How RedPajama datasets are redefining AI development with transparency, scalability, and versatility.
- Transparency in AI Training: RedPajama introduces an unprecedented level of openness in dataset composition, addressing the lack of clarity in current state-of-the-art LLMs.
- Massive Scale: With over 100 trillion tokens, RedPajama datasets provide one of the largest resources for pretraining language models.
- Customizable Versatility: Quality signals and metadata empower researchers to curate datasets tailored to specific needs, advancing open-source LLM innovation.
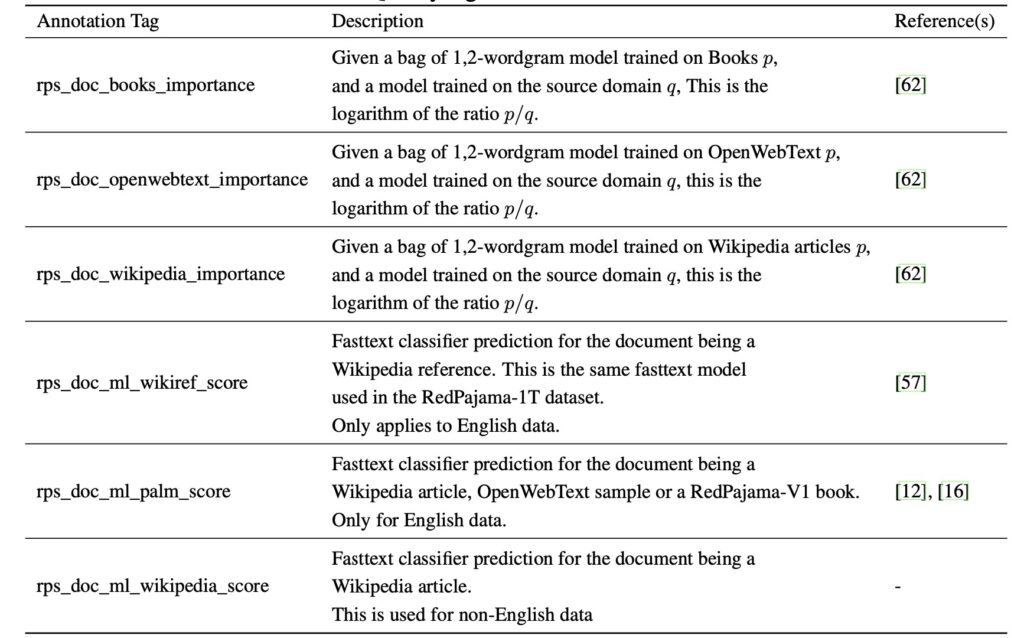
In the evolving world of artificial intelligence, the datasets used to train large language models (LLMs) are crucial yet often shrouded in mystery. Enter RedPajama, a groundbreaking initiative aiming to democratize access to high-quality, transparent, and scalable data for training LLMs. By releasing over 100 trillion tokens across two datasets—RedPajama-V1 and RedPajama-V2—this initiative provides a robust foundation for advancing open-source AI models.
Unlike many leading models that keep their data curation and filtering processes under wraps, RedPajama embraces transparency. Each dataset comes with detailed documentation, metadata, and quality signals, allowing developers to make informed decisions about how to curate and filter their datasets for optimal performance. This initiative seeks to empower researchers and application developers to better understand and design language models, paving the way for innovations in AI development.
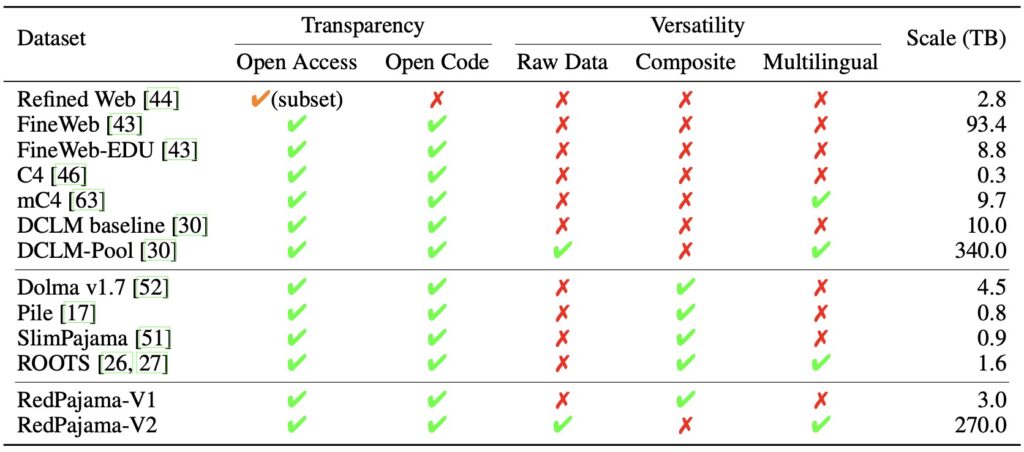
Scale Meets Versatility
The sheer scale of RedPajama datasets sets them apart. With over 100 trillion tokens of raw text data, these datasets rival the training data pools of the most powerful commercial LLMs. The datasets span diverse domains, ensuring versatility in their application. Each document is tagged with quality signals, enabling researchers to filter data based on their specific criteria, whether for general-purpose language models or specialized applications.
This scalability has already shown results. RedPajama has been instrumental in training prominent open-source models like Snowflake Arctic, Salesforce’s XGen, and AI2’s OLMo. These models demonstrate how curated subsets of RedPajama data can yield state-of-the-art performance, highlighting the value of its scale and versatility.
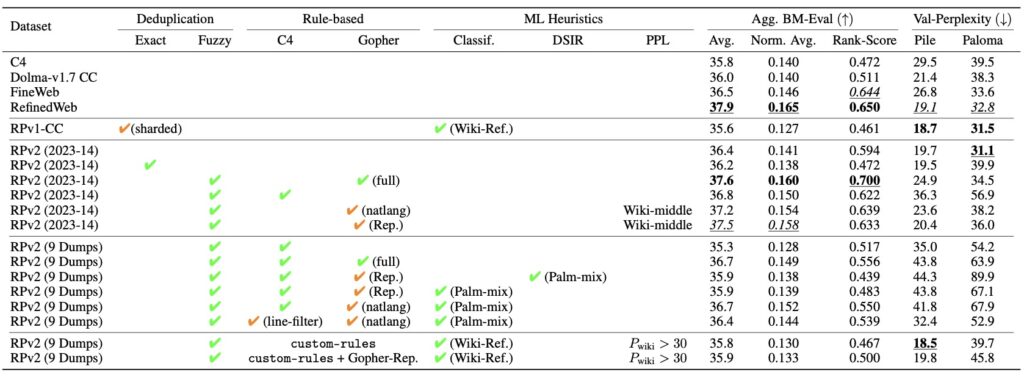
Advancing Open-Source AI
RedPajama’s impact extends beyond just being a dataset—it’s a framework for fostering innovation. By offering datasets in raw, unfiltered form but paired with quality signals, RedPajama invites researchers to experiment with new filtering techniques, curation strategies, and data compositions. This flexibility has already enabled ablation studies and performance analyses that showcase the advantages of well-filtered data on benchmark tasks.
Despite its successes, RedPajama acknowledges its limitations, such as the need for larger-scale exploration and decontamination analysis to address potential biases and privacy concerns. These challenges present opportunities for future work to build on the RedPajama foundation, refining its datasets and creating even more powerful language models.
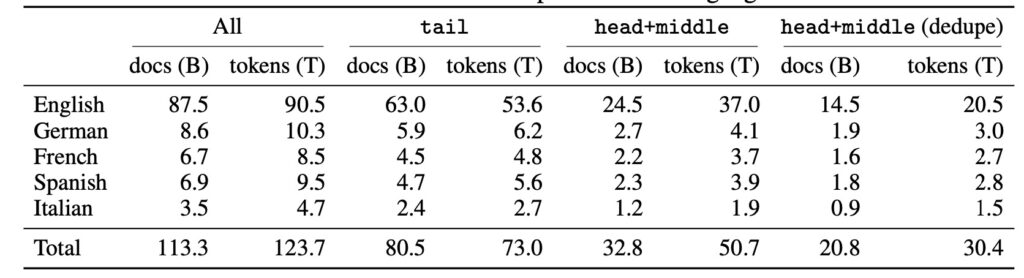
A New Standard for AI Development
RedPajama’s emphasis on transparency, scale, and versatility marks a significant step forward in AI research. By addressing the opaque nature of dataset curation in state-of-the-art LLMs, it sets a new standard for openness in the AI community. Researchers and developers now have a powerful tool to push the boundaries of what’s possible in AI, leveraging the RedPajama datasets to create high-performing, open-source language models.
As AI continues to influence science, technology, and society, RedPajama ensures that innovation remains accessible, equitable, and open to all.