New Method Outperforms Baseline Approaches with Rapid 3D Mesh Creation and Precise Pose Estimation from Minimal Images
- Innovative 3D Reconstruction:Â SpaRP introduces a cutting-edge approach for generating detailed 3D models and camera poses from a few unposed 2D images, addressing challenges in traditional methods that require dense and overlapping views.
- Efficiency and Precision:Â The method leverages advanced 2D diffusion models to provide high-quality 3D reconstructions and accurate pose estimations in just 20 seconds, significantly enhancing performance compared to existing techniques.
- Versatile Applications: SpaRP’s capability to handle sparse views with minimal data makes it ideal for practical scenarios like e-commerce and consumer-grade 3D capture, expanding its utility across various domains.
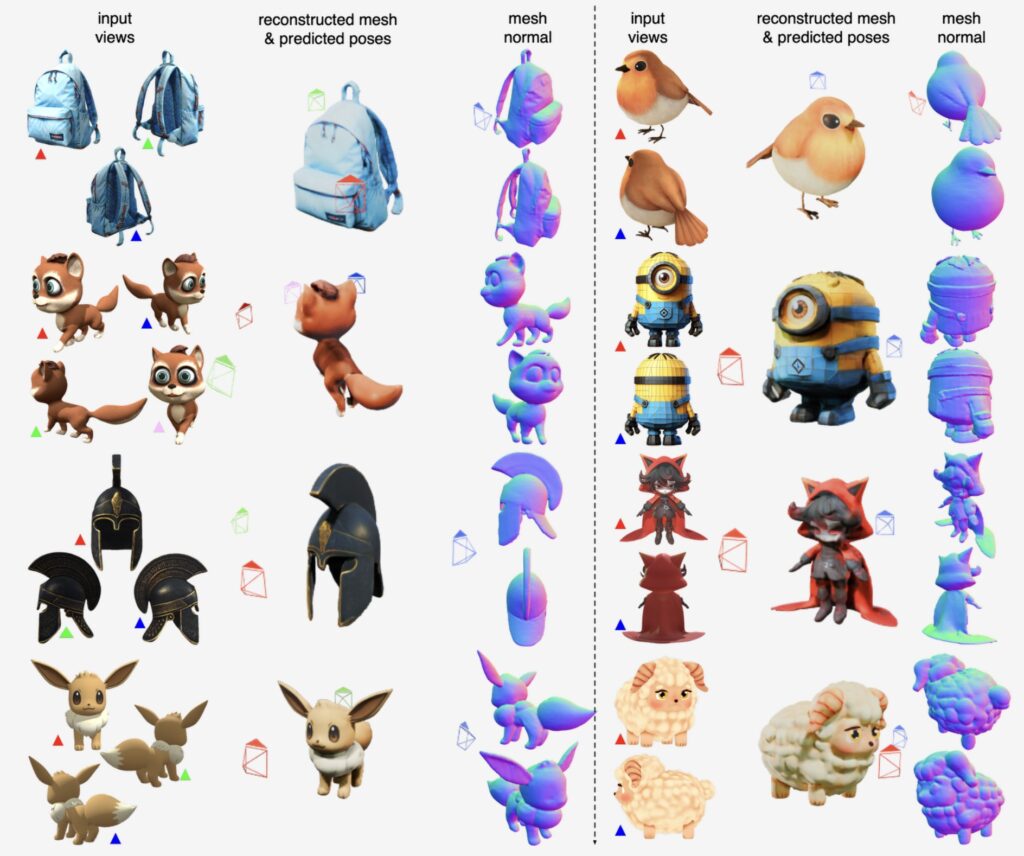
The quest for accurate 3D object reconstruction has long been a cornerstone of advancements in fields ranging from virtual reality to robotics. While traditional methods have made strides in creating high-fidelity 3D models, they typically rely on a dense array of images and precise camera data. The emergence of SpaRP, a novel technique for fast and efficient 3D object reconstruction and pose estimation, promises to revolutionize how we approach this complex problem, particularly in scenarios where such dense data is impractical.
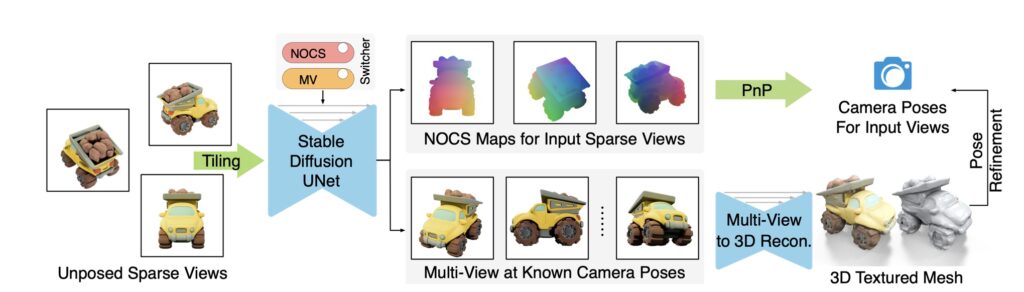
SpaRP (Sparse-view Reconstruction and Pose estimation) represents a significant leap forward by tackling the challenges associated with reconstructing 3D objects from minimal, unposed 2D images. Unlike earlier methods that required extensive overlapping views or involved intricate per-shape optimizations, SpaRP uses advanced 2D diffusion models to synthesize 3D spatial relationships from sparse input. This approach allows SpaRP to deliver a highly accurate textured 3D mesh and precise camera poses with remarkable speed.
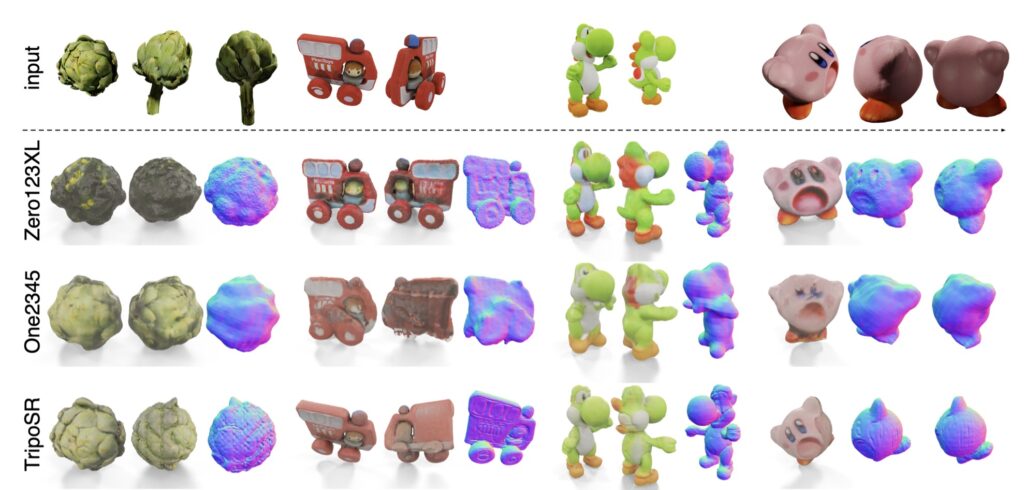
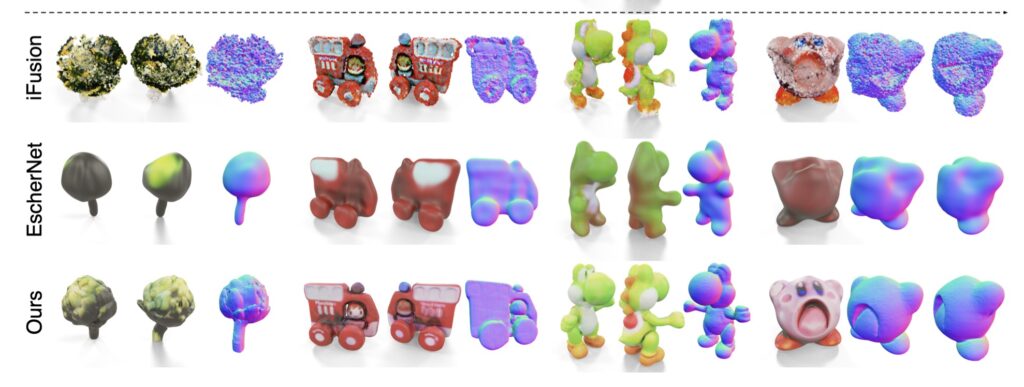
The core innovation of SpaRP lies in its ability to distill knowledge from 2D diffusion models, which are traditionally used for generating detailed textures and geometry from single images. By fine-tuning these models to predict not only the 3D geometry but also the relative camera poses from sparse views, SpaRP integrates this information to produce coherent and high-quality 3D reconstructions. The result is a system capable of generating accurate models in about 20 seconds—a feat that stands in stark contrast to the longer processing times of previous methods.
Extensive experiments on multiple datasets have shown that SpaRP significantly outperforms baseline approaches in both 3D reconstruction quality and pose prediction accuracy. This efficiency makes SpaRP particularly valuable for real-world applications where acquiring a full set of high-resolution images is challenging. For instance, in e-commerce or consumer-grade 3D scanning, where users might only have a few images of a product, SpaRP’s rapid processing and precise output can offer a practical solution.
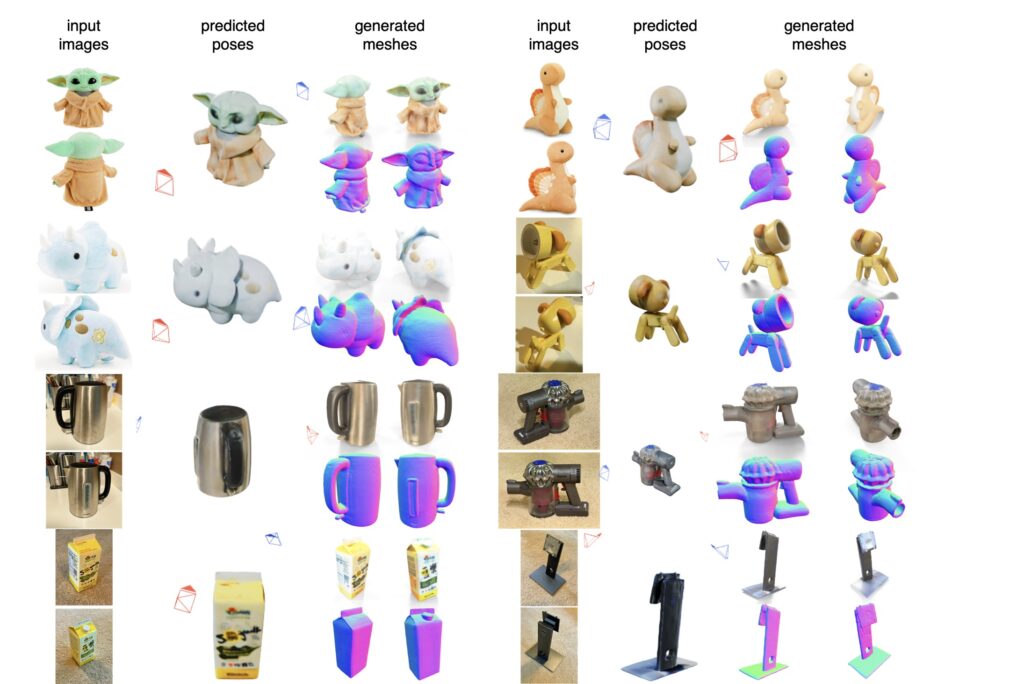
Moreover, SpaRP addresses a common limitation of current methods: the generation of ambiguous or hallucinated regions in areas not visible from the input images. By leveraging its advanced diffusion model capabilities, SpaRP minimizes these ambiguities, providing users with more predictable and controllable 3D outputs. This enhanced control over the reconstruction process is a critical advancement for applications that demand high accuracy and reliability.
SpaRP marks a pivotal advancement in the field of 3D object reconstruction and pose estimation. Its innovative use of 2D diffusion models, coupled with its efficiency and precision, sets a new standard for handling sparse-view inputs. As SpaRP continues to evolve, it holds promise for transforming a wide range of applications, making high-quality 3D reconstruction more accessible and practical than ever before.