Unlocking the Future of Deep Learning: A Deep Dive into GRIN MoE
- Microsoft introduces GRIN MoE, an innovative Gradient-Informed Mixture of Experts model designed to enhance efficiency in deep learning.
- The model selectively activates parameters, dramatically reducing computational demands while maintaining high performance across various tasks.
- GRIN MoE outperforms traditional models on multiple benchmarks, paving the way for more scalable AI solutions.
Artificial intelligence has transformed industries by powering advances in natural language processing, computer vision, and data analytics. However, as models grow in size and complexity, they face significant computational challenges. Traditional approaches often struggle to maintain efficiency and performance as they scale, particularly when it comes to managing resource allocation. Recognizing these issues, Microsoft has launched the GRIN MoE (GRadient-INformed Mixture of Experts) model, a groundbreaking approach that promises to reshape the landscape of deep learning.
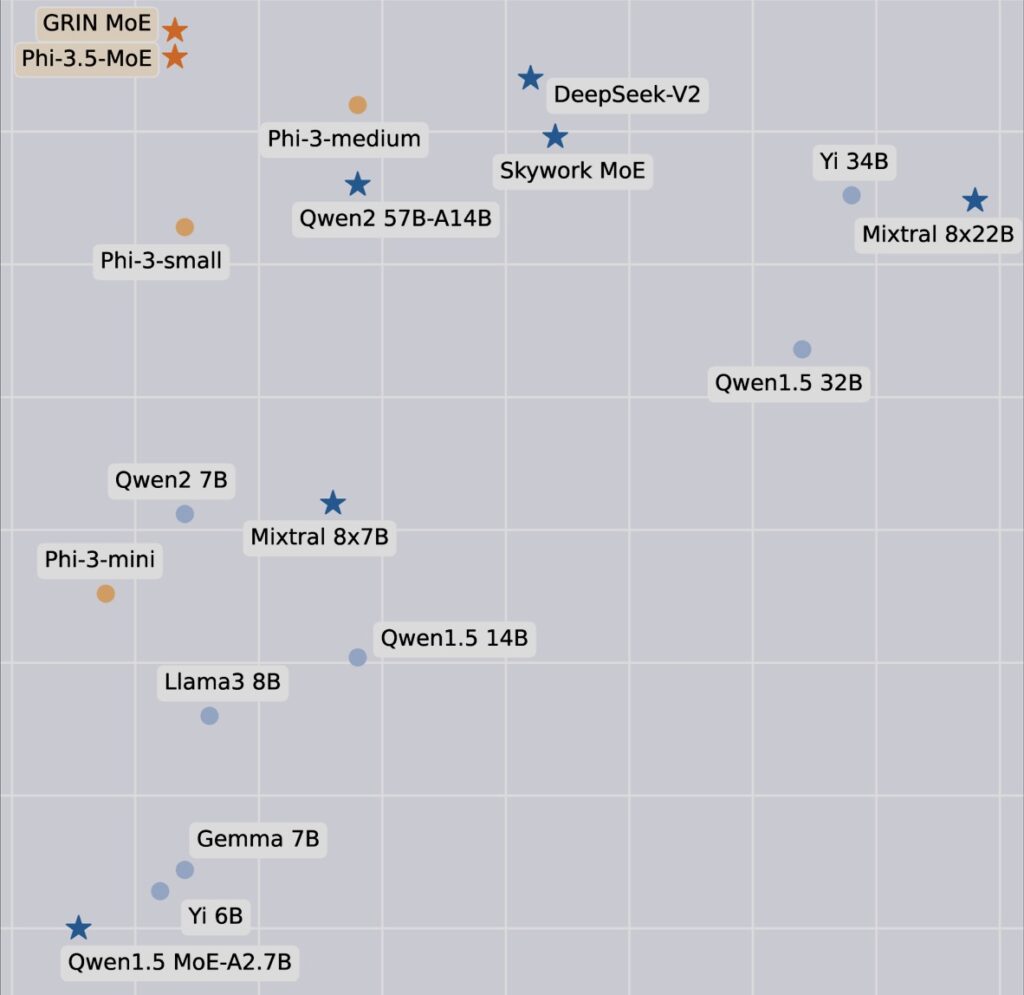
The GRIN MoE model addresses the limitations of both dense and sparse models that have dominated AI research. Dense models, such as GPT-3 and GPT-4, engage all their parameters for each input, leading to high computational costs. Conversely, sparse models aim to activate only a subset of parameters but often rely on complex expert routing mechanisms and token dropping, which can hinder training efficiency. By introducing a novel gradient estimation technique, GRIN MoE enhances model parallelism, enabling more efficient training without sacrificing performance.
At the heart of the GRIN MoE model is its architecture, which comprises multiple MoE layers. Each layer features 16 experts, but only the top two experts are activated per input token, ensuring that computational resources are utilized efficiently. This targeted activation significantly reduces the number of active parameters while maintaining the model’s capability to perform complex tasks. Moreover, the introduction of SparseMixer-v2 allows for better gradient estimation related to expert routing, overcoming the limitations faced by traditional methods that depend on gating gradients.
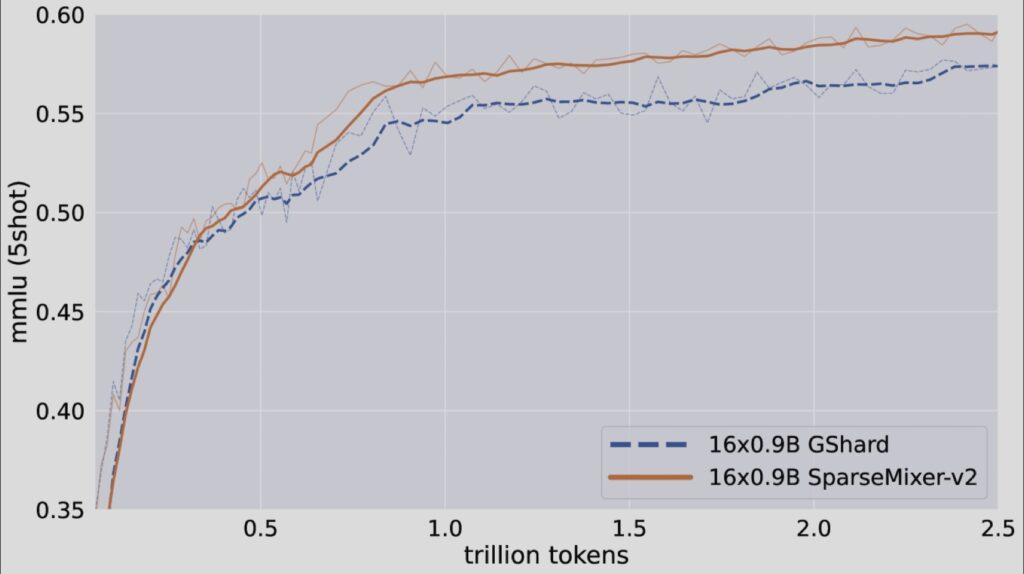
Performance evaluations of GRIN MoE have yielded impressive results across a variety of benchmarks. In the MMLU (Massive Multitask Language Understanding) benchmark, the model achieved a score of 79.4, outperforming several dense models of similar or greater size. It excelled in specialized tasks as well, scoring 83.7 on HellaSwag for common-sense reasoning and 74.4 on HumanEval for coding challenges. Remarkably, GRIN MoE operates with only 6.6 billion activated parameters during inference, surpassing the performance of both a 7-billion parameter dense model and a 14-billion parameter dense model on the same datasets.
In addition to its performance advantages, GRIN MoE significantly improves training efficiency. Tested on 64 H100 GPUs, it achieved an 86.56% throughput, demonstrating that sparse computation can scale effectively while retaining high efficiency. Unlike other models that may compromise accuracy due to token dropping, GRIN MoE maintains robust performance across various tasks, showcasing its potential for real-world applications.
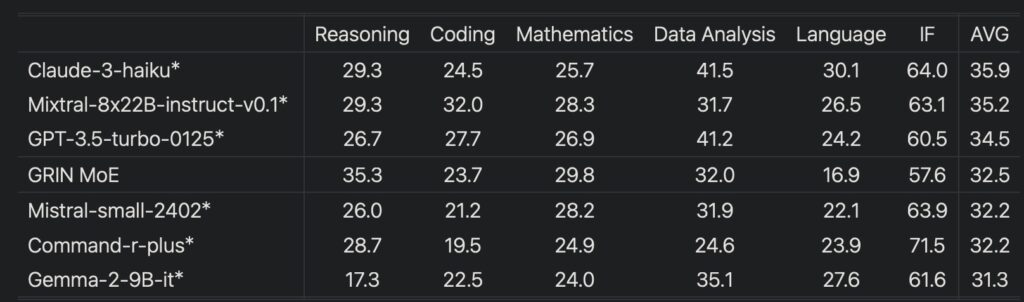
Microsoft’s GRIN MoE model stands as a significant advancement in AI research, addressing the critical challenges of scalability and efficiency in deep learning. By employing a novel approach to gradient estimation and expert routing, GRIN MoE not only enhances performance but also sets a new standard for training efficiency. As researchers and developers continue to explore its capabilities, this model is poised to facilitate groundbreaking applications across numerous fields, solidifying its place in the future of artificial intelligence.