Enhancing Image Generation through Targeted Denoising
- Introduction of Step-aware Preference Optimization (SPO): A novel post-training approach that refines each step of the denoising process, aligning it with human preferences.
- Performance and Efficiency: SPO significantly outperforms existing methods like Diffusion-DPO, improving image alignment with detailed prompts and aesthetics, while being 20 times more efficient in training.
- Innovative Evaluation Method: Incorporating a step-aware preference model and step-wise resampler ensures precise and effective optimization at every denoising step.
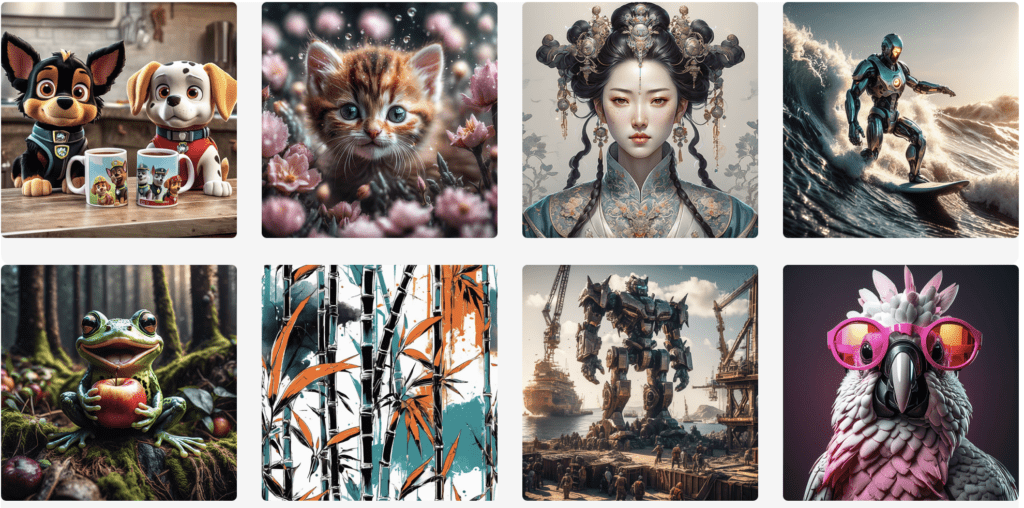
In the rapidly evolving landscape of text-to-image diffusion models, achieving alignment with human preferences remains a critical challenge. Direct Preference Optimization (DPO) has been a significant advancement, but its assumption that all diffusion steps share a consistent preference order has limitations. Addressing this, researchers have developed Step-aware Preference Optimization (SPO), a groundbreaking approach that refines the denoising performance at each step of the diffusion process, leading to superior image generation outcomes.
The Need for Step-specific Optimization
Traditional DPO methods uniformly apply preference signals across all intermediate steps in the diffusion process. However, diffusion models are inherently multi-step, with each step contributing differently to the final image. Early steps focus on generating the layout, while later steps add detailed textures. A uniform application of preference signals can therefore misalign the optimization process, as it fails to account for the varying importance and contribution of each step.
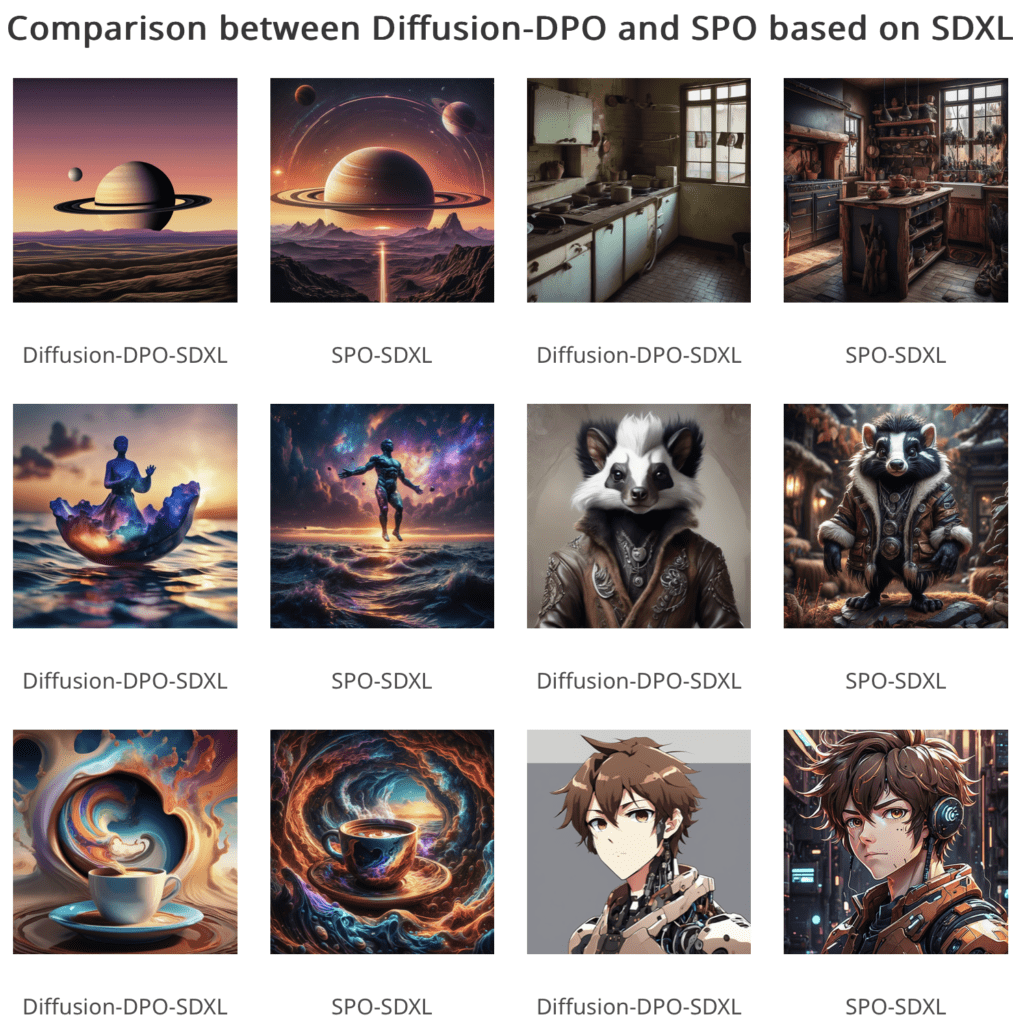
Introducing Step-aware Preference Optimization
SPO innovatively addresses this challenge by tailoring preference signals to each step’s contribution in the denoising process. This involves a step-aware preference model that evaluates and adjusts preferences independently at each step, ensuring that early steps receive signals to improve layout generation, while later steps focus on enhancing textures.
Key Components of SPO:
- Step-aware Preference Model: This model assesses preferences for both noisy and clean images at each denoising step, ensuring that the optimization aligns with the specific contribution of each step.
- Step-wise Resampler: This component samples a pool of images, selects a suitable win-lose pair, and initializes the next denoising step with a randomly selected image from the pool. This ensures independence from the previous step and maintains consistency in preference signals.
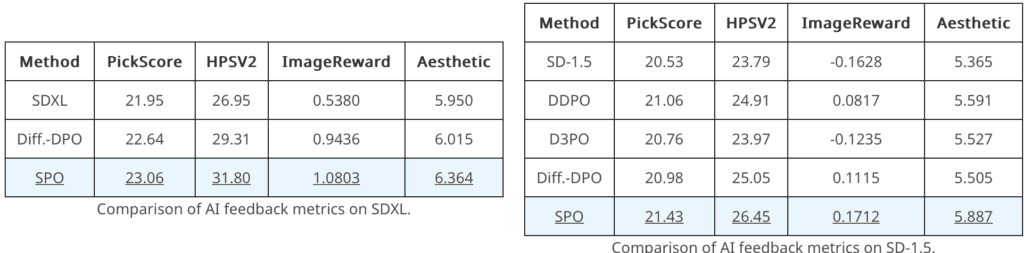
Advantages of SPO
Through extensive experiments using Stable Diffusion v1.5 and SDXL, SPO has demonstrated its superiority over existing Diffusion-DPO methods. Not only does it align generated images more closely with complex and detailed prompts, but it also enhances the aesthetic quality of the images. Moreover, the training efficiency of SPO is remarkable, achieving more than 20 times faster training compared to traditional methods, due to its precise and step-specific preference labeling.
Implications for Image Generation
The introduction of SPO marks a significant advancement in the field of text-to-image diffusion models. By recognizing and optimizing the unique contributions of each denoising step, SPO provides a more refined and effective approach to image generation. This ensures that the generated images are not only aligned with human preferences but also exhibit higher quality and finer details.
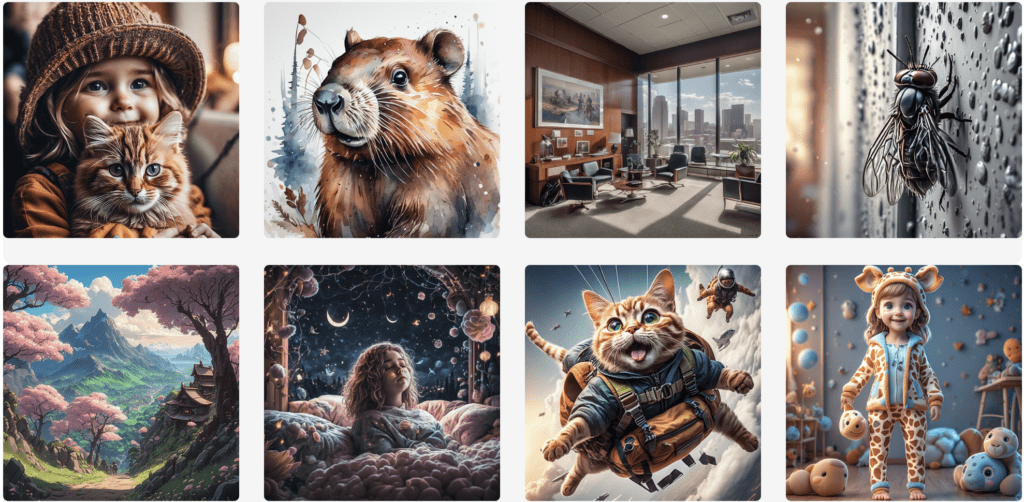
Step-aware Preference Optimization represents a leap forward in the post-training of diffusion models. Its innovative approach to aligning preference signals with the denoising performance at each step results in superior image generation outcomes. As the demand for high-quality, preference-aligned images continues to grow, SPO offers a promising solution that combines enhanced performance with remarkable training efficiency. This development sets a new standard in the field, paving the way for more advanced and user-aligned generative models in the future.