Google DeepMind’s Novel Approach to Efficient AI Language Models
- Innovative Architecture: RecurrentGemma utilizes the Griffin architecture, which combines linear recurrences with local attention, optimizing memory usage and efficiency for processing long sequences.
- Performance and Efficiency: Despite fewer training tokens, RecurrentGemma matches the performance of its predecessor, Gemma-2B, while significantly improving throughput and reducing latency during inference.
- Potential for Resource-Constrained Applications: The model’s efficiency and smaller footprint make it ideal for deployment in environments where resources are limited, opening up new possibilities for the application of powerful language models.
Google DeepMind has introduced a groundbreaking language model, RecurrentGemma, which represents a significant shift from traditional transformer-based models to more efficient architectures suitable for real-world applications. This new model leverages Google’s innovative Griffin architecture, blending linear recurrences with local attention mechanisms, to enhance performance and reduce resource consumption.
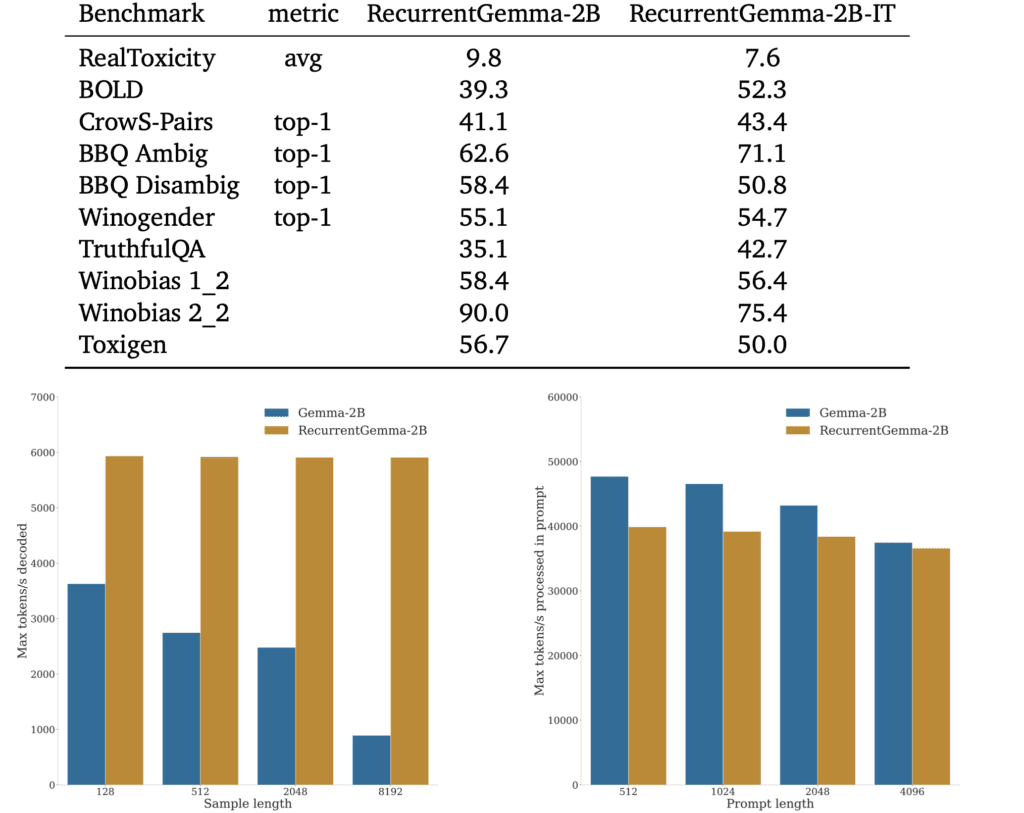
Technical Breakthroughs
RecurrentGemma stands out due to its fixed-sized state capability, which conserves memory and allows for efficient inference, particularly with long text sequences. This model architecture addresses some of the inherent limitations of transformers, particularly their extensive memory and computational demands. RecurrentGemma, with its 2 billion non-embedding parameters, has been meticulously pre-trained and instruction-tuned to ensure it delivers high-quality outputs that rival more extensively trained models like Gemma-2B.
Performance Comparisons and Enhancements
One of the key achievements of RecurrentGemma is its ability to maintain high performance levels while being trained on fewer tokens compared to its predecessors. This efficiency does not come at the cost of performance, as the model achieves throughput during inference that exceeds that of traditional transformer models, especially in handling longer sequences. Such improvements in throughput and reduced latency highlight the model’s potential to enhance user experiences by delivering faster and more accurate responses.
Applications in Constrained Environments
The reduced resource dependency of RecurrentGemma makes it particularly suitable for use in resource-constrained environments. This could lead to broader applications of AI, particularly in mobile devices, embedded systems, and other platforms where power and memory are limited. The ability of RecurrentGemma to operate efficiently without compromising on performance can significantly expand the reach and utility of language models across various industries, including healthcare, automotive, and telecommunications.
RecurrentGemma by Google DeepMind is poised to transform the landscape of language modeling with its efficient, high-performance architecture. As AI continues to integrate deeper into various sectors, RecurrentGemma’s approach offers a sustainable and scalable model that could drive future innovations in AI technology, making advanced language processing tools more accessible and practical for a wide range of applications.