How Multi-Image Synthetic Data and Shared Attention Mechanisms Are Redefining AI-Generated Imagery
- Synthetic Dataset Innovation: A new Synthetic Customization Dataset (SynCD) leverages 3D assets and text-to-image models to generate multiple images of the same object in diverse settings, addressing the lack of multi-image supervision in existing methods.
- Advanced Encoder Architecture: A novel shared attention mechanism enhances the model’s ability to incorporate fine-grained visual details from input images, improving image quality and customization accuracy.
- Inference Breakthrough: A new normalization technique for text and image guidance vectors mitigates overexposure issues during inference, ensuring higher-quality outputs without costly per-object optimization.
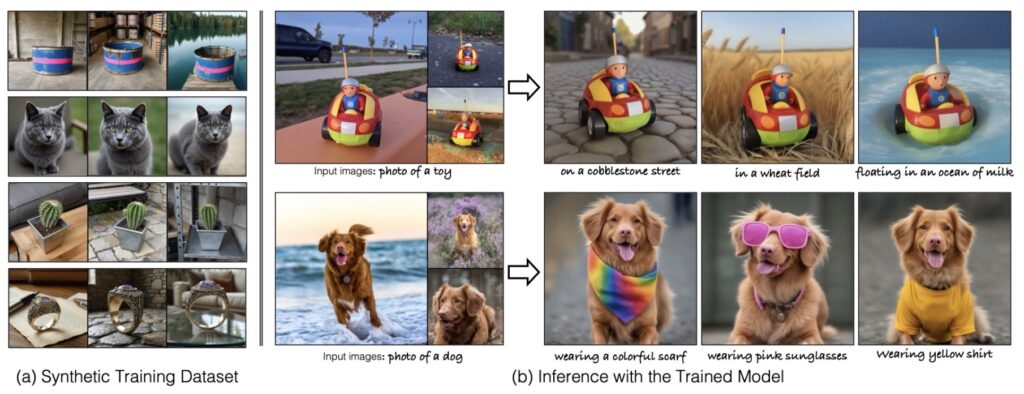
The field of text-to-image generation has made remarkable strides in recent years, with models capable of producing high-fidelity images from simple text prompts. However, these models often struggle to capture the intricate visual details of real-world objects, especially when users want to generate personalized images of specific items in new contexts. Enter the emerging field of model customization, which allows users to insert custom concepts—like a unique toy or a cherished artifact—and generate them in unseen settings, such as a wheat field or a bustling cityscape.
While early customization methods required costly and time-consuming optimization for each new object, recent encoder-based approaches have streamlined the process by enabling tuning-free generation. Yet, these methods still face significant limitations, including reliance on single-image datasets and subpar image quality. In this article, we explore a groundbreaking approach that addresses these challenges through synthetic data generation, advanced model architecture, and innovative inference techniques.
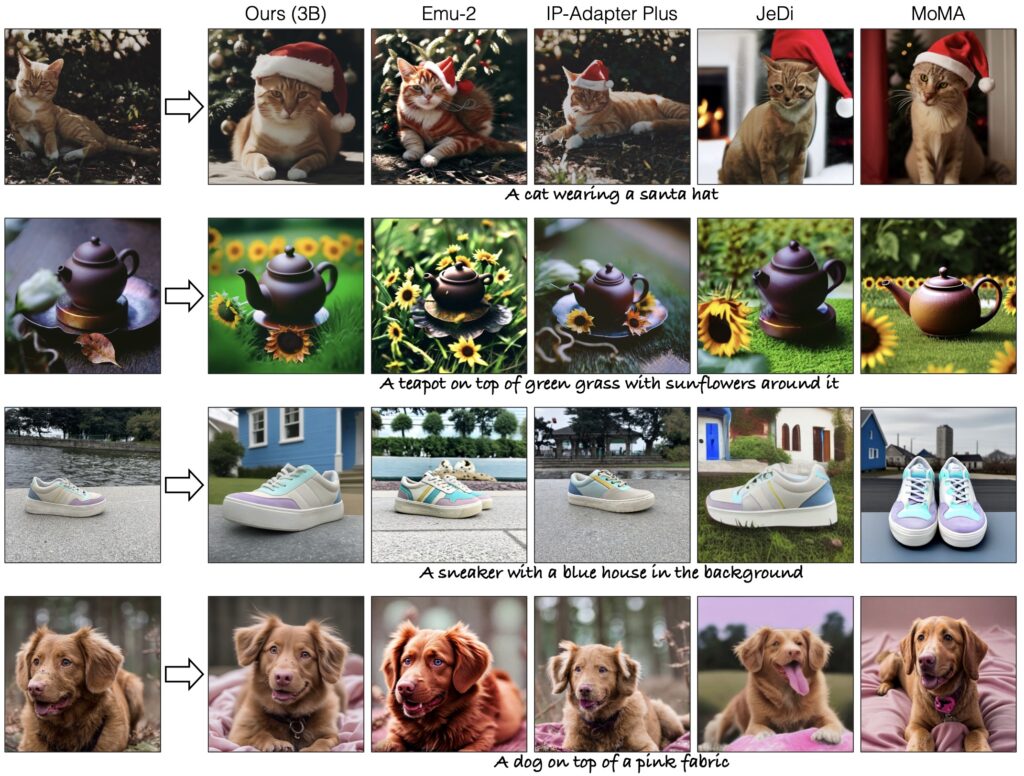
The Challenge of Customization in Text-to-Image Models
Text-to-image models excel at generating realistic images from text prompts, but they often fall short when it comes to customization. For instance, describing a unique toy with rich visual details—such as its texture, color, and shape—through text alone is nearly impossible. Early customization methods attempted to solve this by requiring users to provide multiple images of the object and undergo extensive optimization processes. While effective, these methods were impractical due to their high computational cost and slow performance.
Encoder-based methods emerged as a solution, enabling tuning-free customization by training models on reference images. However, these methods typically rely on single-image datasets, which lack the diversity needed to capture an object’s appearance across different lighting, backgrounds, and poses. This limitation results in lower image quality and reduced flexibility in generating new compositions.
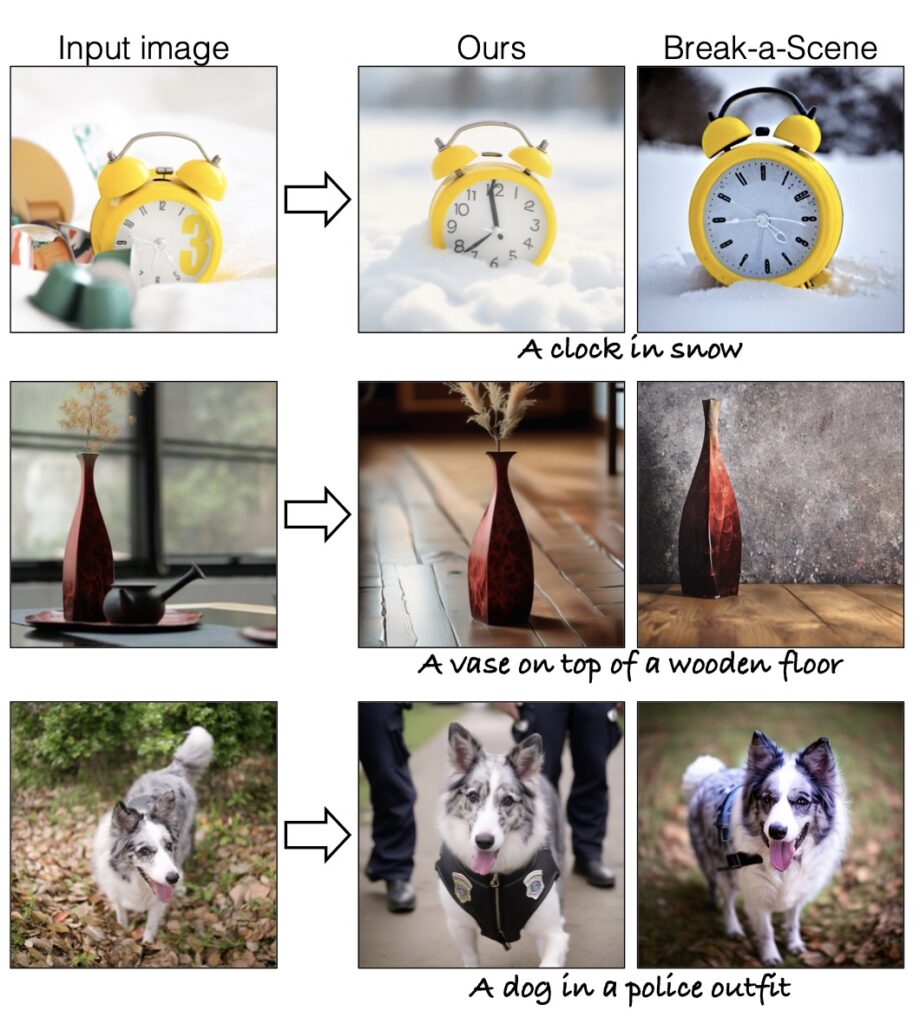
A Synthetic Solution: The SynCD Dataset
To overcome the lack of multi-image training data, we propose the Synthetic Customization Dataset (SynCD). This dataset is created by leveraging existing text-to-image models and 3D assets to generate multiple images of the same object in varied settings. For example, a 3D model of a toy can be rendered in different lighting conditions, placed against diverse backgrounds, and posed in various orientations. This approach ensures that the dataset captures the full spectrum of an object’s visual characteristics, providing the model with the rich, multi-image supervision it needs to excel.
The SynCD dataset not only addresses the data scarcity problem but also enhances the model’s ability to generalize across unseen scenarios. By training on this synthetic data, the model learns to generate high-quality images of custom objects without requiring costly per-object optimization.
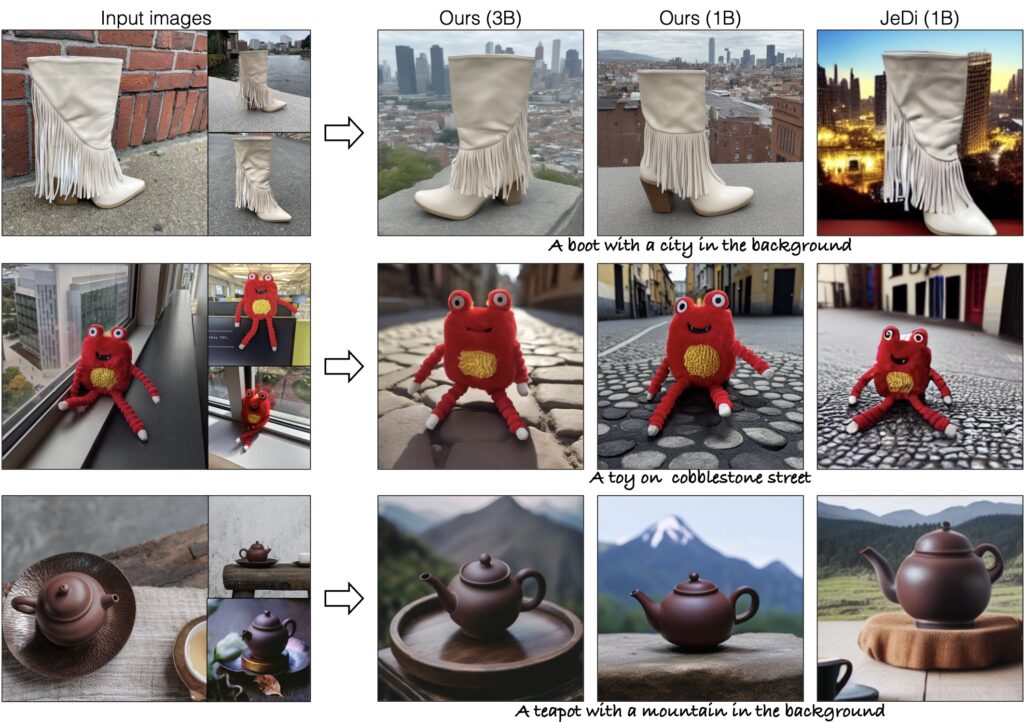
A Leap Forward in Model Architecture
In addition to the synthetic dataset, we introduce a novel encoder architecture based on shared attention mechanisms. Traditional encoders struggle to incorporate fine-grained visual details from input images, leading to a loss of quality in the generated outputs. Our shared attention mechanism solves this by enabling the model to focus on the most relevant visual features across multiple images, ensuring that the generated images retain the object’s unique characteristics.
This architectural innovation significantly improves the model’s performance, allowing it to outperform existing tuning-free methods on standard customization benchmarks. Moreover, it brings the model’s capabilities closer to those of time-consuming tuning-based approaches, making it a practical solution for real-world applications.
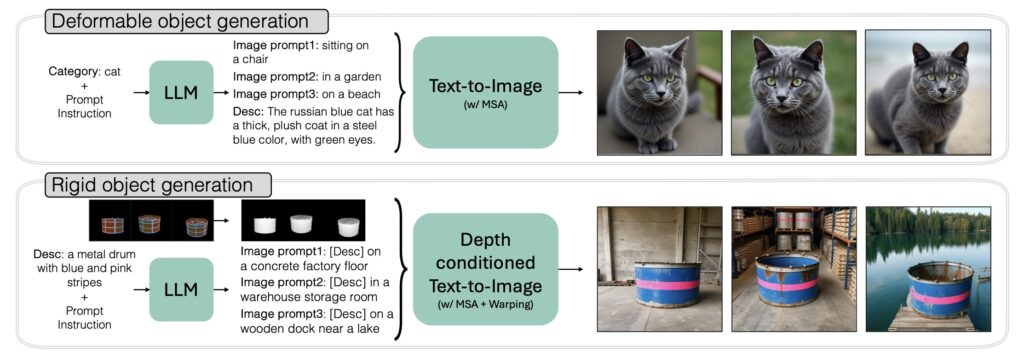
Tackling Overexposure: A New Inference Technique
During inference, text-to-image models often suffer from overexposure issues, where the generated images appear overly bright or washed out. To address this, we propose a new normalization technique for the text and image guidance vectors used in the inference process. By balancing these vectors, our method ensures that the generated images maintain realistic lighting and color balance, further enhancing their quality.
This inference technique, combined with the SynCD dataset and shared attention mechanisms, creates a robust pipeline for text-to-image customization. The result is a model that can generate high-quality, personalized images with minimal computational cost.
While our approach represents a significant advancement, it is not without limitations. For instance, the model may struggle with intricate textures or highly variable poses. Additionally, the quality of the synthetic dataset depends on the fidelity of the 3D assets used, which can sometimes be a bottleneck.
Future work could integrate recent advances in text-to-3D and video generative models to further enhance the dataset’s quality. By combining these technologies, we can create even more realistic and diverse training data, pushing the boundaries of what text-to-image customization can achieve.
The ability to generate personalized images from text prompts has immense potential, from creative applications to practical uses in design and marketing. Our approach—combining synthetic data generation, advanced encoder architecture, and innovative inference techniques—represents a major step forward in this field. By addressing the limitations of existing methods, we have created a model that is both powerful and practical, paving the way for a new era of text-to-image customization.
As AI continues to evolve, the integration of synthetic data and cutting-edge model architectures will undoubtedly play a crucial role in unlocking the full potential of text-to-image generation. The future of AI-generated imagery is bright, and we are just beginning to scratch the surface of what is possible.