Enhancing 3D Models with Structural Detail from Single-view Images
- Innovative Multiview Diffusion Technique: Uses diffusion models to create multiview images for accurate 3D reconstruction.
- Part-aware Segmentation: Incorporates a generalizable 2D segmentation model to predict and integrate part-based structures.
- Contrastive Learning for Consistency: Utilizes contrastive learning to manage multiview segmentation inconsistencies and ensure high-quality 3D models.
The quest for accurate 3D reconstruction from single-view images has taken a significant leap forward with the introduction of Part123. This novel framework addresses the limitations of traditional methods by incorporating part-aware segmentation, resulting in 3D models that are not only accurate but also structurally informative. Part123 leverages advanced diffusion models and a robust segmentation approach to generate high-quality 3D reconstructions that are essential for various downstream applications, including 3D shape editing and feature-preserving reconstruction.
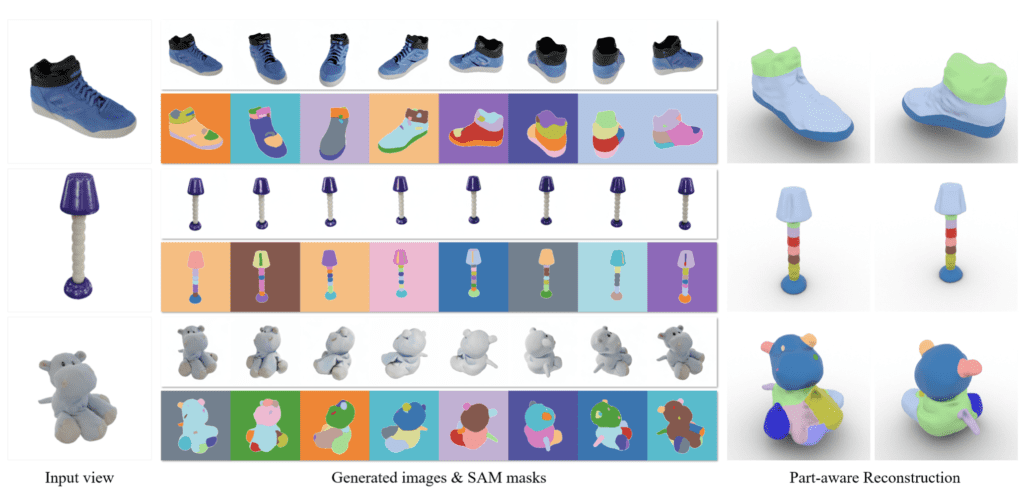
Innovative Multiview Diffusion Technique
Part123 begins with an innovative use of diffusion models to generate multiview-consistent images from a single input image. This technique ensures that the generated images align well across different viewpoints, laying a strong foundation for accurate 3D reconstruction. The multiview diffusion approach mitigates the common issues of noise and blurriness in reconstructed meshes, providing a clearer and more detailed representation of the object.
The diffusion models are particularly effective in capturing the intricate details of the object, which are crucial for the subsequent segmentation and reconstruction processes. By generating multiple consistent views, Part123 significantly enhances the quality and accuracy of the 3D model, making it more useful for practical applications.
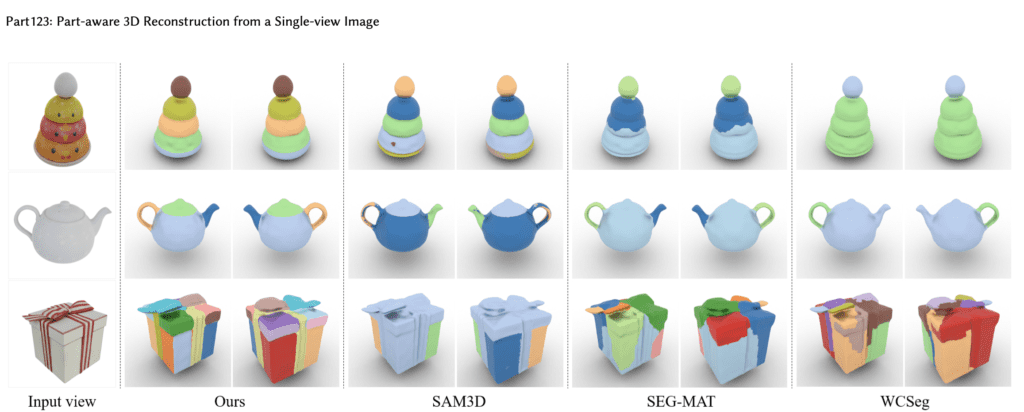
Part-aware Segmentation
One of the standout features of Part123 is its ability to incorporate part-aware segmentation into the 3D reconstruction process. Traditional methods often produce unstructured meshes that lack detailed part-based information, limiting their utility in applications requiring structural detail. Part123 overcomes this limitation by using the Segment Anything Model (SAM), a powerful 2D segmentation tool that generalizes well to various objects.
SAM generates multiview segmentation masks, which are then integrated into the 3D reconstruction process. This integration ensures that the final 3D model retains detailed part-based information, making it more suitable for tasks such as primitive fitting and 3D shape editing. The part-aware segmentation is particularly beneficial for creating models that are both visually accurate and structurally informative.
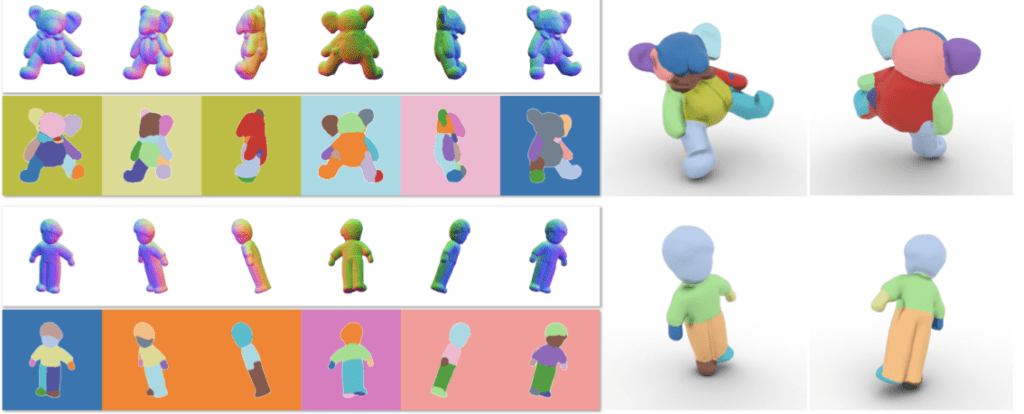
Contrastive Learning for Consistency
To handle the inconsistencies that arise from multiview segmentation, Part123 introduces contrastive learning into its neural rendering framework. Contrastive learning helps the model learn a part-aware feature space that maintains consistency across different views. This approach ensures that the reconstructed 3D models are coherent and free from artifacts commonly associated with multiview inconsistencies.
The use of contrastive learning is a significant advancement in 3D reconstruction, as it allows for the creation of high-quality models without the need for additional vision encoders or complex computer vision models. By embedding the part-based information directly into the feature space, Part123 achieves a level of accuracy and detail that sets it apart from existing methods.
However, there are areas for improvement. Currently, Part123 directly synthesizes 2D videos from given viewpoints, which can result in subtle artifacts. Future work may focus on incorporating explicit 4D representations to enhance the accuracy and quality of the models further. Additionally, improving the generation of fine details such as fingers and accessories remains a challenge that future research can address.
Part123 represents a significant advancement in the field of 3D reconstruction from single-view images. Its innovative use of multiview diffusion techniques, part-aware segmentation, and contrastive learning sets it apart from traditional methods. By providing a scalable and efficient solution for generating high-quality, part-aware 3D models, Part123 has the potential to revolutionize various applications in virtual reality, animation, and beyond. As research continues to refine and expand this technology, the possibilities for creating detailed and accurate 3D models will only grow.