A Paradigm Shift in AI Language Learning with Selective Language Modeling
- Introduction of Selective Language Modeling (SLM): Rho-1, Microsoft’s latest language model, uses a novel approach by focusing on key tokens during training, which are identified using a high-quality reference model.
- Significant Improvements in Learning Efficiency: This selective approach leads to substantial gains in model performance, demonstrating up to 30% improvement in few-shot learning accuracy on mathematical tasks.
- Potential for Large-Scale Application: While current validations are on smaller models, the inherent efficiency and scalability of SLM suggest potential benefits for larger models and broader datasets.

Microsoft‘s latest breakthrough in artificial intelligence comes with the introduction of Rho-1, a language model that revolutionizes the training process by employing Selective Language Modeling (SLM). This approach challenges the traditional method of uniformly applying a next-token prediction loss to all tokens during training. Instead, Rho-1 evaluates the utility of each token using a reference model and selectively trains only on those that are deemed beneficial, significantly optimizing both the training process and the use of computational resources.
Core Technology and Methodology
The core innovation of Rho-1 lies in its ability to differentiate between ‘useful’ and ‘non-beneficial’ tokens. This discrimination is based on initial analyses that reveal distinct loss patterns across tokens, suggesting that some contribute more to learning than others. By employing SLM, Rho-1 focuses its learning efforts on tokens with higher excess loss, which are more likely to enhance model performance. This method not only reduces wasted computation but also accelerates the learning process.
In practical terms, Rho-1 has shown remarkable improvements in performance. When pre-trained on the 15B OpenWebMath corpus, the model achieved an absolute increase of up to 30% in few-shot accuracy across nine math tasks. Additionally, after fine-tuning, Rho-1 models with 1B and 7B parameters produced top-tier results on the MATH dataset, achieving accuracies of 40.6% and 51.8%, respectively. These results were accomplished using only 3% of the pretraining tokens typically required, showcasing the efficiency of SLM.
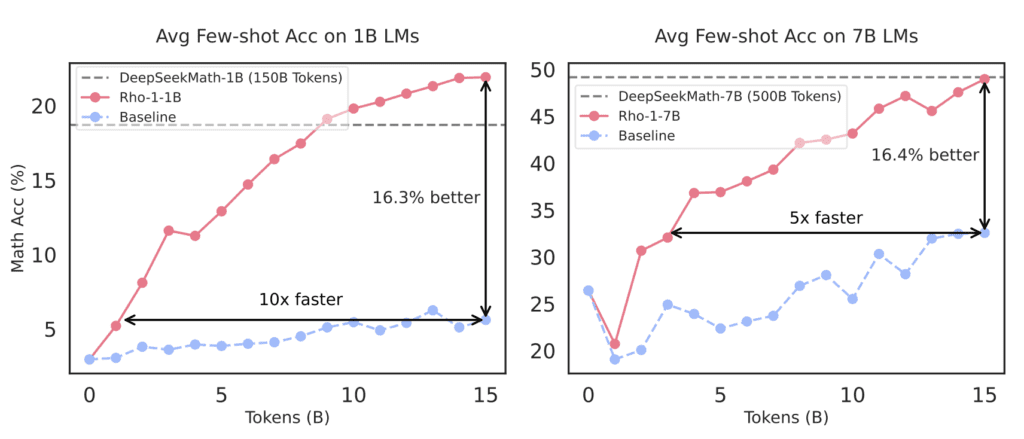
Broader Implications and Future Prospects
The implications of Rho-1’s selective training are vast. For one, it introduces a more sustainable model of AI development where efficiency is paramount. This is particularly crucial as the field of AI continues to grapple with the environmental and economic costs associated with training large models.
Moreover, the scalability of Rho-1’s methodology suggests that it could be applied to much larger models and datasets, potentially reducing the time and resources required for training without compromising on performance. Future research will explore extending this technique to larger scales and possibly integrating it with other AI training strategies, such as reinforcement learning or curriculum learning, to further enhance its effectiveness.
Rho-1 represents a significant advancement in the field of AI and machine learning. By prioritizing which tokens to train on, Microsoft not only improves the efficiency of its language models but also sets a new standard for the industry in terms of how AI training might be approached in the future. This model not only promises better performance but also aligns with a broader move towards more sustainable and cost-effective AI development.