
- Gaming: Designers build immersive worlds where every object adheres to physical laws, enhancing player realism.
- Robotics: CAST-generated scenes train robots in simulation environments that mirror real kitchens, warehouses, or hospitals.
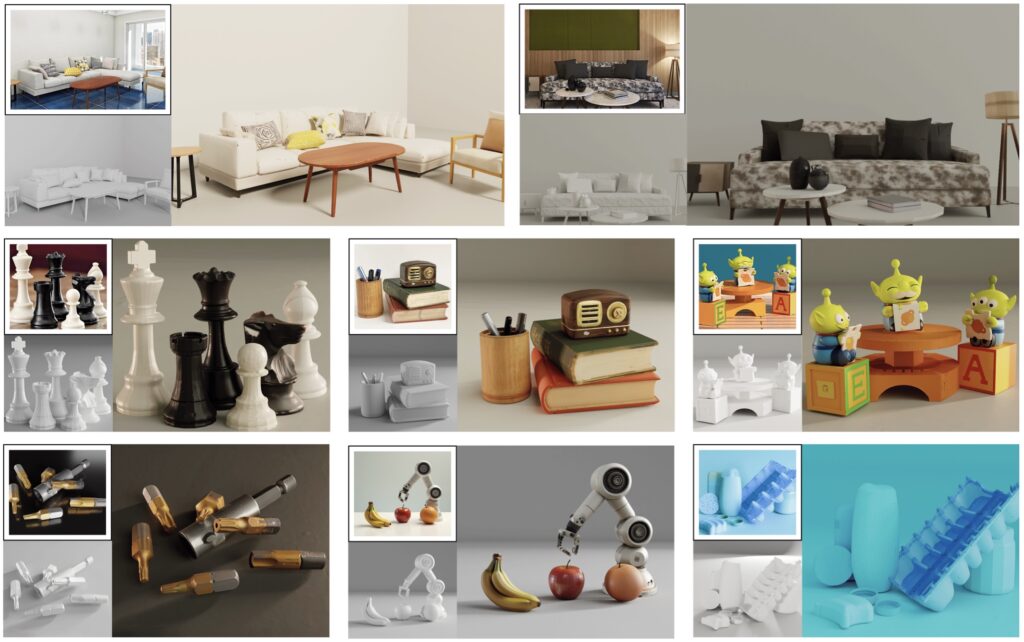
While CAST marks a leap forward, challenges remain:
- Object Detail: Current 3D generators lack precision for intricate textures (fabric patterns), occasionally causing visual mismatches.
- Lighting Gaps: CAST doesn’t yet model dynamic lighting, leaving shadows static and reflections oversimplified.
- Complex Scenes: Densely packed environments (cluttered workshops) may strain the spatial analyzer.
Future iterations could integrate neural radiance fields (NeRFs) for lighting and generative AI for ultra-detailed objects. Scaling to video inputs—reconstructing 3D scenes frame-by-frame—is another frontier.
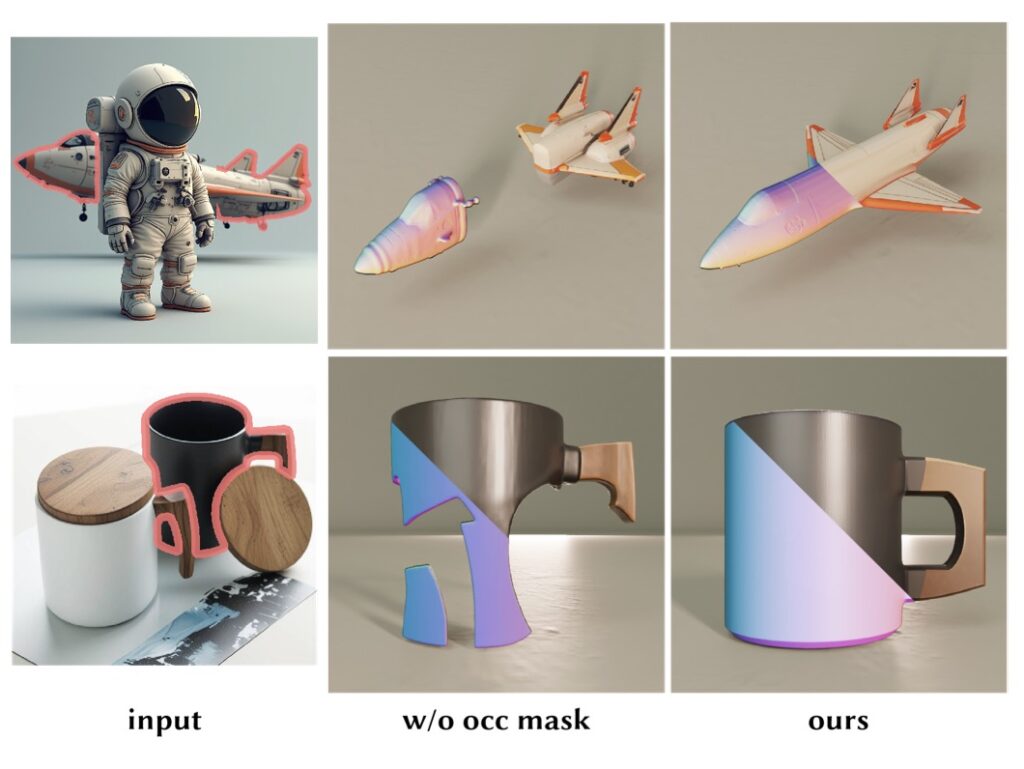
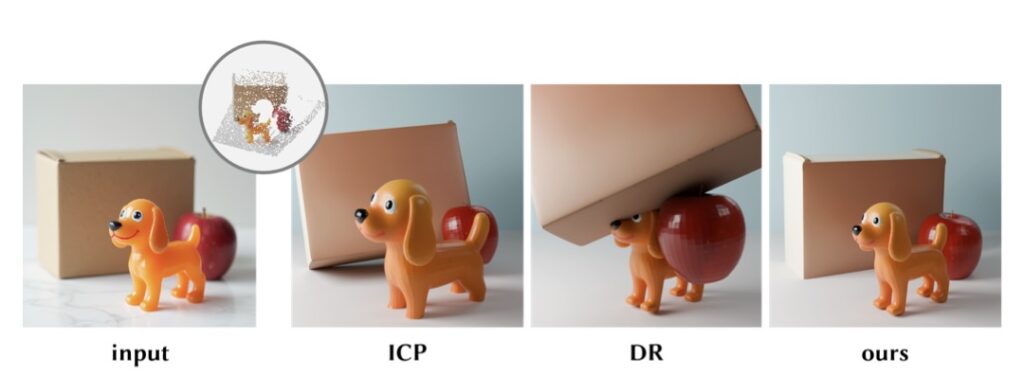
A New Era for Virtual Worlds
CAST redefines single-image 3D reconstruction by treating scenes as ecosystems of interdependent objects. Its blend of spatial reasoning, occlusion resilience, and physics-driven refinement sets a new standard for realism. As AI continues to blur the line between digital and physical, frameworks like CAST will power the next generation of virtual experiences—where every pixel tells a story, and every object obeys the rules of reality.
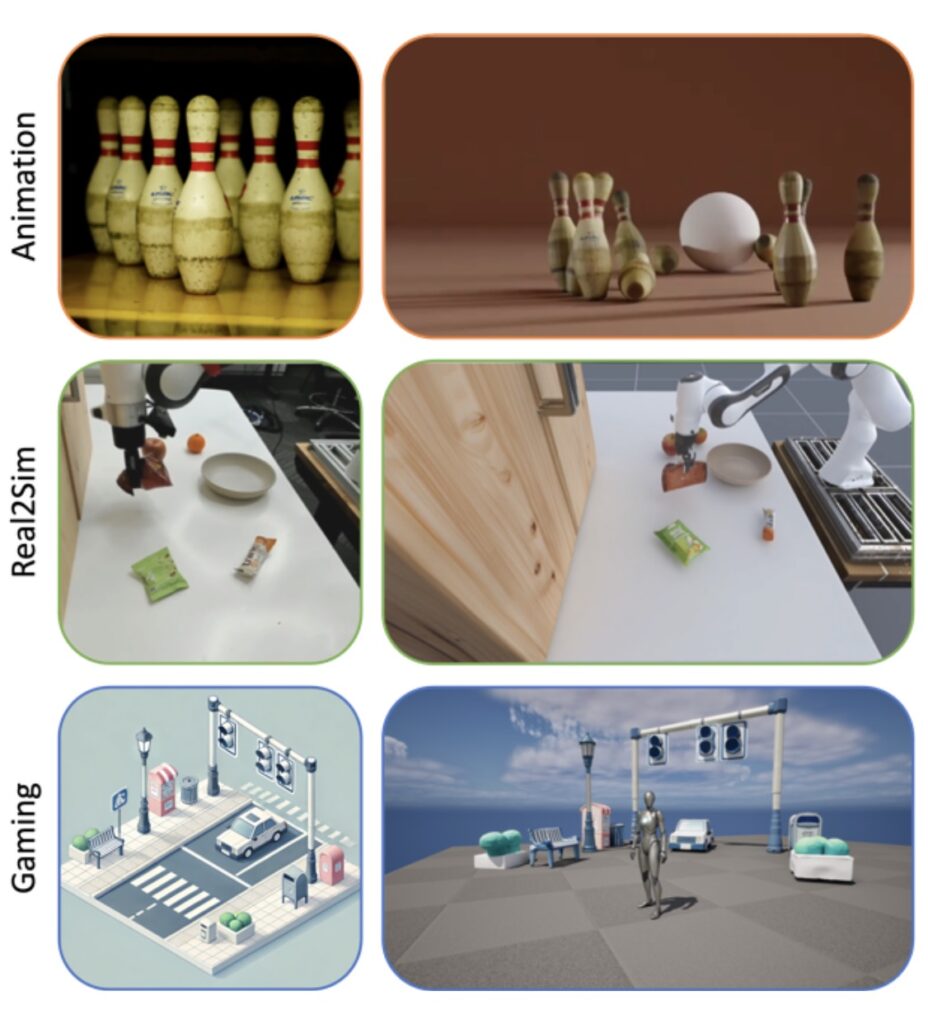