Expanding the Horizons of AI Comprehension and Memory
- Innovative Memory Management: Infini-attention introduces a compressive memory technique that allows LLMs to retain and access information from extremely long input sequences, addressing the memory limitations of traditional Transformer models.
- Enhanced Contextual Understanding: By preserving attention memory across old context windows in compressed key-value states, Infini-attention enables LLMs to maintain an ongoing contextual understanding without the typical constraints of fixed-size windows.
- Potential Applications and Implications: This breakthrough could significantly impact tasks requiring deep contextual awareness, such as document summarization and complex decision-making processes in AI, paving the way for more sophisticated and capable AI systems.
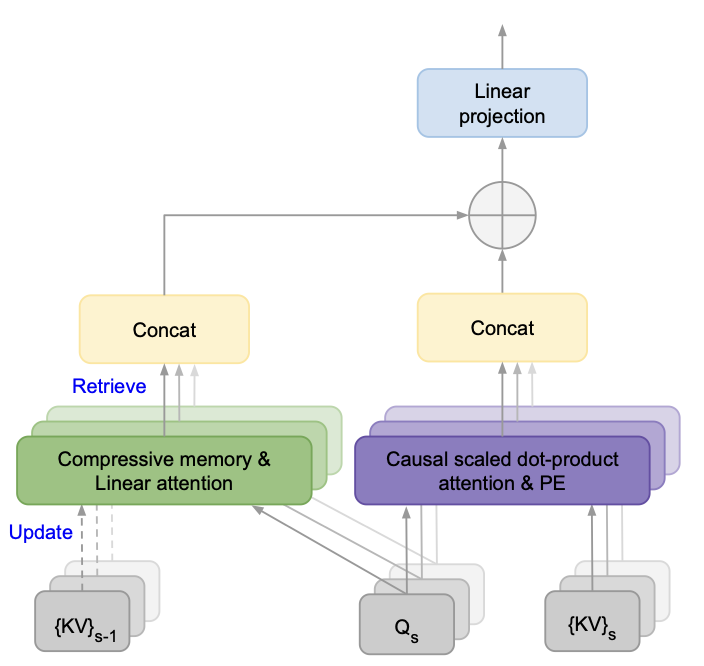
Google researchers have developed a groundbreaking technique known as Infini-attention, aimed at overcoming one of the significant limitations faced by current Large Language Models (LLMs)—the inability to manage long input sequences effectively. Traditional Transformer models, while effective in handling various tasks, struggle with memory management when dealing with extensive data. They typically discard old context to accommodate new information, thus losing valuable insights from earlier data.
Infini-attention addresses this issue by integrating a novel compressive memory system into the Transformer’s attention mechanism. This system preserves the memory of old context windows in a compressed format, allowing the model to access a complete record of previous information even as new data is processed. This capability of handling an “infinite context” without losing performance could revolutionize how LLMs understand and interact with complex datasets.
The mechanism works by maintaining both local and global attention spans where local attention deals with immediate data, and global attention, enhanced through a compression technique, maintains continuity across the entire dataset. Such a configuration not only improves the model’s efficiency and scalability but also enhances its ability to perform tasks that require understanding of extensive or complete datasets, such as analyzing long texts, synthesizing information from large documents, or continuous learning from sequential data without reset.
The implications of Infini-attention are vast. In academic and professional settings, it could improve AI’s ability to summarize lengthy academic papers or manage extensive legal and medical records. In customer service, it could enhance chatbots’ ability to understand and recall details from long interactions, providing responses and support based on comprehensive historical context.
This innovation sets the stage for new AI capabilities where understanding and memory are no longer bottlenecked by the technology’s architectural constraints. As LLMs continue to evolve, Infini-attention may well become a standard feature, fundamentally changing the landscape of artificial intelligence by making it more adept at mimicking true human-like understanding and memory retention.