The Rise of Open Source AI Threatens the Dominance of Tech Giants like Google and OpenAI
- The Open Source Threat: A leaked internal document from Google posits that the company and OpenAI are being outperformed by open source AI initiatives. This is due to the latter’s ability to innovate rapidly, cost-effectively, and in a more customized manner.
- The Power of LoRA: Low Rank Adaptation (LoRA), a technique that allows for efficient model fine-tuning, has played a significant role in the open source AI success, enabling rapid personalization of language models on consumer hardware.
- The Need for Speed and Flexibility: The document suggests that focusing on maintaining large models might not be as effective in the long run as iterating faster on smaller models. This is due to the fact that anyone with an idea can quickly generate and distribute updates for smaller models, leading to rapid cumulative improvements that can eventually surpass larger models.
The semi-analysis article focuses on a leaked Google document that suggests neither Google nor OpenAI is positioned to win the current AI “arms race”. Instead, the real contenders are open source initiatives that are outpacing the tech giants in terms of innovation, customization, and speed.
The open source community got its hands on a powerful foundation model when Meta’s LLaMA was leaked to the public. This led to an outpouring of innovation with new ideas coming from a broad spectrum of contributors, not just major research organizations. This has lowered the barrier to entry for AI development, with the ability to tinker with models being available to anyone with a beefy laptop and an evening to spare.
One of the critical factors enabling the rapid progress in open source AI has been the adoption of Low Rank Adaptation (LoRA). This technique allows model updates to be represented as low-rank factorizations, reducing the size of update matrices by a factor of several thousand. This has made it possible to fine-tune models at a fraction of the cost and time, and even personalize a language model in a few hours on consumer hardware. The document highlights that this technology is currently underexploited within Google, even though it could significantly impact their ambitious projects.
Moreover, the document mentions that retraining models from scratch is not as effective as fine-tuning existing ones. The open source world has shown that iterative improvements to a model can dominate, making a full retrain extremely costly. Hence, it’s essential to consider whether each new application or idea truly necessitates a whole new model.
Another significant insight is the potential inefficiency of maintaining large models. While Google and OpenAI have been focusing on these, the document suggests that in the long run, smaller models that can be iterated upon quickly could prove more effective. As almost anyone can generate and distribute updates for smaller models, it doesn’t take long before these rapid, cumulative improvements overtake larger models.
In the face of these developments, the Google document proposes a shift in strategy. It suggests that the company should prioritize learning from and collaborating with open source initiatives, and consider where its value add really lies. It also proposes a shift towards smaller model variants, given the rapid advancements possible in the sub-20 billion parameter regime.
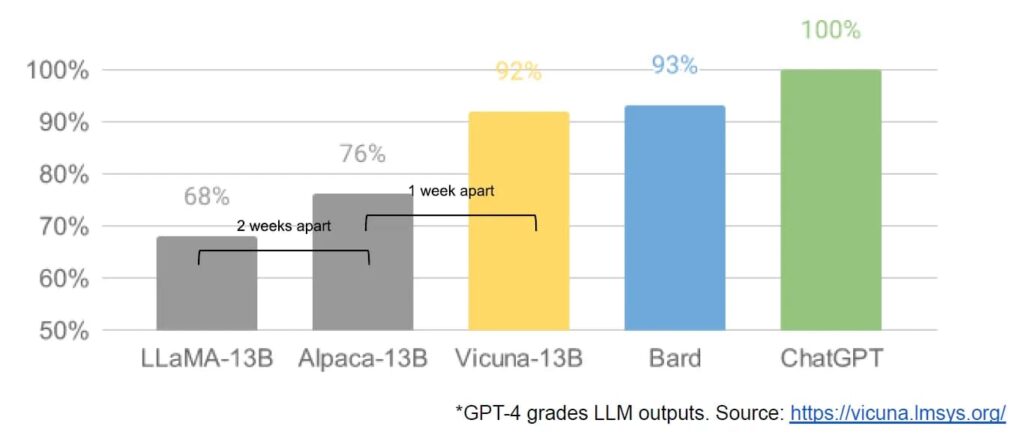
The leaked internal Google document you’re asking about was shared by an anonymous individual on a public Discord server and then republished by SemiAnalysis on May 4, 2023. The document was confirmed to originate from a researcher within Google, though it was stated that the views expressed do not necessarily represent the entirety of the firm.
According to the document, the author argues that both Google and OpenAI are not positioned to win the so-called “AI arms race.” The author believes that the real competition is coming from the open-source community, which has been making significant strides in developing AI technologies. A few examples of open-source advancements listed in the document include:
- Language Learning Models (LLMs) running on a phone, specifically mentioning the Google Pixel 6 running foundation models at 5 tokens/sec.
- Scalable Personal AI: You can fine-tune a personalized AI on your laptop in an evening.
- Responsible Release: The open-source community has made progress in creating models without restrictions.
- Multimodality: The state of the art multimodal ScienceQA was trained in an hour.
The document mentions that while Google’s models still hold a slight edge in terms of quality, open-source models are closing this gap rapidly. The open-source models are faster, more customizable, more private, and pound-for-pound more capable. The document highlights that the open-source community is accomplishing tasks with $100 and 13 billion parameters that Google and OpenAI struggle with at $10 million and 540 billion parameters. The author concludes that this development has profound implications for Google and OpenAI, suggesting a shift in strategy to learn from and collaborate with what others are doing outside Google, and to prioritize third-party integrations.
A significant turning point for the open-source community occurred in March, when Meta’s LLaMA, a capable foundation model, was leaked to the public. This led to a wave of innovation and rapid development, with the barrier to entry for training and experimentation dropping significantly. Now, training and experimentation have become accessible to more individuals with even a single person and a laptop capable of making meaningful contributions.
The author of the document also acknowledges that the rise of the open-source community should not have been surprising. The developments closely resemble the renaissance in image generation, with a lot of public involvement and the invention of new technologies. The author refers to this as the “Stable Diffusion moment” for LLMs, as it enabled a flurry of ideas and iteration from individuals and institutions around the world, quickly outpacing the large players.
The document also emphasizes the importance of LoRA (Low Rank Adaptation), a technique that allows model fine-tuning at a fraction of the cost and time, thus enabling a language model to be personalized on consumer hardware in just a few hours. This technique is seen as a game-changer and is said to be underexploited inside Google. The author also argues that retraining models from scratch is a hard path, as it throws away pretraining and any iterative improvements that have been made on top. Instead, the author suggests that new and better datasets and tasks can keep the model up to date without having to pay the cost of a full run.
The document asserts that large models are not necessarily more capable in the long run if small models can be iterated upon faster. It emphasizes the importance of the pace of improvement, which can be vastly higher for small models due to the low cost and short time required for producing LoRA updates.