Unleashing the Power of Diffusion Priors for Consistent Geometry Estimation
- GeometryCrafter introduces a novel framework that recovers high-fidelity point map sequences with temporal coherence from open-world videos, enabling accurate 3D/4D reconstruction and depth-based applications.
- The core of GeometryCrafter lies in a point map Variational Autoencoder (VAE) that learns a latent space agnostic to video latent distributions, facilitating effective encoding and decoding of point maps.
- Extensive evaluations demonstrate that GeometryCrafter achieves state-of-the-art 3D accuracy, temporal consistency, and generalization capability, outperforming prior methods in performance and generalization.
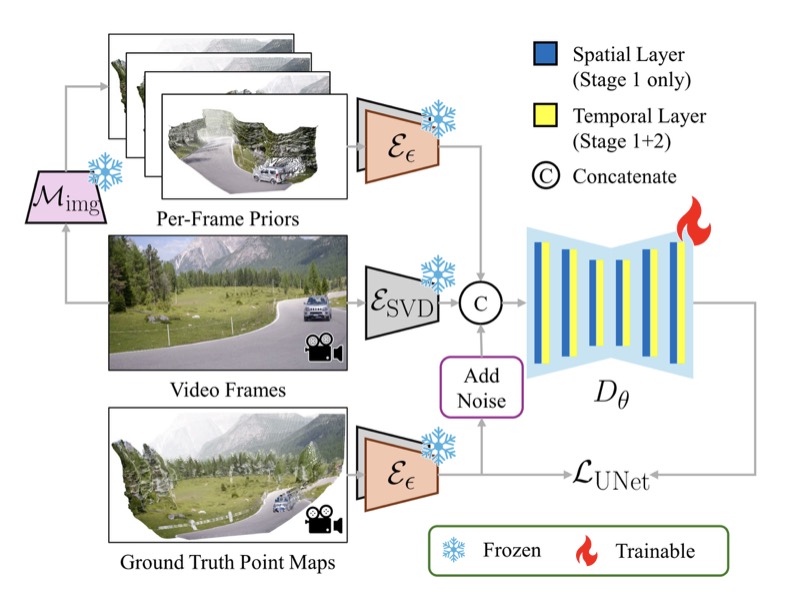
In the realm of video depth estimation, remarkable advancements have been made, yet existing methods still grapple with inherent limitations in achieving geometric fidelity through affine-invariant predictions. These limitations hinder their applicability in reconstruction and other metrically grounded downstream tasks. However, a groundbreaking solution has emerged in the form of GeometryCrafter, a novel framework that promises to revolutionize the field of 3D reconstruction from open-world videos.
At the heart of GeometryCrafter lies a point map Variational Autoencoder (VAE) that learns a latent space agnostic to video latent distributions. This innovative approach enables effective encoding and decoding of point maps, overcoming the challenges posed by unbounded point map values. By leveraging the VAE, GeometryCrafter trains a video diffusion model to model the distribution of point map sequences conditioned on the input videos, ensuring temporal coherence and high-fidelity reconstruction.
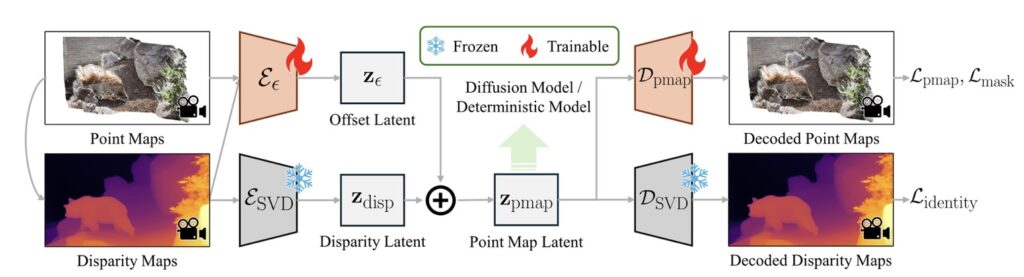
One of the key strengths of GeometryCrafter is its ability to facilitate a wide range of downstream applications. From accurate 3D/4D reconstruction to camera parameter estimation and depth-based video editing or generation, GeometryCrafter opens up new possibilities for researchers and practitioners alike. Its decoupled point map representation eliminates the location-dependent characteristics of point maps, enhancing robustness to resolutions and aspect ratios, further expanding its applicability.
To achieve these remarkable results, GeometryCrafter employs a dual-encoder design within the point map VAE. The native encoder, inherited from SVD, captures normalized disparity maps, while a residual encoder embeds remaining information as an offset. This approach preserves the original latent space by regulating the latents via the original decoder, enabling the utilization of pretrained diffusion priors. The point map decoder then recovers the final point maps from the latent codes, ensuring accurate and high-quality reconstruction.
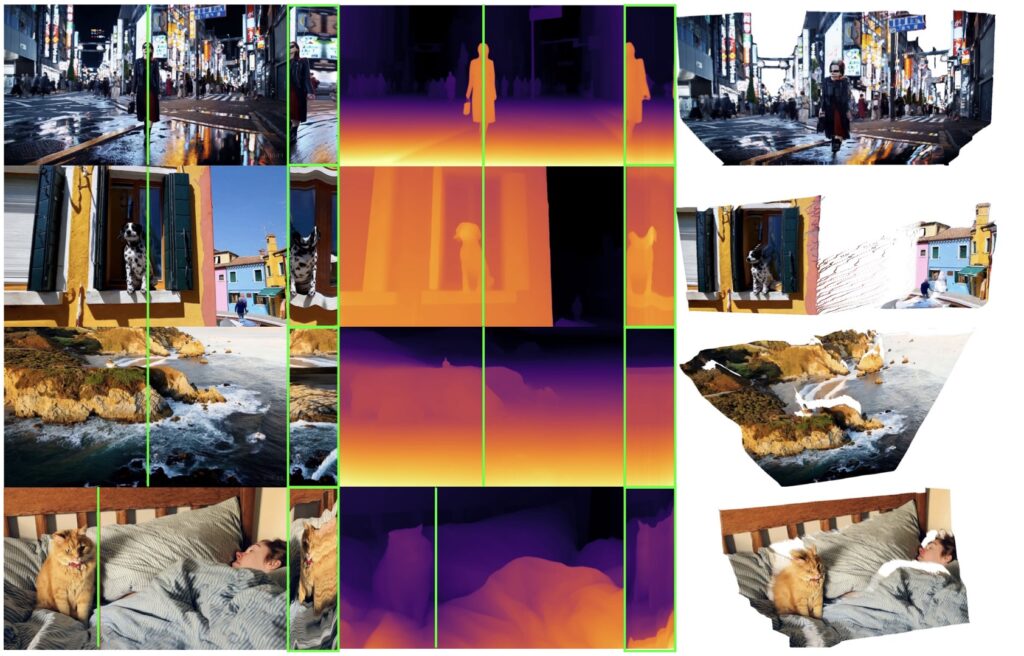
Extensive evaluations on diverse datasets have demonstrated that GeometryCrafter outperforms prior methods in terms of 3D accuracy, temporal consistency, and generalization capability. Its ability to handle open-world videos, where the content and camera motion are unconstrained, sets it apart from existing approaches. However, it is worth noting that GeometryCrafter’s main limitation lies in its relatively high computational and memory overhead due to the large model size.
GeometryCrafter holds immense potential for transforming the way we approach 3D reconstruction and depth-based applications. Its ability to recover high-fidelity point map sequences with temporal coherence from open-world videos opens up new avenues for research and innovation. Whether it’s in the realm of virtual reality, autonomous navigation, or content creation, GeometryCrafter is poised to make a significant impact on the field of computer vision and beyond.
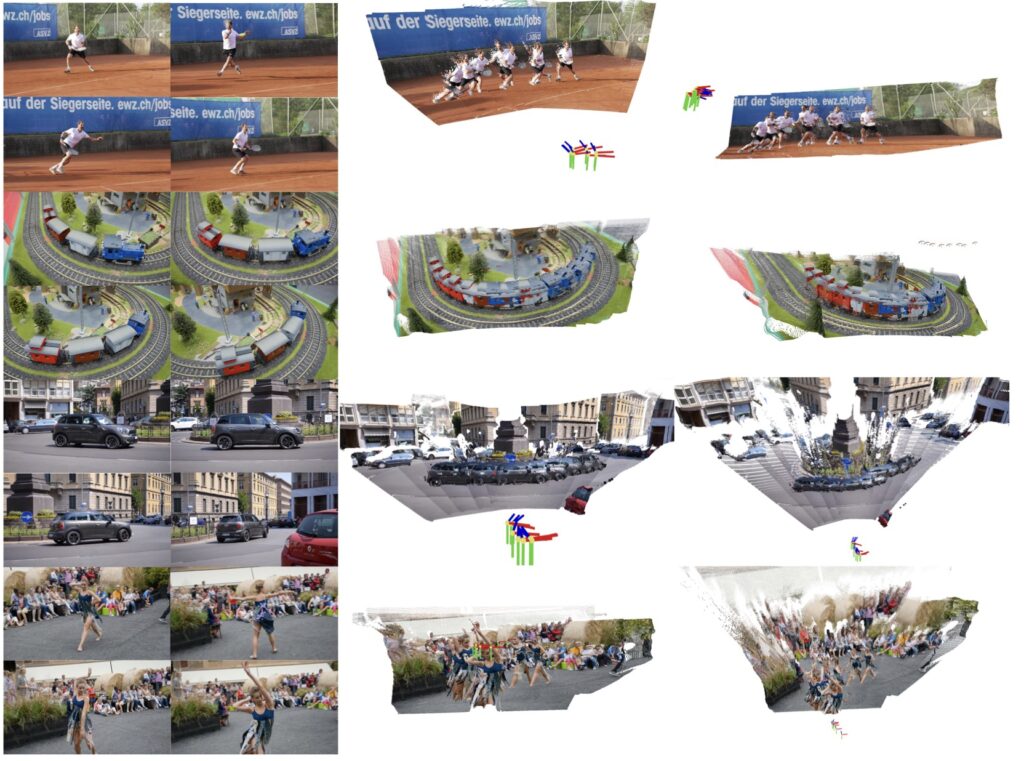