Unlocking Fine-Grained Control Over Visual Concepts with Component-Controllable Personalization
In the rapidly evolving world of text-to-image (T2I) diffusion models, a new frontier is emerging that promises to redefine how we generate and personalize images. Recent advancements have made it possible to create high-quality images from textual prompts, yet challenges remain in achieving precise control over specific visual elements. This article explores the groundbreaking framework, MagicTailor, which introduces component-controllable personalization in T2I models.
- Addressing Existing Challenges: MagicTailor tackles two significant obstacles in image generation: semantic pollution, which disrupts the integrity of personalized concepts, and semantic imbalance, which skews the learning of visual semantics.
- Innovative Framework: The framework incorporates Dynamic Masked Degradation (DM-Deg) to eliminate unwanted visual elements and Dual-Stream Balancing (DS-Bal) to ensure balanced learning of desired visual components, enabling more nuanced image customization.
- Practical Applications and Future Prospects: With its advanced capabilities, MagicTailor not only sets a new benchmark for T2I models but also opens doors for a range of creative applications in image and video generation.
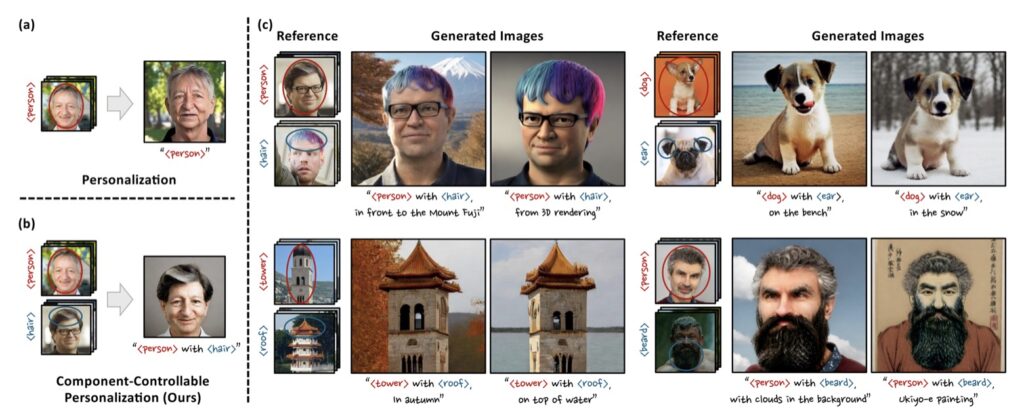
As T2I diffusion models become increasingly sophisticated, users seek greater control over the visual aspects of the generated images. Traditional models often rely on reference images to replicate concepts but fall short when it comes to fine-tuning individual components of those concepts. The inability to manipulate specific visual elements can lead to unwanted artifacts and a lack of coherence in personalized images. This gap in functionality has necessitated a new approach, leading to the development of MagicTailor, which empowers users to customize visual concepts with unprecedented precision.
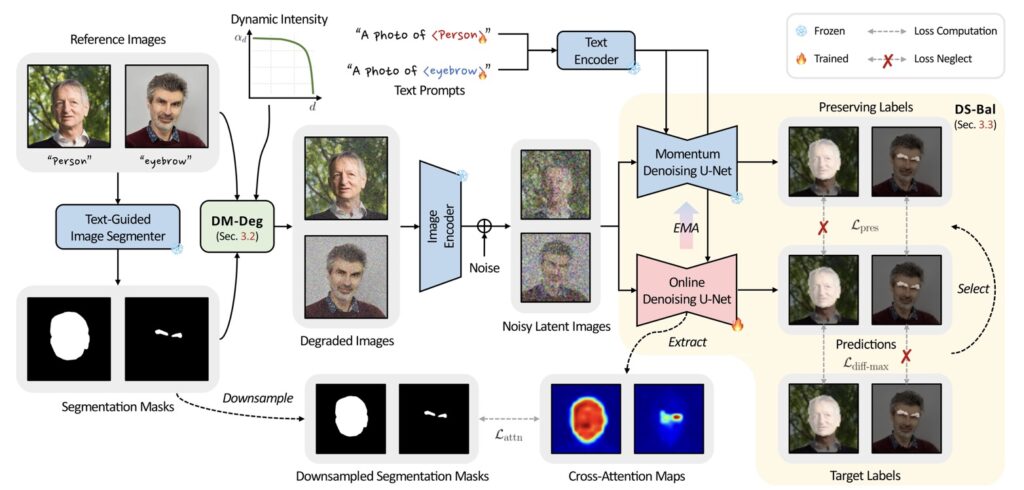
Overcoming Semantic Challenges
The MagicTailor framework specifically addresses two major hurdles: semantic pollution and semantic imbalance. Semantic pollution occurs when extraneous visual elements corrupt the intended concept, leading to unsatisfactory results. Conversely, semantic imbalance refers to the disproportionate learning of certain visual components, hindering the overall quality of image generation.
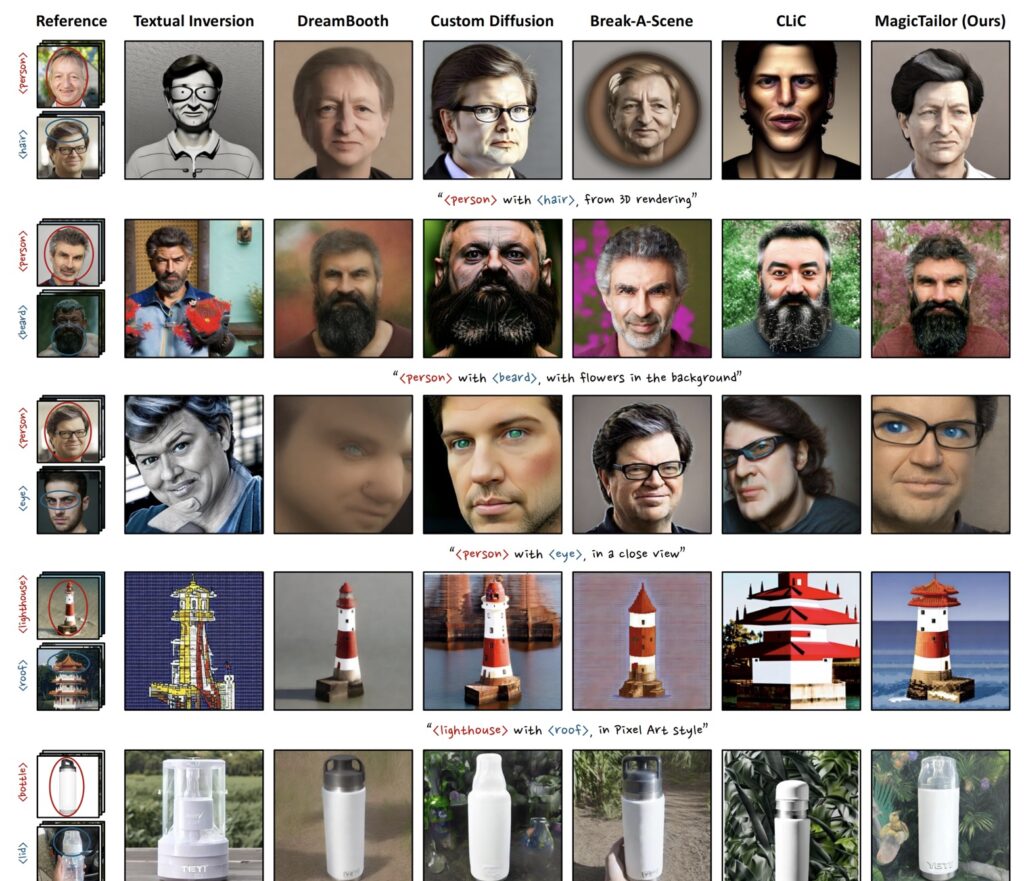
To combat these challenges, MagicTailor employs Dynamic Masked Degradation (DM-Deg), which effectively mitigates unwanted visual semantics by dynamically perturbing them. This mechanism ensures that only the desired components are emphasized, preserving the integrity of the personalized concept. Simultaneously, Dual-Stream Balancing (DS-Bal) establishes a balanced learning environment, allowing the model to focus equally on all components, leading to more coherent and visually appealing images.
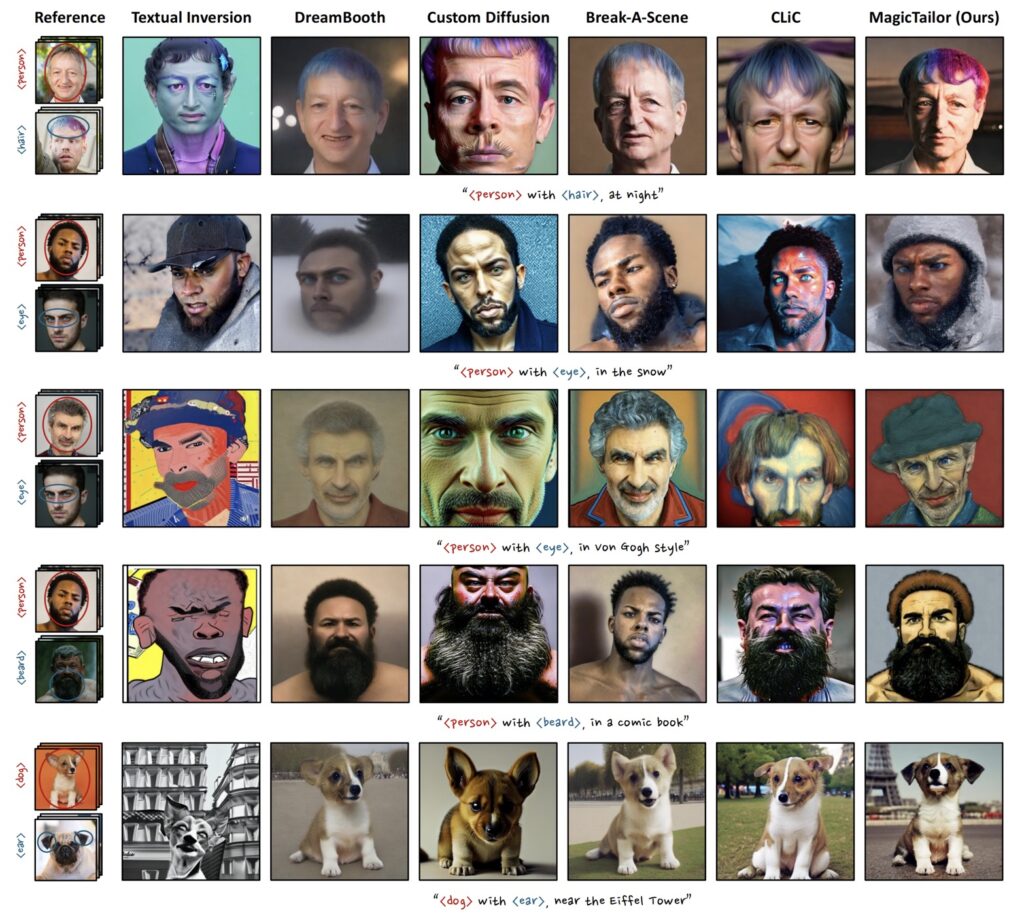
Comprehensive Experiments and Results
Extensive experiments conducted using the MagicTailor framework demonstrate its superior performance in the realm of component-controllable personalization. Through rigorous comparisons and ablation studies, the framework has been shown to excel in overcoming the challenges posed by semantic pollution and imbalance. The results not only set new benchmarks for T2I models but also highlight the framework’s potential for practical applications across various creative domains.
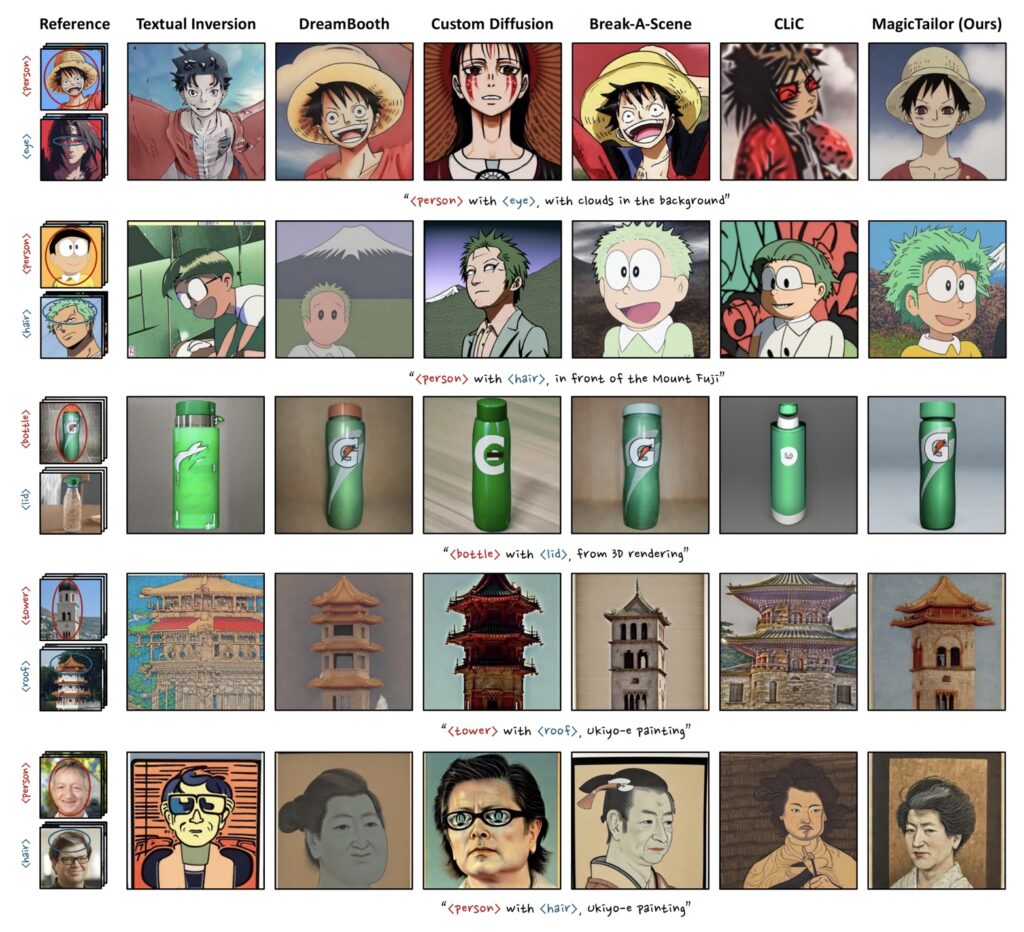
MagicTailor’s ability to generate nuanced and customized images opens up exciting possibilities for artists, designers, and content creators. Whether it’s tailoring images for marketing campaigns, creating personalized artwork, or developing visual narratives, the implications of this technology are vast and transformative.
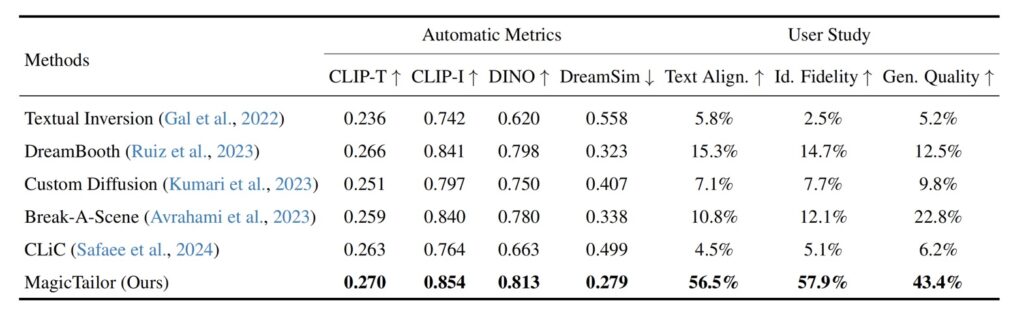
Future Directions and Broader Applications
Looking ahead, the creators of MagicTailor envision extending its capabilities to other areas of image and video generation. By exploring how multi-level visual semantics can be recognized, controlled, and manipulated, the framework could unlock even more sophisticated generative capabilities. This expansion could revolutionize fields such as virtual reality, gaming, and digital storytelling, providing creators with powerful tools to express their visions.
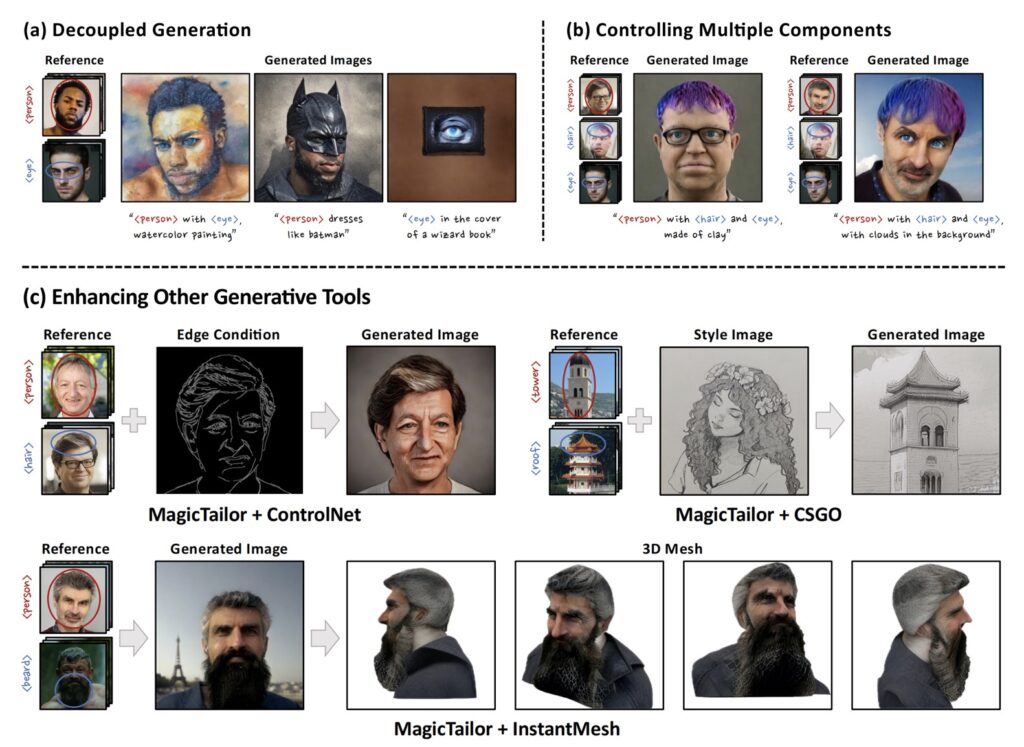
MagicTailor represents a significant leap forward in text-to-image diffusion models, enabling component-controllable personalization that was previously unattainable. By addressing critical challenges and offering innovative solutions, this framework paves the way for a new era of creative possibilities in visual content generation. As technology continues to evolve, the potential for MagicTailor and similar advancements will only grow, shaping the future of how we interact with and create visual media.