A Leap Forward in Human Motion Generation with Enhanced Personalization
- Enhanced Individualization in Motion: in2IN introduces a novel diffusion model that conditions human-human motion generation not only on general interaction descriptions but also on specific actions of each individual involved, enhancing the personalization of generated movements.
- DualMDM for Diverse Dynamics: The introduction of DualMDM, a model composition technique, allows for greater diversity in individual motions and improved control over intra-person dynamics, making the generated interactions more realistic and varied.
- State-of-the-Art Performance: Utilizing advanced techniques and a large language model to enrich the InterHuman dataset, in2IN has achieved state-of-the-art results in motion generation, setting a new benchmark in the field.
The field of human motion generation has witnessed significant advancements with the introduction of in2IN, a cutting-edge artificial intelligence model designed to generate human interactions based on textual descriptions. This model represents a pivotal step in the evolution of generative AI, particularly in applications demanding high fidelity in human motion such as robotics, gaming, animation, and the burgeoning metaverse.
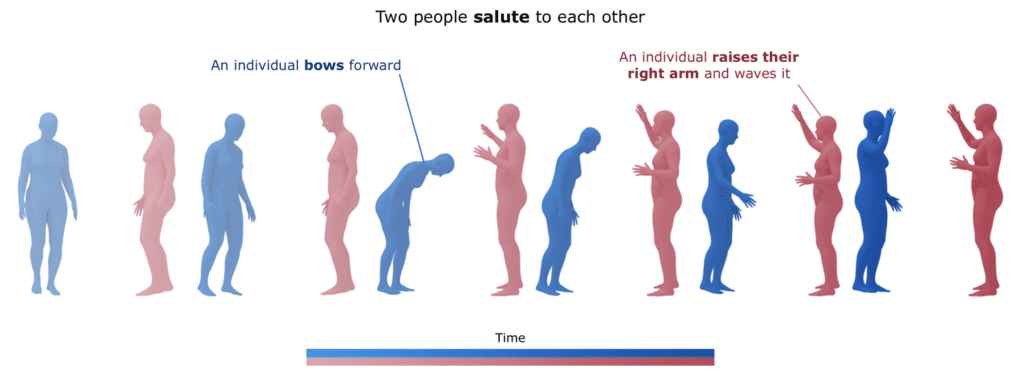
Innovative Approach to Motion Generation
in2IN stands out by incorporating individual descriptions of each person’s actions within a given interaction, allowing for a more nuanced generation of human movements. This method addresses the longstanding challenge of capturing the complex dynamics of interpersonal interactions and the inherent diversity of individual actions. By training the model with enriched data from the InterHuman dataset, in2IN not only enhances the accuracy of motion generation but also ensures a broader applicability across different scenarios and environments.
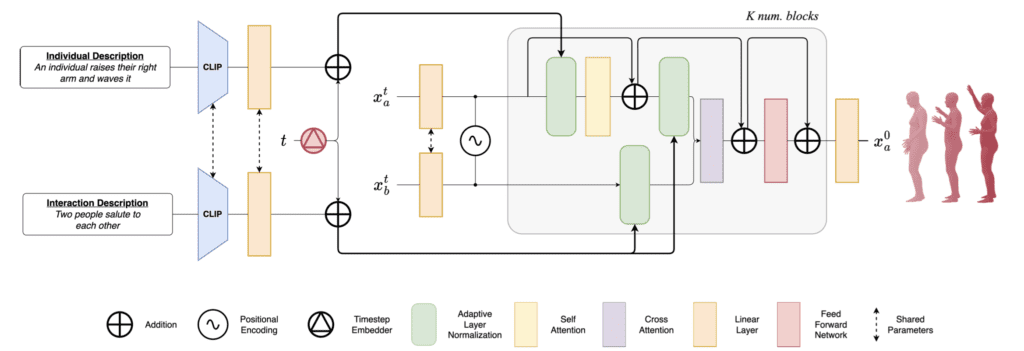
Dual Model Composition Technique
The DualMDM technique introduced alongside in2IN furthers the capability of the model by integrating motions generated from a single-person motion prior, which has been pre-trained on the HumanML3D dataset. This integration facilitates a richer diversity in motion dynamics, providing enhanced control over the movements of each individual within the interaction. The result is a more lifelike simulation of human interactions, where the nuances of individual behaviors are accurately depicted.
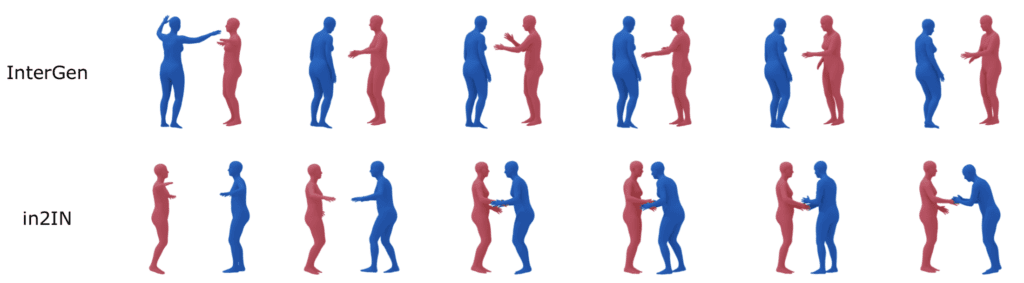
Achievements and Future Prospects
in2IN’s performance on the InterHuman dataset has set new standards in the field, demonstrating the effectiveness of its approach through improved metrics on various benchmarks. Looking ahead, the developers of in2IN plan to explore more sophisticated methods for generating individual descriptions that better match the generated motions. Additionally, future iterations will aim to refine the blending strategies used in DualMDM, potentially automating the adjustment of model outputs based on the dynamics of the interaction and individual variability.
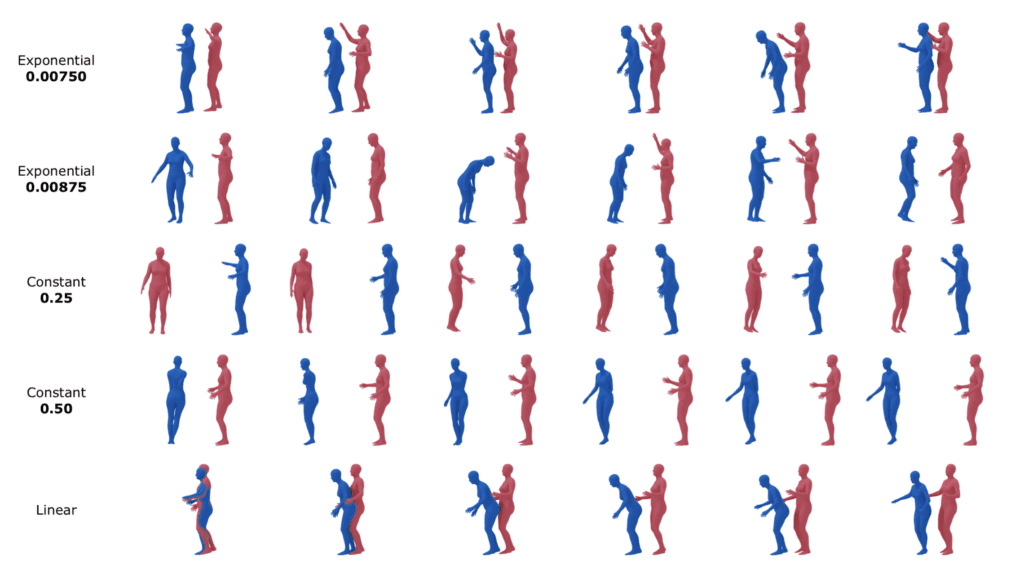
With its innovative approach to motion generation and the introduction of DualMDM, in2IN is poised to revolutionize the way AI interprets and generates human interactions. This technology not only enhances the realism and applicability of generated motions but also opens new possibilities for personalized interactions in digital environments. As the technology matures, it is expected to become an integral part of various applications, offering a more dynamic and responsive user experience across multiple platforms.