A New Benchmark for Realistic Human Image Animation and Camera Control
- HumanVid introduces the first large-scale, high-quality dataset tailored for human image animation, combining real-world and synthetic data.
- The dataset includes comprehensive annotations for both human and camera motions, addressing significant gaps in current animation models.
- The baseline model, CamAnimate, trained on HumanVid, achieves state-of-the-art performance in animating human poses with dynamic camera movements.
Human image animation, the process of generating videos from static character photos, has significant potential for video and movie production. However, existing methods often lack high-quality datasets and neglect the crucial element of camera motion, resulting in limited control and unstable video generation. To address these challenges, researchers have introduced HumanVid, a comprehensive dataset designed to enhance the realism and controllability of human image animations.
The Need for HumanVid
Recent advancements in video generation have primarily focused on 2D human motion, often overlooking the importance of camera movements in creating dynamic and engaging videos. Current benchmarks fail to provide the necessary data for evaluating compositional text-to-video generation, limiting the development of more sophisticated models. HumanVid aims to fill this gap by offering a dataset that includes high-quality real-world and synthetic videos with detailed annotations for both human and camera motions.
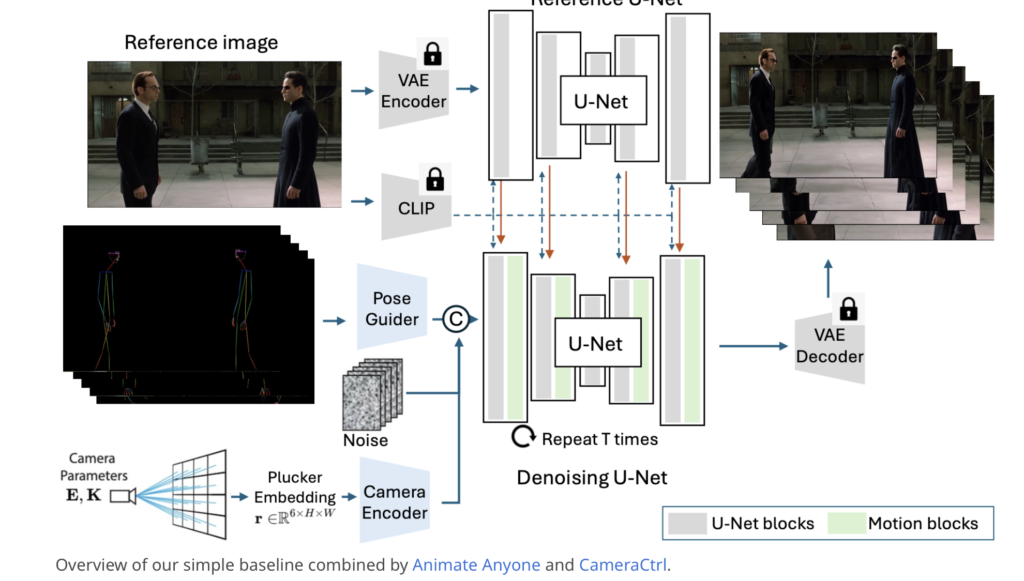
Building the Dataset
HumanVid comprises two main components: real-world videos and synthetic data. The real-world dataset is sourced from copyright-free internet platforms, resulting in a collection of 20,000 human-centric videos in 1080P resolution. These videos undergo a rigorous rule-based filtering process to ensure high quality, focusing exclusively on human and camera motions while eliminating noise from visual effects, occlusions, and object movements. Advanced techniques such as SLAM-based methods and precise pose estimators are used to annotate camera trajectories and human poses accurately.
The synthetic component of HumanVid includes 2,300 copyright-free 3D avatar assets, augmented with a rule-based camera trajectory generation method. This addition allows for diverse and precise camera motion annotations, rarely found in real-world data. The combination of these datasets provides a robust foundation for training models capable of handling complex animations with high visual fidelity.
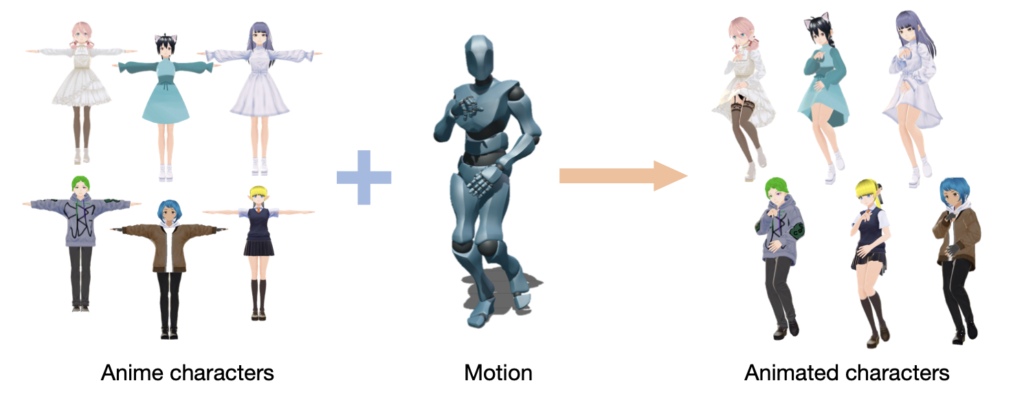
Introducing CamAnimate
To validate the effectiveness of HumanVid, the researchers developed CamAnimate, a baseline model designed to consider both human and camera motions. Extensive experiments demonstrate that CamAnimate, trained on HumanVid, outperforms existing models in generating realistic and controllable human animations. The model’s ability to animate characters from new viewpoints with dynamic camera movements marks a significant advancement in the field.
CamAnimate leverages the detailed annotations provided by HumanVid to achieve state-of-the-art performance. By integrating human and camera motions as conditions, the model produces videos that accurately reflect the input prompts, setting a new benchmark for human image animation.
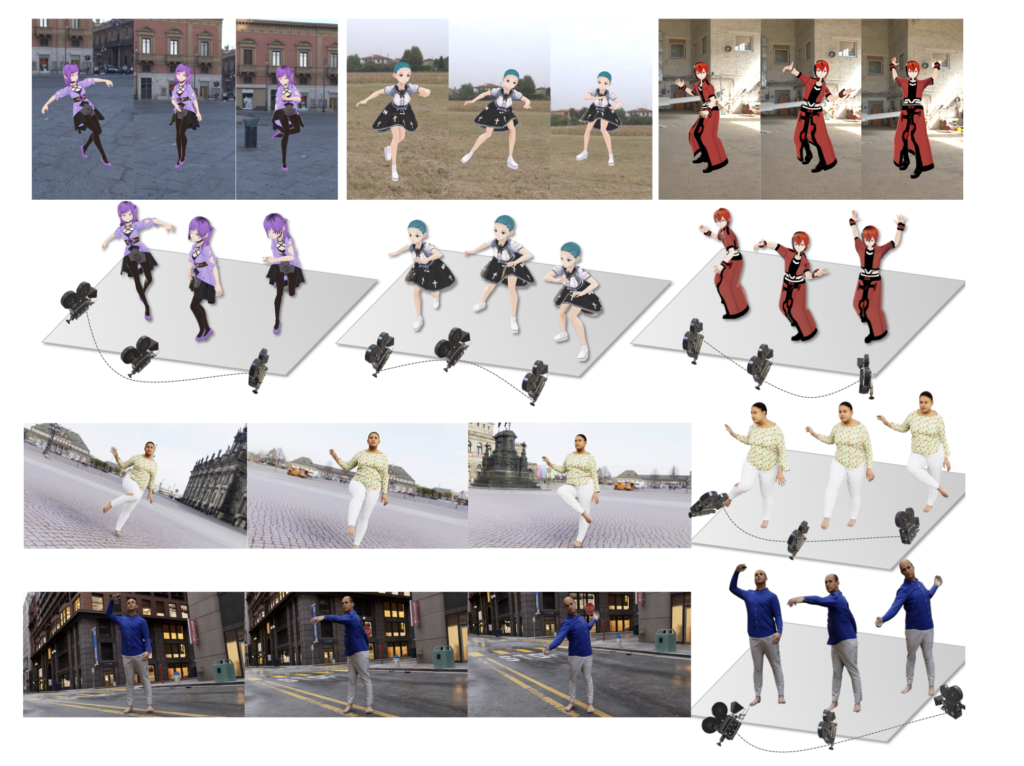
Impact and Future Directions
The introduction of HumanVid addresses critical limitations in current human image animation techniques. By providing a high-quality dataset with comprehensive annotations, it paves the way for more transparent and fair benchmarking in the field. Researchers and developers can now evaluate their models more effectively, fostering further advancements and innovations in video and movie production.
The potential applications of HumanVid and CamAnimate extend beyond entertainment. Imagine recreating iconic movie performances using just a single photo of the characters, or generating realistic training simulations for various industries. The possibilities are vast, and HumanVid’s contributions are set to drive significant progress in these areas.
Looking ahead, the researchers plan to continue refining HumanVid, incorporating more diverse scenes and improving annotation techniques. They also aim to explore the integration of multimodal data, such as audio and text, to enhance the realism and interactivity of generated videos.
HumanVid represents a significant step forward in the field of human image animation, addressing key challenges and setting new standards for quality and control. By combining real-world and synthetic data with detailed annotations for both human and camera motions, it provides a robust foundation for developing advanced animation models. The success of CamAnimate, trained on HumanVid, underscores the dataset’s potential to drive innovation and improve the realism of human animations, marking a new era in video and movie production.