Transforming Video Generation for Enhanced AI Interactivity
- Scalable Autoregressive Transformer: iVideoGPT integrates multimodal signals into a sequence of tokens for interactive AI experiences.
- Compressive Tokenization Technique: Efficiently discretizes high-dimensional visual data, facilitating large-scale pre-training.
- Versatile Foundation for AI Tasks: Enables action-conditioned video prediction, visual planning, and model-based reinforcement learning.
ing and action-conditioned video prediction. By pre-training on a vast dataset comprising millions of human and robotic manipulation trajectories, iVideoGPT establishes a robust foundation adaptable to various downstream tasks, making it a versatile tool in AI development.
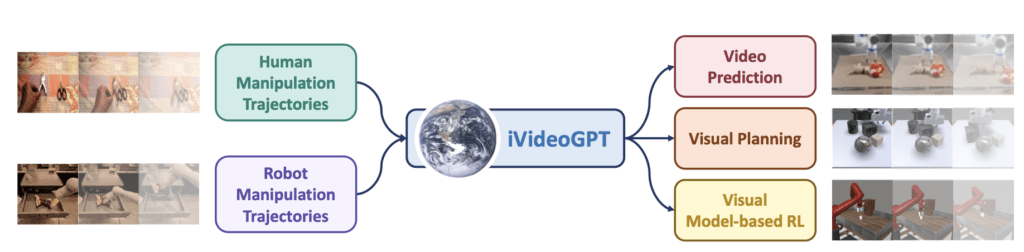
Compressive Tokenization Technique
One of the critical innovations in iVideoGPT is its compressive tokenization technique, which discretizes high-dimensional visual observations into manageable tokens. This approach significantly enhances the model’s efficiency, enabling it to handle large-scale data without compromising performance. The tokenization process ensures that the model can retain essential information from initial video frames, providing a contextual basis for predicting future frames.
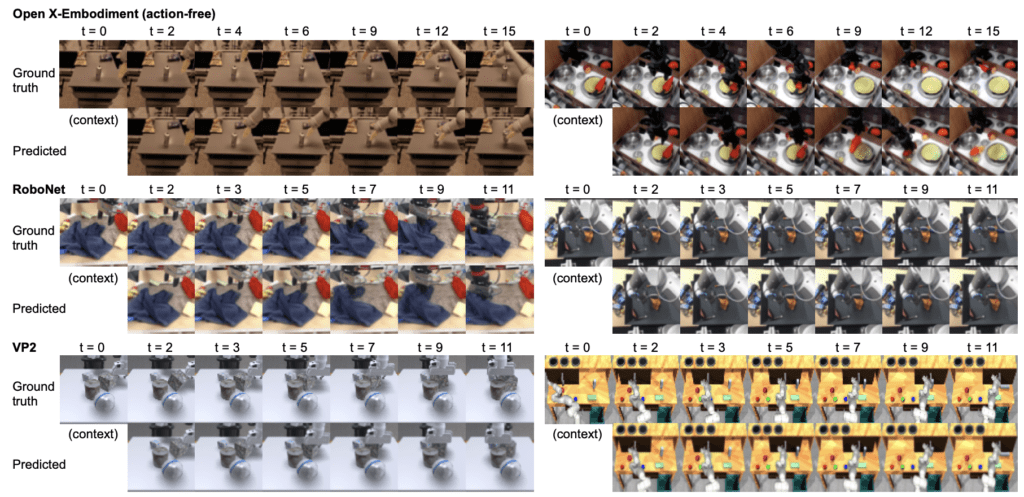
This efficiency is crucial for scaling the model across diverse datasets and applications. The ability to process extensive visual data quickly and accurately allows iVideoGPT to perform tasks like model-based reinforcement learning more effectively, reducing the number of real-world trials needed for AI agents to acquire new skills.
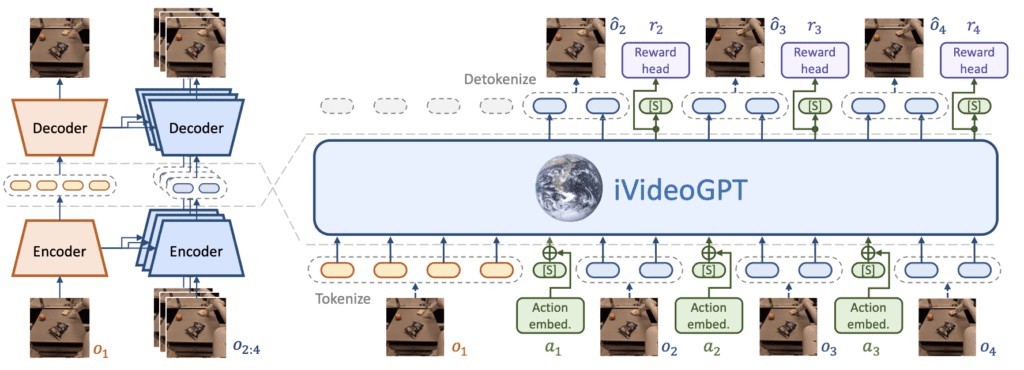
Versatile Foundation for AI Tasks
iVideoGPT’s design enables it to excel in various applications, making it a powerful foundation for AI tasks. Its ability to integrate and process multimodal signals allows it to perform accurate video predictions and support model-based planning. The model’s versatility is demonstrated through its competitive performance on benchmarks requiring diverse capabilities, proving its efficacy in both low-level control tasks and complex visual planning scenarios.
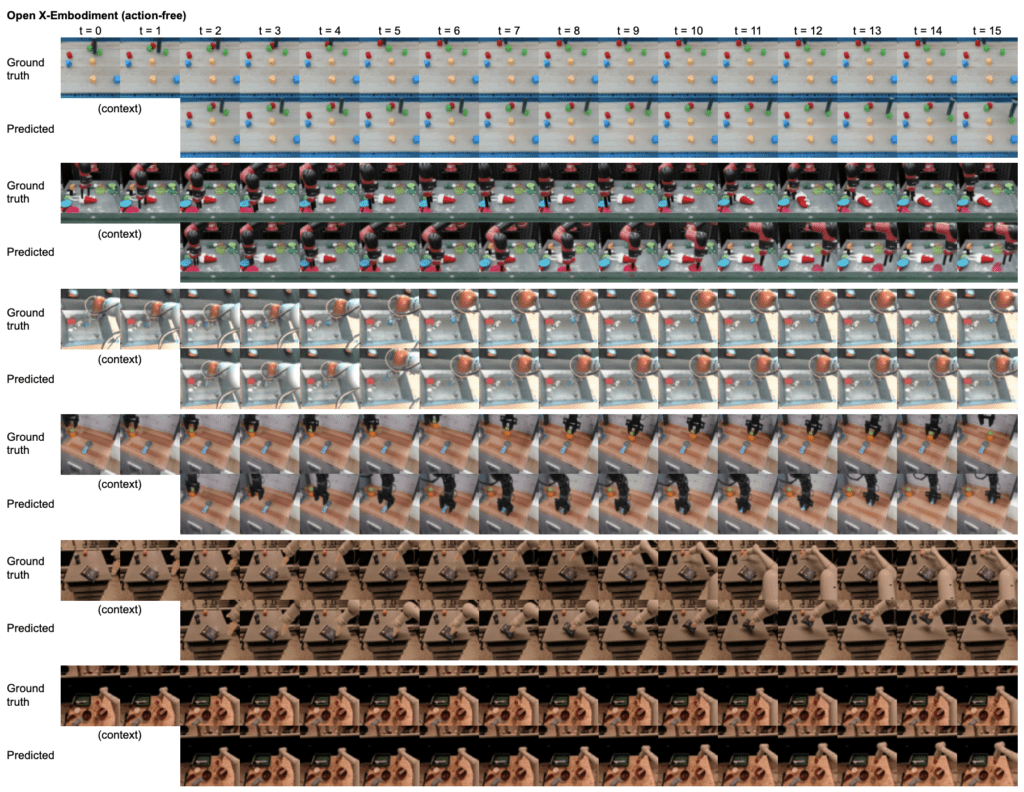
The model’s pre-training on human and robotic manipulation trajectories ensures that it accumulates a broad understanding of real-world interactions. This knowledge is crucial for tasks like action-conditioned video prediction, where the model anticipates future events based on specific actions taken by the agent. The result is a more interactive and responsive AI that can adapt to new situations with ease.
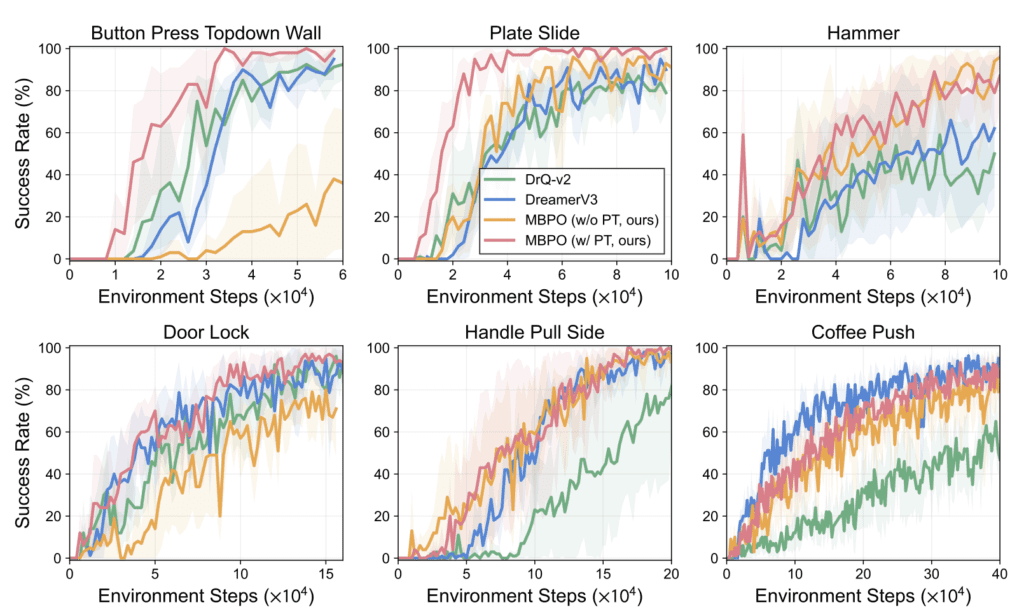
iVideoGPT is a pioneering framework that enhances the capabilities of generative video models through a scalable autoregressive transformer architecture. By efficiently integrating multimodal signals into a sequence of tokens, the model provides a rich interactive experience for AI agents. While there are areas for improvement, particularly in terms of data diversity and resource requirements, iVideoGPT sets a new standard for interactive world models. As researchers continue to refine and expand upon this model, it promises to unlock new possibilities for AI interaction and decision-making.