New Approach Converts 3D Models into 2D Images for Simplified Generation
- New method encapsulates 3D geometry and appearance into a 64×64 pixel image, simplifying the generation process.
- Uses image generation models like Diffusion Transformers for creating high-quality 3D objects.
- Achieves point cloud FID comparable to recent models while supporting PBR material generation.
A groundbreaking approach to generating realistic 3D models has been introduced, transforming the complexity of 3D shapes into a more manageable 2D format through a method called “Object Images.” This innovative technique encapsulates surface geometry, appearance, and patch structures within a 64×64 pixel image, making it easier to handle the inherent geometric and semantic irregularities of 3D shapes.
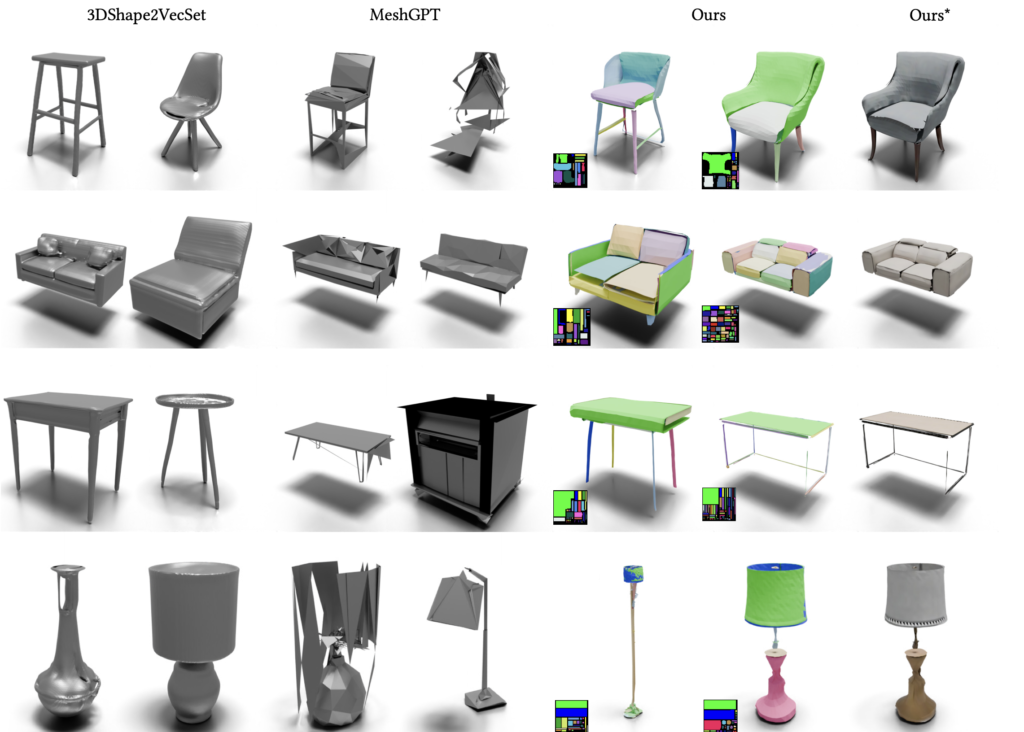
Simplifying 3D Shape Generation
Traditional methods of creating 3D models involve intricate processes that can be both time-consuming and technically challenging. These methods often struggle with the irregular nature of polygonal meshes and the complex topologies of 3D objects, which include holes and multiple connected components. Moreover, 3D assets come with rich semantic sub-structures essential for editing, interaction, and animation, further complicating the modeling process.
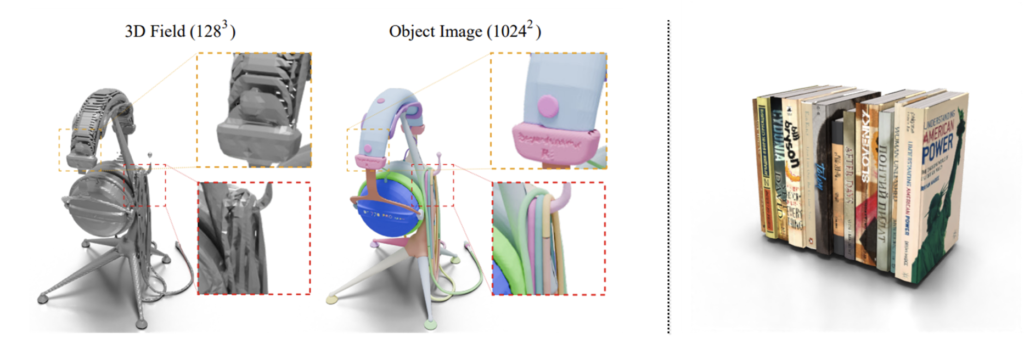
The newly introduced method addresses these issues by converting complex 3D shapes into a simplified 2D format. This conversion allows the use of image generation models, such as Diffusion Transformers, to directly generate 3D shapes. Evaluated on the ABO dataset, the generated shapes with patch structures show point cloud Fréchet Inception Distance (FID) scores comparable to the latest 3D generative models. Additionally, this approach naturally supports Physically Based Rendering (PBR) material generation, enhancing the realism of the 3D objects.
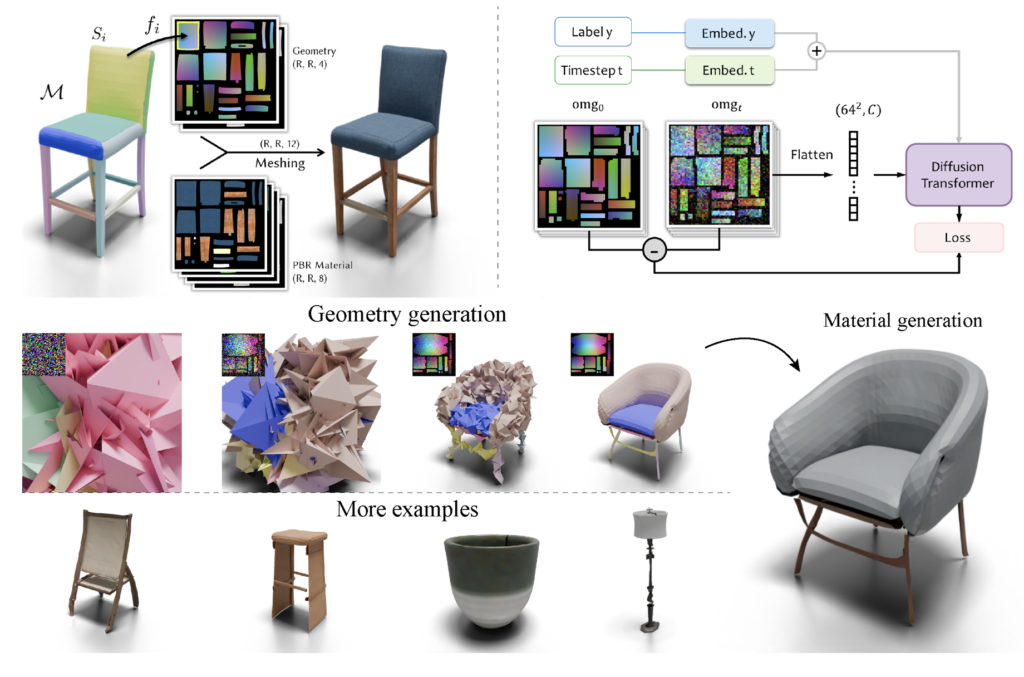
Overcoming Challenges in 3D Model Generation
Previous attempts to tackle the irregularities of 3D modeling either focused on geometric or semantic irregularity but rarely both. Many methods converted 3D shapes into more regular representations like point clouds or multi-view images, which, while easier for neural networks to process, often resulted in a loss of essential geometric and semantic information.
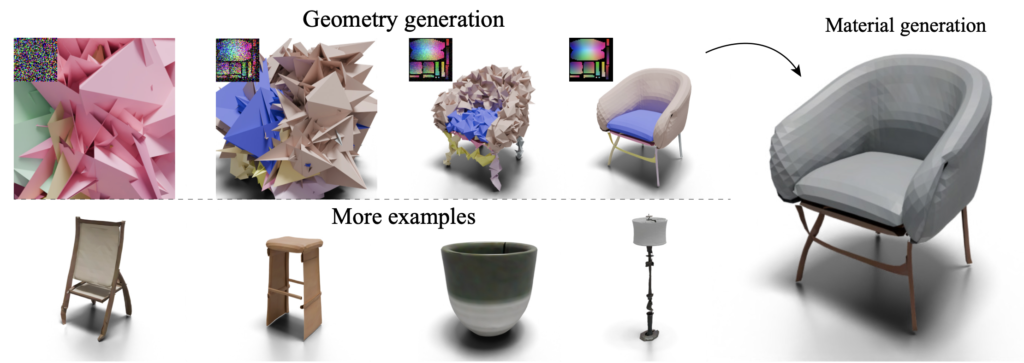
The new paradigm of using a 64×64 2D image to represent 3D objects overcomes these limitations. By denoising a small 2D image with an image diffusion model, researchers can generate high-quality 3D objects with detailed textures and accurate patch structures. This method shows promise for generating photo-realistic 3D models that maintain their structural integrity and are suitable for applications like animation and interaction.
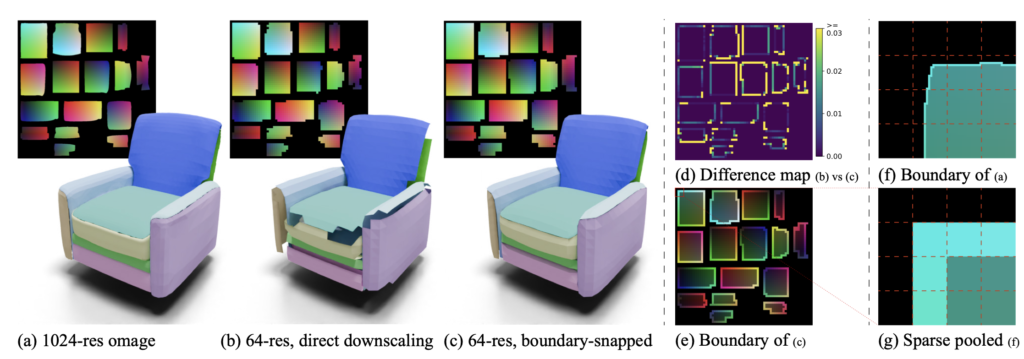
Future Directions and Limitations
While this method marks a significant advancement in 3D model generation, it is not without limitations. Currently, it cannot guarantee the creation of watertight meshes and requires high-quality UV atlases for training 3D shapes. Additionally, the resolution is limited to 64×64 pixels, which may not be sufficient for all applications.
Future research will focus on addressing these limitations to fully leverage the benefits of this regular representation. Enhancing the resolution and ensuring the generation of watertight meshes are critical next steps. By continuing to refine this approach, the potential for high-quality, structured 3D asset generation can be fully realized, offering new tools and efficiencies for industries such as film, interactive entertainment, manufacturing, and robotics.
The introduction of the Object Images method for generating 3D models represents a significant leap forward in 3D modeling technology. By simplifying the process and addressing key challenges in geometric and semantic irregularity, this approach has the potential to streamline the creation of high-quality 3D assets. As the technology continues to evolve, it promises to open up new possibilities for various applications, revolutionizing the way we create and interact with 3D content.