Leveraging AI to Synthesize a New Dataset for Enhanced Image Editing Models
- Innovative Dataset Creation: HQ-Edit introduces a new way of building image editing datasets by leveraging AI models, GPT-4V and DALL-E 3, to automate the generation and expansion of image data.
- Enhanced Evaluation Metrics: The study proposes new metrics, Alignment and Coherence, to quantitatively assess the quality of edits, enhancing the reliability of automated image editing.
- Benchmark Setting Performance: Models trained on HQ-Edit achieve breakthrough performance, demonstrating improvements over previous methods that relied on human-annotated data.
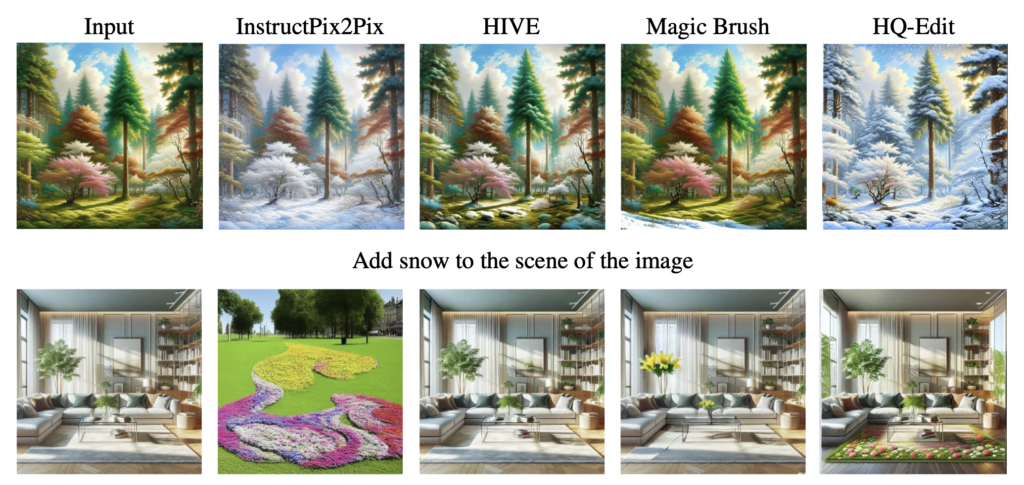
HQ-Edit represents a significant advancement in the field of AI-driven image editing, providing a high-quality dataset that empowers newer and more effective editing models. This dataset, comprised of around 200,000 image edits, diverges from traditional methods by utilizing an AI-centric approach for data generation and refinement.
Dataset Generation with AI
The core innovation of HQ-Edit lies in its unique data collection pipeline. Utilizing the capabilities of advanced foundation models, specifically GPT-4V and DALL-E 3, the researchers have developed a scalable method to automatically generate, rewrite, and expand seed image data. This process ensures a robust dataset that includes detailed diptychs of input and output images, paired with precise editing instructions. These diptychs are further refined through meticulous post-processing to ensure high fidelity and alignment with the intended edits.
Advanced Evaluation Techniques
To maintain and assure the quality of the dataset, the study introduces two novel evaluation metrics: Alignment and Coherence. These metrics are designed to quantitatively assess how well the edited images adhere to the provided instructions and how coherent the image content remains post-editing. Such metrics are crucial for validating the effectiveness of image editing models trained on the HQ-Edit dataset.
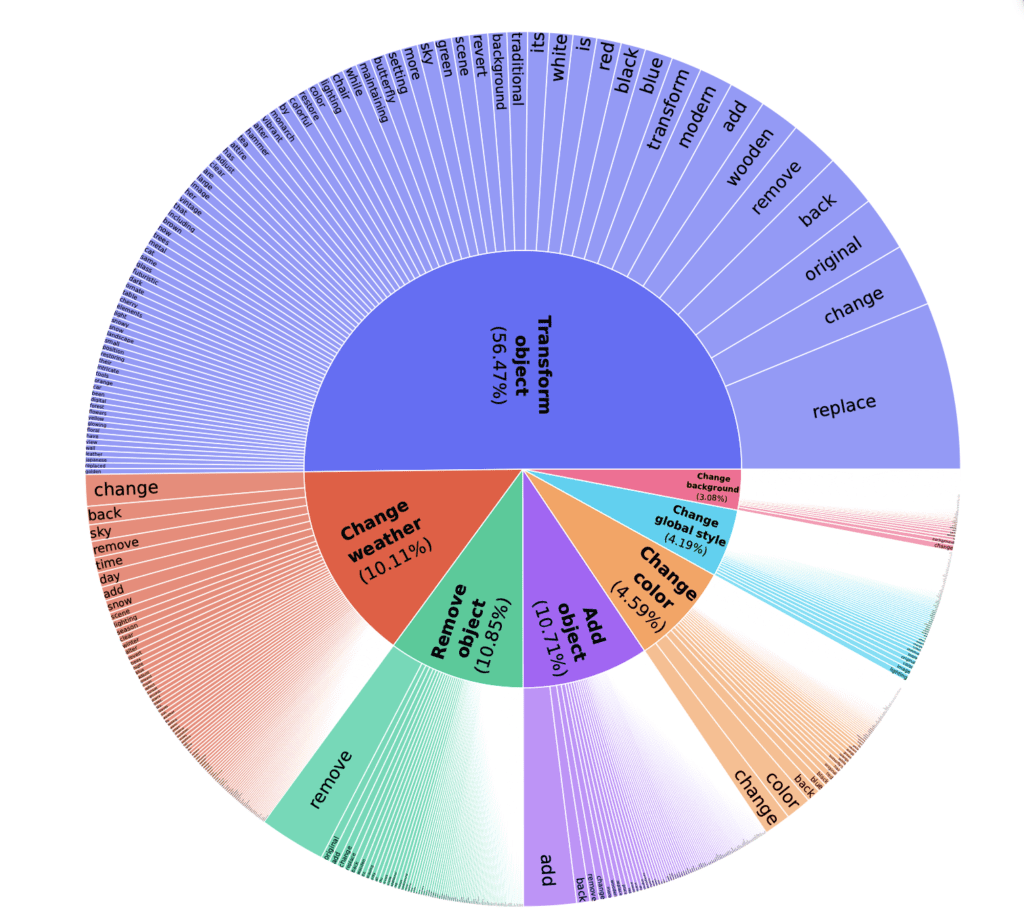
Performance Benchmarks
The practical implications of the HQ-Edit dataset are profound. When models such as InstructPix2Pix are fine-tuned on HQ-Edit, they achieve superior performance, setting new benchmarks in the realm of instruction-based image editing. This represents a notable leap forward from previous models trained on human-annotated datasets. The enhanced capabilities of these models demonstrate HQ-Edit’s potential to significantly advance the field, offering more precise and contextually accurate edits than ever before.
The introduction of HQ-Edit marks a transformative step in image editing technology. By synthesizing a large-scale, high-quality dataset through automated AI processes, and by establishing rigorous metrics for evaluation, the researchers have not only set new performance standards but also proposed a model that could streamline and enhance the development of future image editing tools. This study showcases the growing synergy between artificial intelligence and creative processes, opening up new avenues for both technological innovation and artistic expression in digital media.