How Open Post-Training Recipes and RLVR Framework Redefine Large-Scale Model Performance
- Tülu 3 405B demonstrates the scalability of open post-training recipes, achieving competitive or superior performance to DeepSeek V3 and GPT-4o, while outperforming other open-weight models like Llama 3.1 405B Instruct and Nous Hermes 3 405B.
- The Reinforcement Learning from Verifiable Rewards (RLVR) framework significantly enhances MATH performance at larger scales, showcasing the potential of specialized training for complex tasks.
- Scaling to 405B parameters required overcoming significant technical challenges, including compute requirements and hyperparameter tuning, but the results validate the robustness of the Tülu 3 training pipeline.
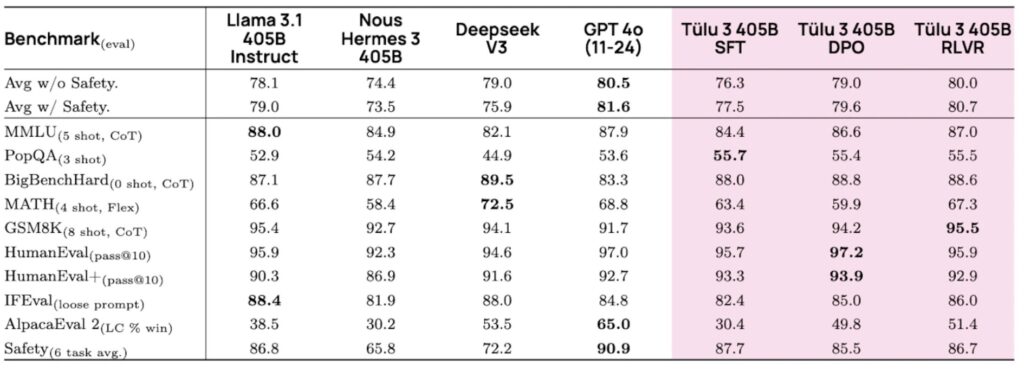
The release of Tülu 3 405B marks a monumental leap in the evolution of large-scale language models. Building on the success of the Tülu 3 release in November, this latest iteration applies fully open post-training recipes to the largest open-weight models, setting a new benchmark for performance and scalability. By leveraging a novel Reinforcement Learning from Verifiable Rewards (RLVR) framework and meticulous data curation, Tülu 3 405B not only surpasses its predecessors but also outperforms industry giants like DeepSeek V3 and GPT-4o on multiple benchmarks.
The Tülu 3 Recipe: A Blueprint for Success
The Tülu 3 post-training recipe is a carefully crafted methodology designed to enhance model performance through a combination of supervised fine-tuning, preference optimization, and RLVR. For the 405B model, the recipe was scaled up while maintaining the core principles that made the 8B and 70B models successful:
- Data Curation and Synthesis: Targeting core skills through high-quality, diverse datasets.
- Supervised Fine-Tuning (SFT): Training on a curated mix of prompts and completions to refine model behavior.
- Direct Preference Optimization (DPO): Balancing off-policy and on-policy preference data to align the model with human preferences.
- RLVR Framework: Enhancing specific skills, such as mathematical problem-solving, through verifiable rewards.
This structured approach ensures that the model not only performs well on general tasks but also excels in specialized domains like mathematics and instruction following.
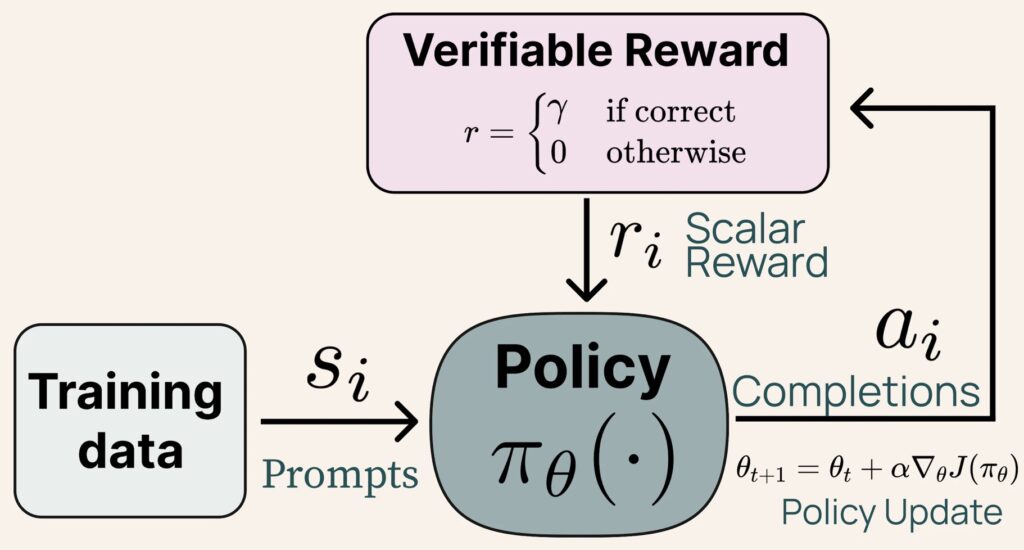
RLVR: The Secret Sauce for Scaling
The RLVR framework is a game-changer for large-scale models. By focusing on tasks with verifiable outcomes, such as solving math problems, RLVR allows the model to learn more effectively from its mistakes and successes. At the 405B scale, RLVR demonstrated even greater efficacy, particularly in improving MATH performance. This contrasts with smaller models, which benefit more from diverse datasets like GSM8k and IFEval.
To implement RLVR at this scale, the team deployed the model using vLLM with 16-way tensor parallelism, while utilizing 240 GPUs for training. Each RLVR iteration involved inference, weight transfer, and training, with the entire process optimized to reduce computational costs. The use of an 8B value model further streamlined the process, though future work could explore larger value models or alternative RL algorithms like GRPO.
Technical Challenges and Triumphs
Scaling to 405B parameters was no small feat. The compute requirements alone were staggering, with 32 nodes (256 GPUs) running in parallel for training and inference. The team also faced challenges like NCCL timeout and synchronization issues, which required constant monitoring and intervention.
Hyperparameter tuning was another hurdle. Given the computational costs, the team adhered to the principle of “lower learning rates for larger models,” a strategy consistent with prior work on Llama models. Despite these challenges, the training pipeline proved robust, enabling the successful release of the largest model trained with a fully open recipe to date.
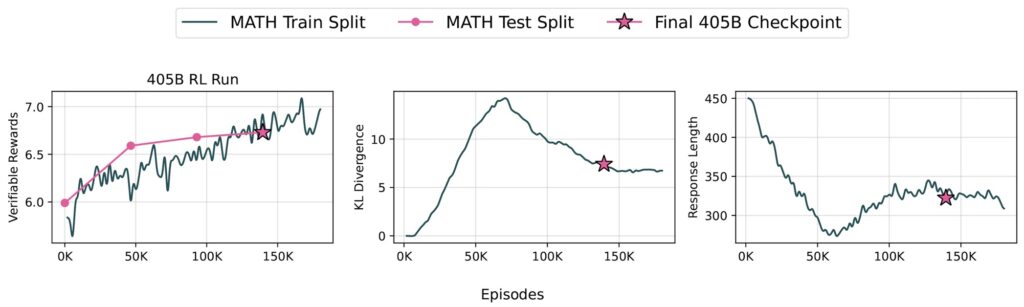
Tülu 3 405B vs. the Competition
Tülu 3 405B’s performance speaks for itself. On standard benchmarks, it consistently outperforms prior open-weight models like Llama 3.1 405B Instruct and Nous Hermes 3 405B. It also holds its own against DeepSeek V3 and GPT-4o, particularly in safety benchmarks.
One of the most exciting findings is the scalability of RLVR. The framework’s impact on MATH performance was more pronounced at the 405B scale, suggesting that larger models are better suited for complex, specialized tasks. This aligns with findings from the DeepSeek-R1 report and underscores the potential of RLVR for future model development.
The Future of Open Post-Training Recipes
The success of Tülu 3 405B is a testament to the power of open post-training recipes and the RLVR framework. By making these methods fully open, the team has not only advanced the field but also set the stage for further innovation. Future work could explore larger value models, alternative RL algorithms, or even more specialized datasets to push the boundaries of what large-scale models can achieve.
As the AI community continues to grapple with the challenges of scaling and performance, Tülu 3 405B stands as a shining example of what’s possible when cutting-edge research meets meticulous execution. The journey to surpass DeepSeek V3 and GPT-4o is far from over, but with Tülu 3 405B, the future looks brighter than ever.