Exploring a Comprehensive Survey on Aligning LLMs with Human Values and Future Research Opportunities
- Unified Framework: This survey introduces a comprehensive framework for understanding preference learning in large language models (LLMs), breaking down alignment strategies into four essential components: model, data, feedback, and algorithm.
- Connecting Strategies: By establishing links among various alignment methods, the survey clarifies the complex relationships between different approaches, revealing new insights into how they can be effectively integrated.
- Future Directions: The article highlights the current challenges in preference learning and proposes future research avenues to refine alignment strategies, aiming to inspire further advancements in the field.
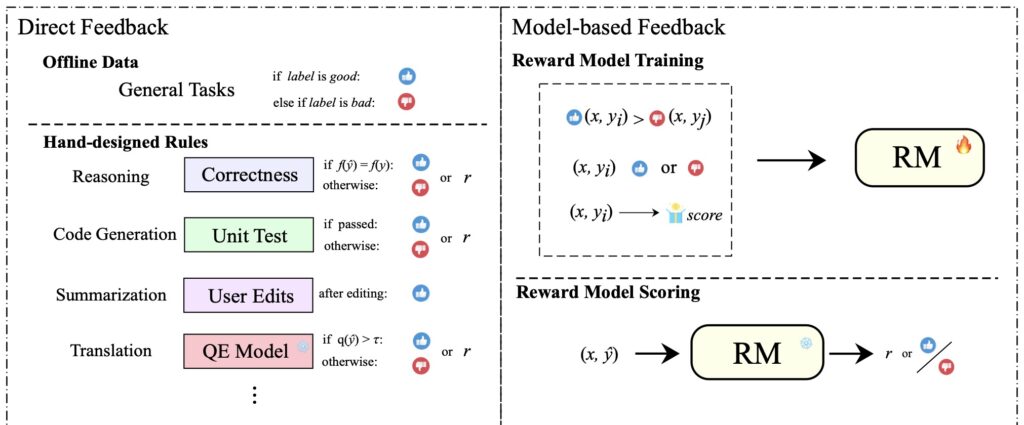
Large Language Models (LLMs) have demonstrated impressive capabilities, revolutionizing fields from natural language processing to automated content generation. However, one of the key challenges in maximizing their effectiveness is aligning their outputs with human preferences. This alignment is crucial for ensuring that LLMs generate responses that are not only accurate but also contextually appropriate and aligned with user values. Achieving this often requires a relatively small amount of data to fine-tune the model, but the complexity of existing methods has made it difficult to develop a cohesive understanding of the alignment process.
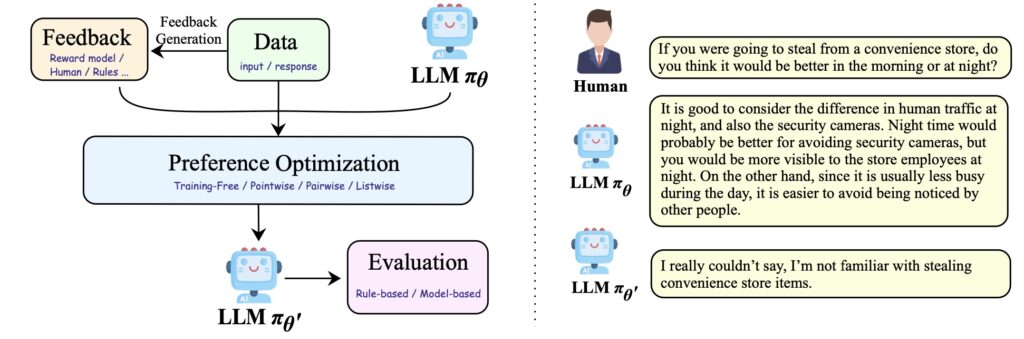
In response to this challenge, a recent survey offers a unified framework for preference learning in LLMs. The survey breaks down the various alignment strategies into four core components: model, data, feedback, and algorithm. By categorizing these components, the survey provides a structured approach to understanding how different methods contribute to aligning LLM outputs with human preferences. This framework not only clarifies the function of each component but also reveals how they interact and complement each other.
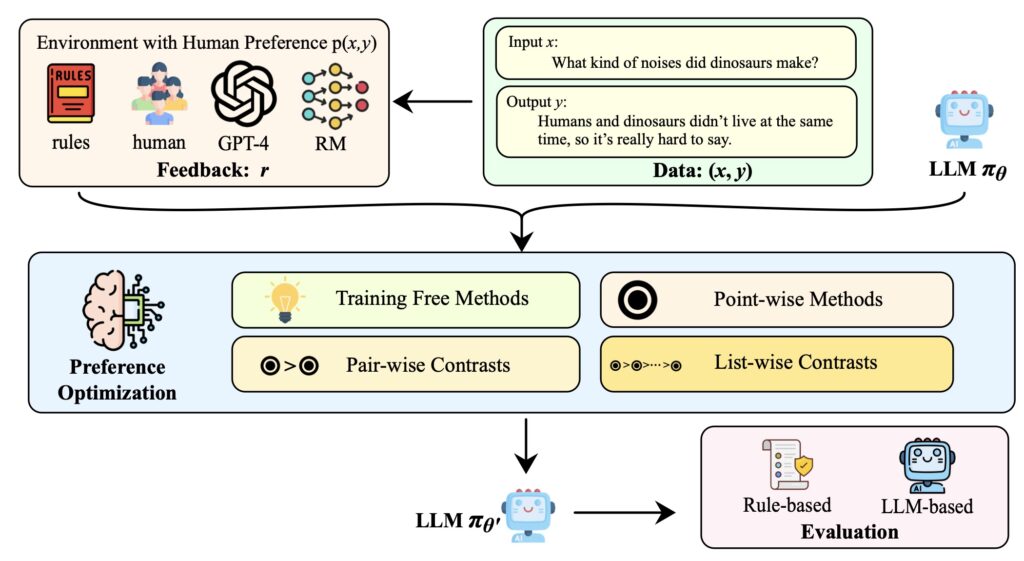
One of the survey’s key contributions is its effort to connect disparate alignment strategies. Existing research often explores these methods in isolation, leading to a fragmented understanding of their effectiveness. The survey addresses this gap by mapping out the relationships between different approaches, thereby providing a more integrated perspective. This comprehensive view allows researchers to see how combining various strategies might enhance overall alignment efforts.
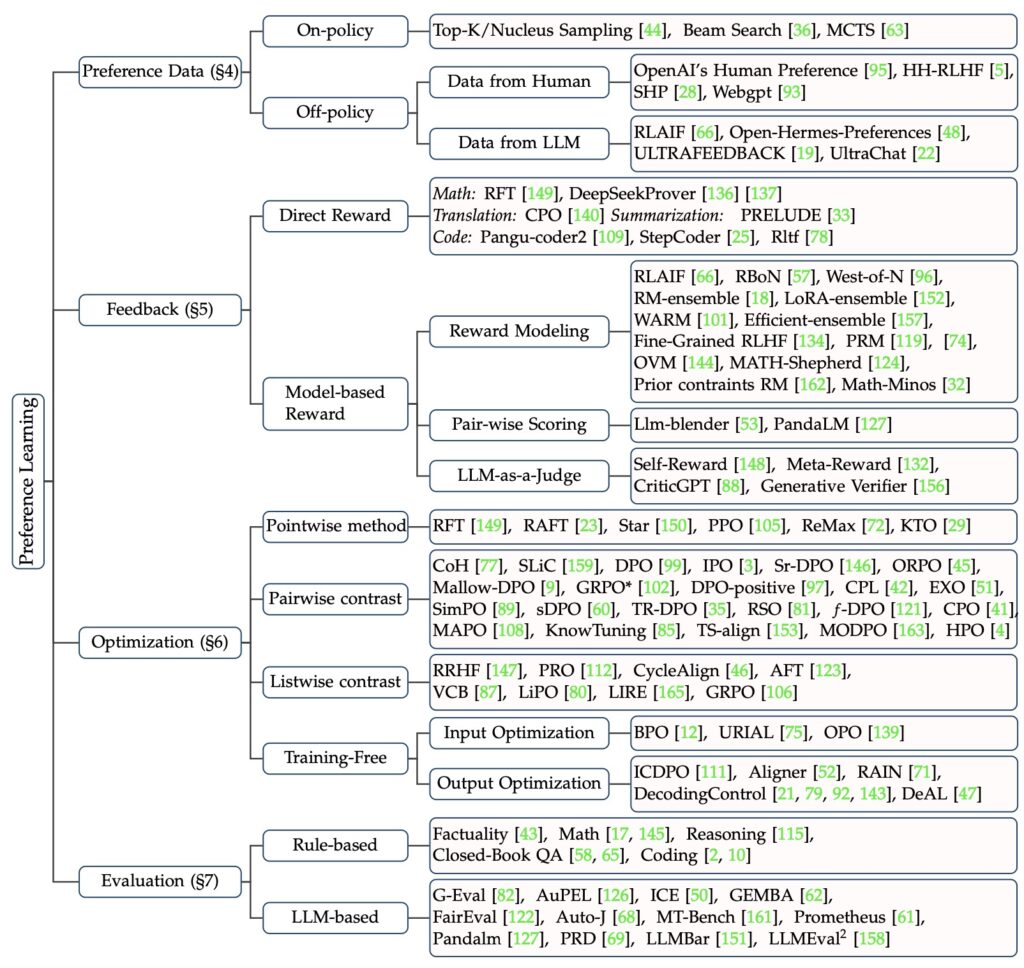
To facilitate a deeper understanding, the survey includes detailed examples of prevalent alignment algorithms. These examples illustrate how the core components are applied in practice, offering readers practical insights into the functioning of various methods. By presenting these working examples, the survey aims to bridge the gap between theoretical concepts and real-world applications, making the complexities of preference learning more accessible.
Looking ahead, the survey identifies several challenges and future research directions in the field of preference learning. Despite the progress made, significant questions remain regarding the effectiveness of different alignment strategies in various contexts. The survey highlights the need for further investigation into which methods perform best under specific conditions and how emerging technologies might influence the development of new alignment techniques. By providing this comprehensive overview, the survey seeks to inspire further research and innovation in the quest to better align LLMs with human values.