Leveraging Large Language Models for Contextual Learning and Iterative Optimization in Medical Imaging Reports
Key Points:
- ImpressionGPT leverages ChatGPT’s in-context learning capability, utilizing dynamic prompts and domain-specific, individualized data.
- An iterative optimization algorithm is employed to improve generated impression results by providing automated evaluation feedback.
- ImpressionGPT achieves state-of-the-art performance on both MIMIC-CXR and OpenI datasets without requiring additional training data or fine-tuning.
- This approach demonstrates potential applicability in various domains, bridging the gap between general-purpose LLMs and specific language processing needs.
ImpressionGPT is a proposed model that aims to improve radiology report summarization by leveraging the in-context learning capabilities of Large Language Models (LLMs) like ChatGPT. This is achieved by constructing dynamic contexts using domain-specific, individualized data. The model is further optimized using an iterative optimization algorithm, which performs automatic evaluation of generated impression results and composes corresponding instruction prompts for improvement.
ImpressionGPT’s dynamic prompt approach enables the model to learn contextual knowledge from semantically similar examples found within existing data. The iterative optimization method improves generated results by providing automated evaluation feedback, along with instructions for desired responses. The model achieves state-of-the-art performance on both MIMIC-CXR and OpenI datasets without the need for additional training data or fine-tuning of LLMs.
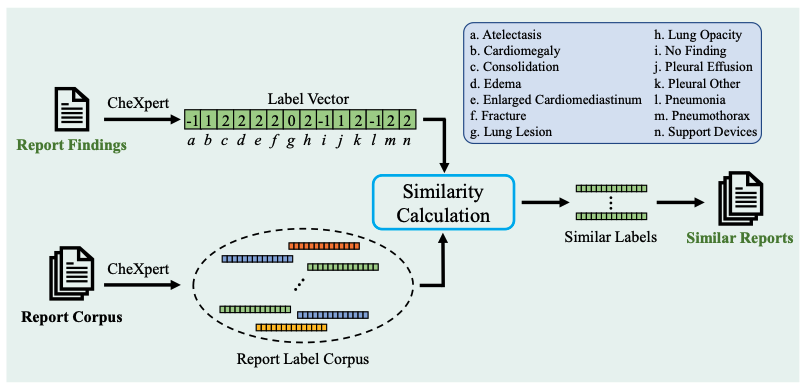
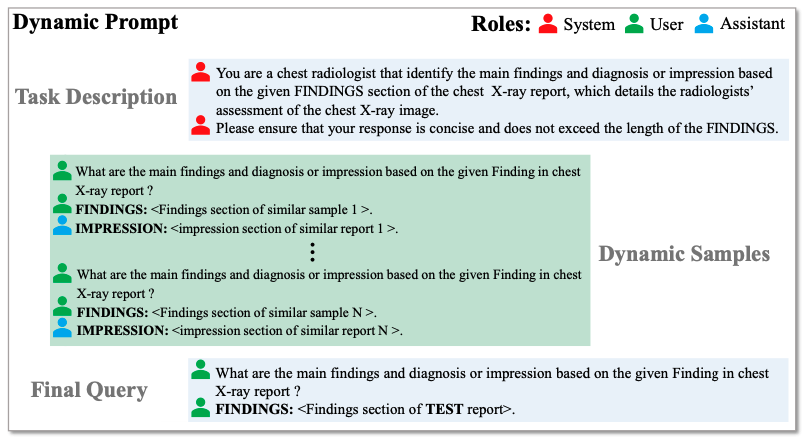
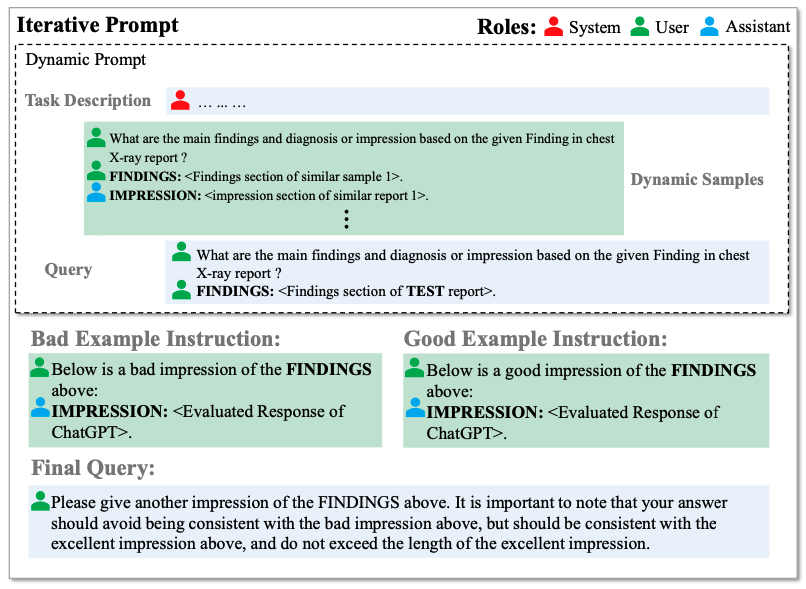
This groundbreaking work presents a paradigm for localizing LLMs that can be applied across a wide range of application scenarios, addressing the gap between general-purpose LLMs and the specific language processing needs of various domains. The approach can be further improved by refining the prompt design to incorporate domain-specific data, addressing privacy concerns in multi-institution scenarios, and integrating knowledge graphs to conform to existing domain knowledge.
Future work will also explore the potential of human-in-the-loop approaches, introducing radiologists and other domain experts to the iterative optimization process. By incorporating human evaluations and input, the model can be fine-tuned to better align with expert opinions and decisions, offering enhanced performance in radiology report summarization and other domain-specific language processing tasks.