DeepMind’s JEST Method Optimizes Data for Remarkable Performance Gains
- Faster and More Efficient Training: DeepMind’s JEST method achieves 13 times the training speed and 10 times higher power efficiency.
- Innovative Data Selection: JEST focuses on selecting high-quality data batches, enhancing training efficiency.
- Environmental Impact: This advancement could significantly reduce the power demands of AI data centers.
Google DeepMind, the AI research arm of Google, has unveiled groundbreaking research that promises to revolutionize AI model training. The new JEST (joint example selection) method claims to improve training speed and energy efficiency by an order of magnitude, delivering 13 times faster performance and ten times greater power efficiency than current techniques. This development is particularly timely as discussions around the environmental impact of AI data centers gain momentum.
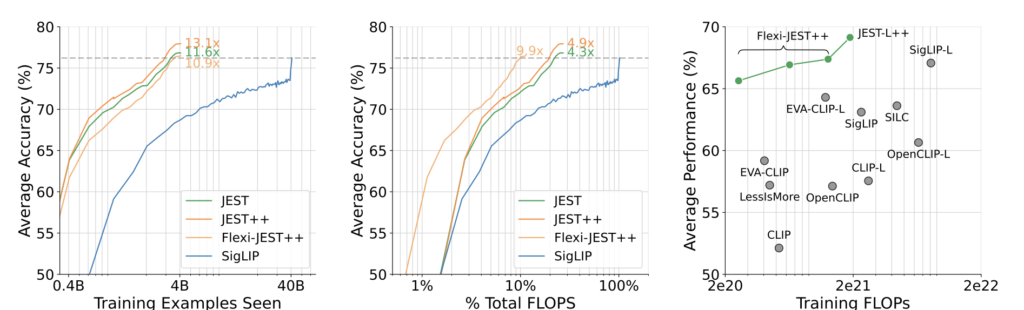
Faster and More Efficient Training
DeepMind’s JEST method introduces a significant departure from traditional AI training techniques, which typically focus on individual data points. Instead, JEST optimizes the training process by evaluating entire data batches. The approach begins with a smaller AI model that grades data quality from high-quality sources. These graded batches are then compared to a larger, lower-quality dataset. The small JEST model identifies the most suitable batches for training, and the larger model is subsequently trained based on these findings.
The results are impressive. DeepMind’s research shows that JEST surpasses state-of-the-art models, requiring up to 13 times fewer iterations and 10 times less computation. This efficiency gain could have profound implications for the future of AI, particularly in reducing the computational and energy costs associated with training large models.
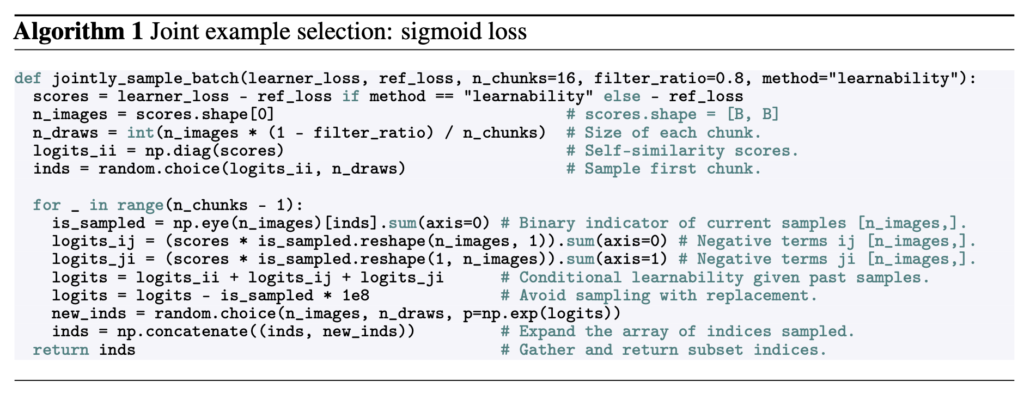
Innovative Data Selection
A key element of the JEST method is its ability to steer the data selection process towards smaller, well-curated datasets. This technique, which leverages multimodal contrastive learning, exposes the dependencies between data points and measures the joint learnability of a batch. By selecting data in larger, high-quality batches rather than individual examples, JEST significantly accelerates the training process.
However, the success of this method hinges on the quality of the initial training data. The adage “garbage in, garbage out” is particularly relevant here, as the effectiveness of JEST relies on human-curated datasets of the highest possible quality. This requirement may pose challenges for hobbyists or amateur AI developers who lack the resources to curate such data, making JEST more suitable for large-scale, professional research environments.
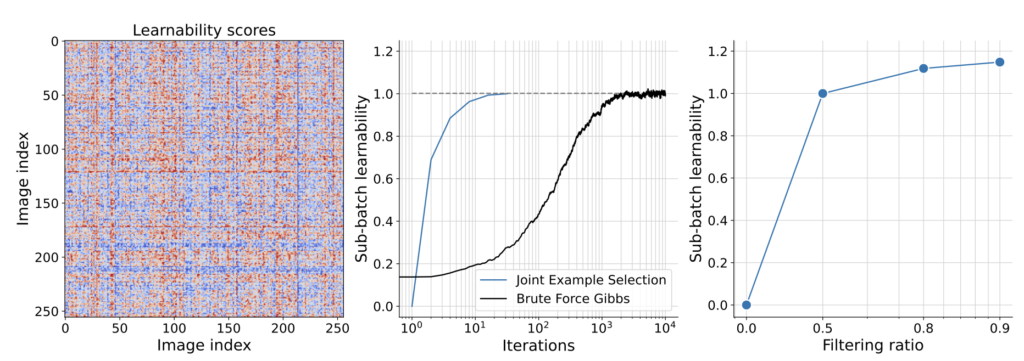
Environmental Impact
The introduction of JEST comes at a critical time. AI workloads consumed about 4.3 GW in 2023, nearly matching the annual power consumption of Cyprus. With AI’s energy demands projected to grow, efficient training methods like JEST could play a crucial role in mitigating the environmental impact of AI technologies. A single ChatGPT request, for instance, costs ten times more in power than a Google search, highlighting the urgent need for more efficient AI systems.
DeepMind’s research suggests that JEST could help reduce the power draw required for training AI models, potentially easing the strain on power grids and lowering operational costs. However, there is also the possibility that companies might use these efficiency gains to accelerate training outputs further, keeping power consumption high but achieving faster results.
Ideas for Further Exploration
- Broader Industry Adoption: Investigating how JEST methods can be integrated into various AI applications across different industries, including healthcare, finance, and autonomous systems.
- Sustainable AI Practices: Developing guidelines and best practices for implementing energy-efficient AI training methods to minimize environmental impact.
- Educational Outreach: Creating educational resources and training programs to help smaller AI research labs and independent developers understand and utilize JEST techniques.
The JEST method represents a significant advancement in AI training, offering a solution to the escalating computational and energy costs associated with large language models. As the AI industry continues to evolve, innovations like JEST will be essential in balancing performance gains with sustainable practices, ensuring that the growth of AI technologies does not come at an untenable environmental cost.