Harnessing AI for Advanced Crystal Generation through Natural Language and Diffusion Models
- Generative Hierarchical Materials Search (GenMS) represents a breakthrough in materials science by automating the creation of crystal structures from natural language descriptions.
- The innovative approach combines a language model, a diffusion model, and a graph neural network to efficiently generate and evaluate potential crystal structures.
- Despite its impressive capabilities, GenMS faces challenges in generating complex structures and ensuring synthesizability, highlighting areas for future development.
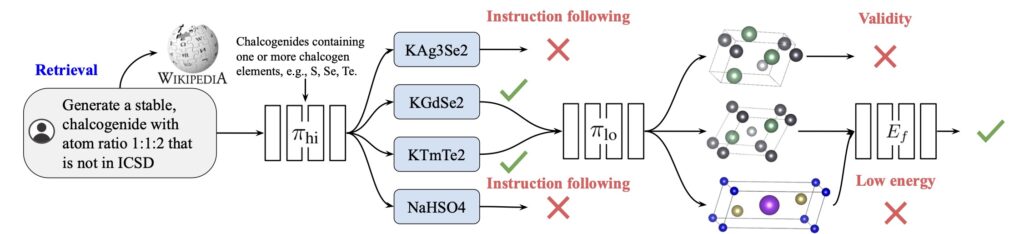
The field of materials science is undergoing a transformative shift with the advent of advanced generative models. These models, trained on extensive datasets, are increasingly capable of producing not only text and images but also scientific data such as crystal structures. The latest development in this space is Generative Hierarchical Materials Search (GenMS), a novel framework designed to bridge the gap between high-level language instructions and detailed crystal structure generation. This advancement promises to revolutionize how researchers design and discover new materials.
GenMS introduces a multi-faceted approach to generating crystal structures, combining a language model, a diffusion model, and a graph neural network. The language model interprets natural language inputs to generate intermediate textual data about crystals, such as chemical formulae. This information is then fed into a diffusion model, which translates it into detailed, continuous-value crystal structures. Additionally, a graph neural network predicts material properties like formation energy from these generated structures. This integrated system allows GenMS to perform an efficient forward tree search over the possible crystal structures, ensuring both adherence to user specifications and the production of low-energy structures.
The power of GenMS lies in its ability to interpret natural language prompts to generate specific crystal structures. For instance, users can input a description such as “a stable chalcogenide with atom ratio 1:1:2 that is not on ICSD,” and GenMS will produce candidate structures that match these criteria. This capability is a significant advancement over previous methods, which often required either a vast number of samples or pre-defined chemical formulas. By enabling users to specify desired characteristics in plain language, GenMS democratizes access to advanced materials design and speeds up the discovery process.
Despite its innovative approach, GenMS is not without limitations. The current system excels at generating simpler crystal structures but struggles with more complex ones like Mxenes or Kagome lattices. Additionally, while GenMS effectively generates structures that meet user specifications, the actual synthesis of these materials remains unverified. Ensuring that generated structures are synthesizable and exploring applications beyond crystal structures, such as molecules and proteins, are areas for future development.
Generative Hierarchical Materials Search (GenMS) marks a significant leap in the intersection of AI and materials science. By enabling the generation of crystal structures from natural language descriptions, GenMS offers a powerful tool for researchers and scientists, streamlining the process of materials design and discovery. As the technology continues to evolve, addressing its current limitations will be crucial for fully realizing its potential and expanding its applications in the field.