New AI Technique Promises Better Cross-Domain Generalization in Image Matching
- Foundation Model Guidance: OmniGlue uses a vision foundation model to improve feature matching across different image domains.
- Keypoint-Position Attention: A novel mechanism that disentangles spatial and appearance information, enhancing matching accuracy.
- Superior Performance: OmniGlue shows significant improvements over existing methods in diverse datasets, proving its versatility and robustness.
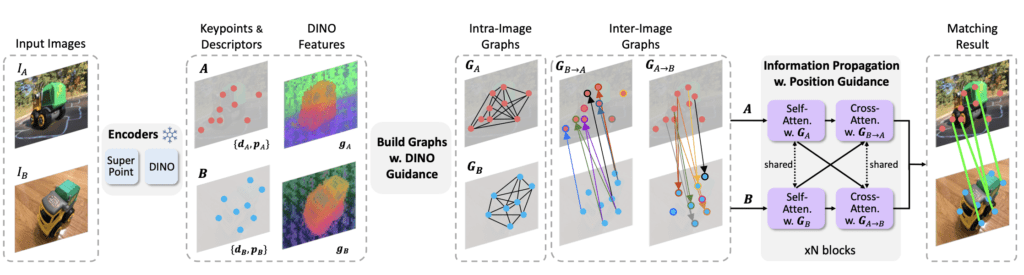
The field of image matching has seen numerous advancements with the development of novel feature matching techniques. However, many of these techniques struggle with generalization to new image domains not seen during training. Addressing this limitation, researchers have introduced OmniGlue, a revolutionary image matcher designed with generalization as a core principle. By leveraging a vision foundation model and a novel keypoint-position attention mechanism, OmniGlue sets a new standard in cross-domain feature matching.
Foundation Model Guidance
OmniGlue stands out by integrating the broad visual knowledge of a foundation model into its feature matching process. This model, trained on extensive datasets, provides generalizable correspondence cues that guide the inter-image feature propagation process. Unlike traditional methods that often specialize in specific image domains, OmniGlue’s foundation model offers a coarse but highly adaptable framework that enhances generalization across varied image types.
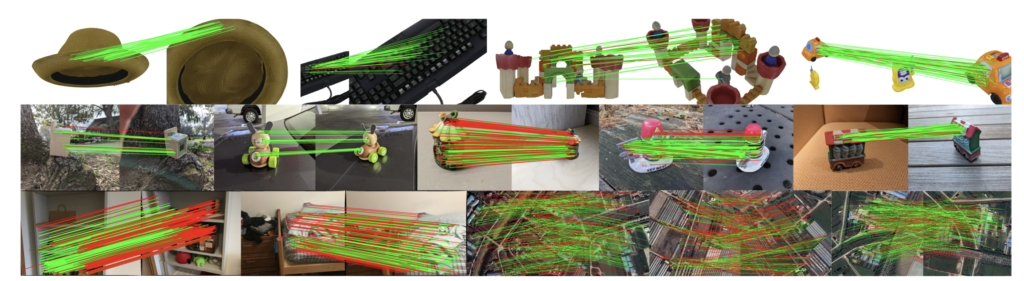
The foundation model’s guidance is particularly beneficial in scenarios involving unfamiliar image domains. By using this model, OmniGlue can effectively handle diverse datasets, including scene-level, object-centric, and aerial images, ensuring robust performance regardless of the image context.
Keypoint-Position Attention
A significant innovation within OmniGlue is its keypoint-position attention mechanism. This module disentangles spatial information from appearance features, preventing the model from becoming overly specialized in the training distribution of keypoints and relative pose transformations. By separating these elements, OmniGlue creates more flexible and accurate matching descriptors that adapt well to new and unseen domains.
This attention mechanism addresses a common issue in previous models where descriptor-position entanglement limited the model’s ability to generalize. OmniGlue’s approach ensures that spatial and appearance features are independently optimized, leading to enhanced matching accuracy and better overall performance.
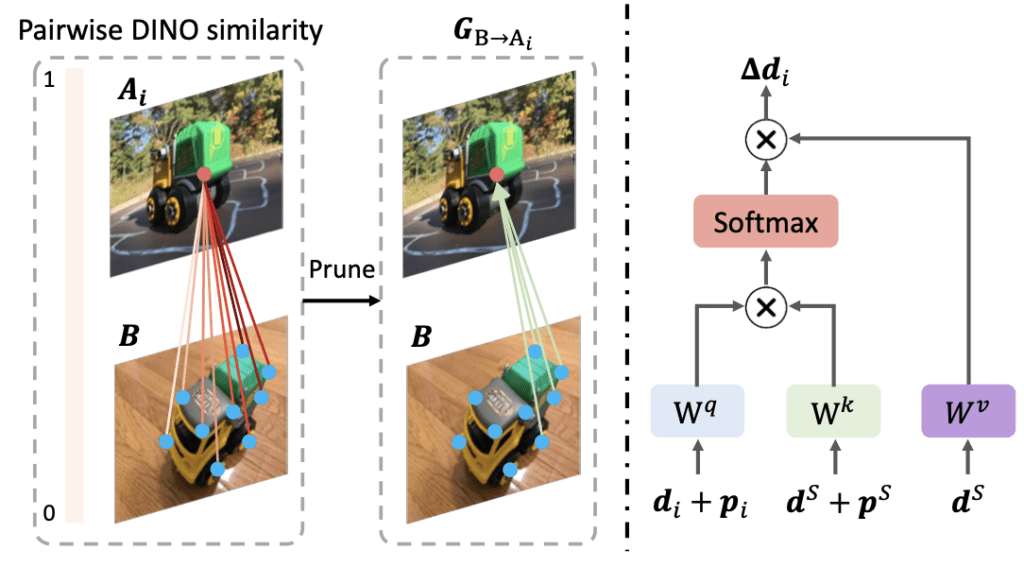
Superior Performance
OmniGlue’s effectiveness is demonstrated through comprehensive experiments on seven diverse datasets, showcasing its superior cross-domain generalization capabilities. The model achieves relative gains of 20.9% over directly comparable reference models and outperforms the recent LightGlue method by 9.5%. These results highlight OmniGlue’s potential to become a foundational tool in image matching applications.
One of the key advantages of OmniGlue is its adaptability. The model can be fine-tuned with a limited amount of data from a target domain, further improving its performance. This adaptability ensures that OmniGlue can be effectively applied in various real-world scenarios, from medical imaging to autonomous driving.
Future Directions
The development of OmniGlue opens several avenues for future research. Exploring how to leverage unannotated data in target domains could further enhance the model’s generalization capabilities. Additionally, refining the architectural design and data strategies could pave the way for even more robust and versatile image matching models.
OmniGlue represents a significant advancement in the field of image matching. By leveraging the broad visual knowledge of a foundation model and introducing a keypoint-position attention mechanism, OmniGlue achieves unprecedented cross-domain generalization. Its superior performance and adaptability make it a promising tool for a wide range of applications, setting a new standard for feature matching in computer vision. As the technology evolves, OmniGlue is poised to play a crucial role in advancing the capabilities of AI-driven image analysis.