Transforming Instruction-Based Editing Through Rectified Guidance and Contrastive Learning
- SuperEdit introduces a groundbreaking approach to instruction-based image editing by rectifying editing instructions and aligning them more accurately with original-edited image pairs, addressing the core issue of noisy supervision signals.
- By leveraging contrastive supervision with positive and negative instructions, alongside a unified guideline based on diffusion priors, SuperEdit enhances model training effectiveness without relying on complex vision-language models (VLMs) or extensive pre-training.
- Demonstrating remarkable results, SuperEdit outperforms existing state-of-the-art methods like SmartEdit by 9.19% on the Real-Edit benchmark, using 30 times less training data and a model 13 times smaller in size.
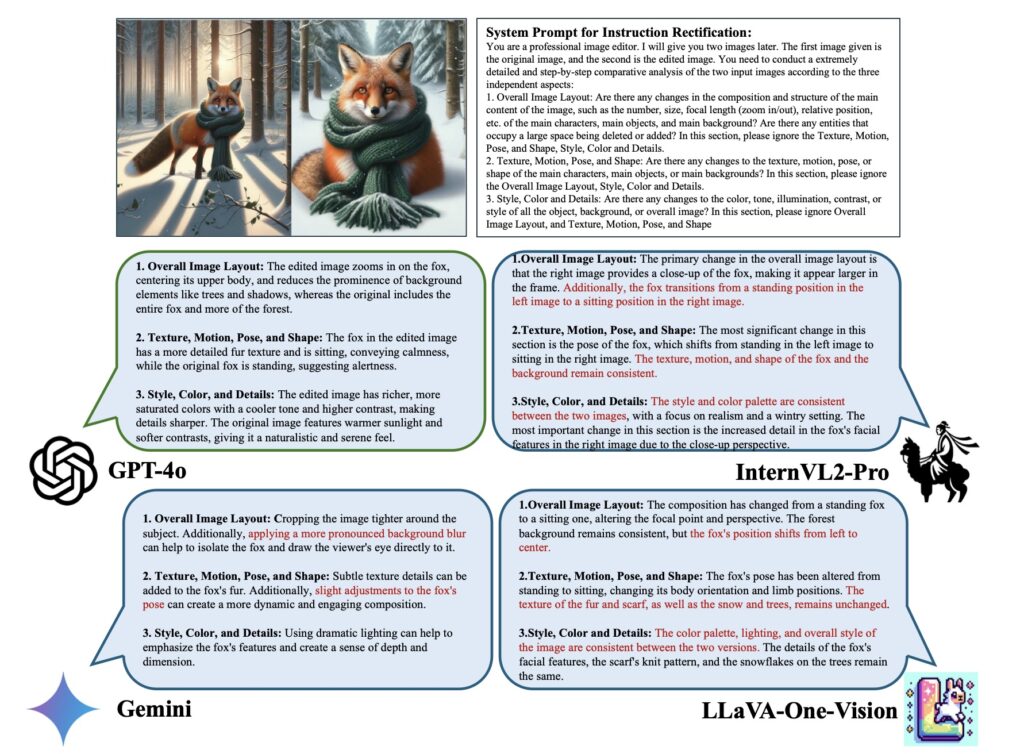
Instruction-based image editing has long been a challenging frontier in computer vision, primarily due to the difficulty of obtaining accurate, high-quality editing data. Traditional datasets, often constructed through automated methods, suffer from noisy supervision signals caused by mismatches between editing instructions and the corresponding original-edited image pairs. This fundamental issue has hindered the performance of editing models, even as recent efforts have focused on generating better images, incorporating massive vision-language models (VLMs), or relying on pre-training tasks for recognition. However, these approaches have largely sidestepped the root problem of supervision quality. Enter SuperEdit, a novel solution that redefines the landscape of image editing by prioritizing the enhancement of supervision signals through rectified instructions and contrastive learning, offering a simpler yet highly effective alternative.
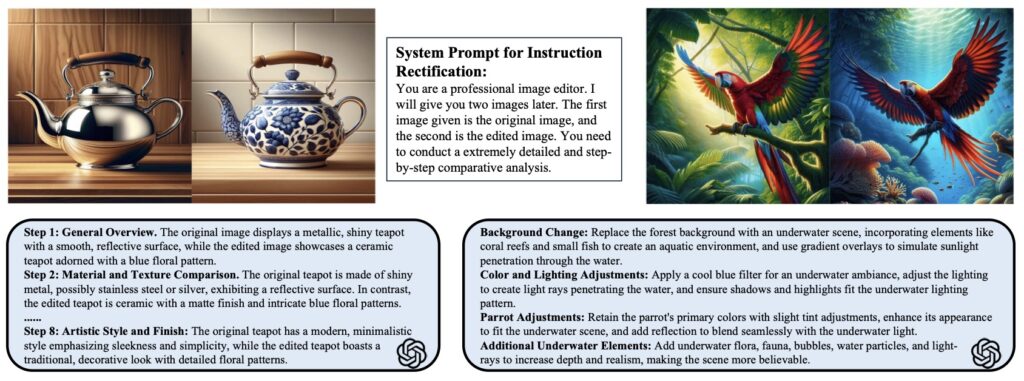
At the heart of SuperEdit lies the recognition that editing instructions must be closely aligned with the image pairs they describe to provide meaningful guidance to models. Many existing datasets fail in this regard, as automated methods for data collection often result in instructions that poorly reflect the actual edits made to an image. SuperEdit tackles this by introducing a unified guideline for rectifying editing instructions, drawing on specific generation attributes of editing models—known as diffusion priors—that manifest at different inference steps, independent of the text input. By using these priors, SuperEdit ensures that vision-language models, when employed, can refine instructions to better match the visual transformations in the image pairs. This rectification process is a game-changer, as it directly addresses the mismatch issue without requiring the cumbersome integration of VLMs into the core architecture or additional pre-training tasks, streamlining the path to improved supervision.
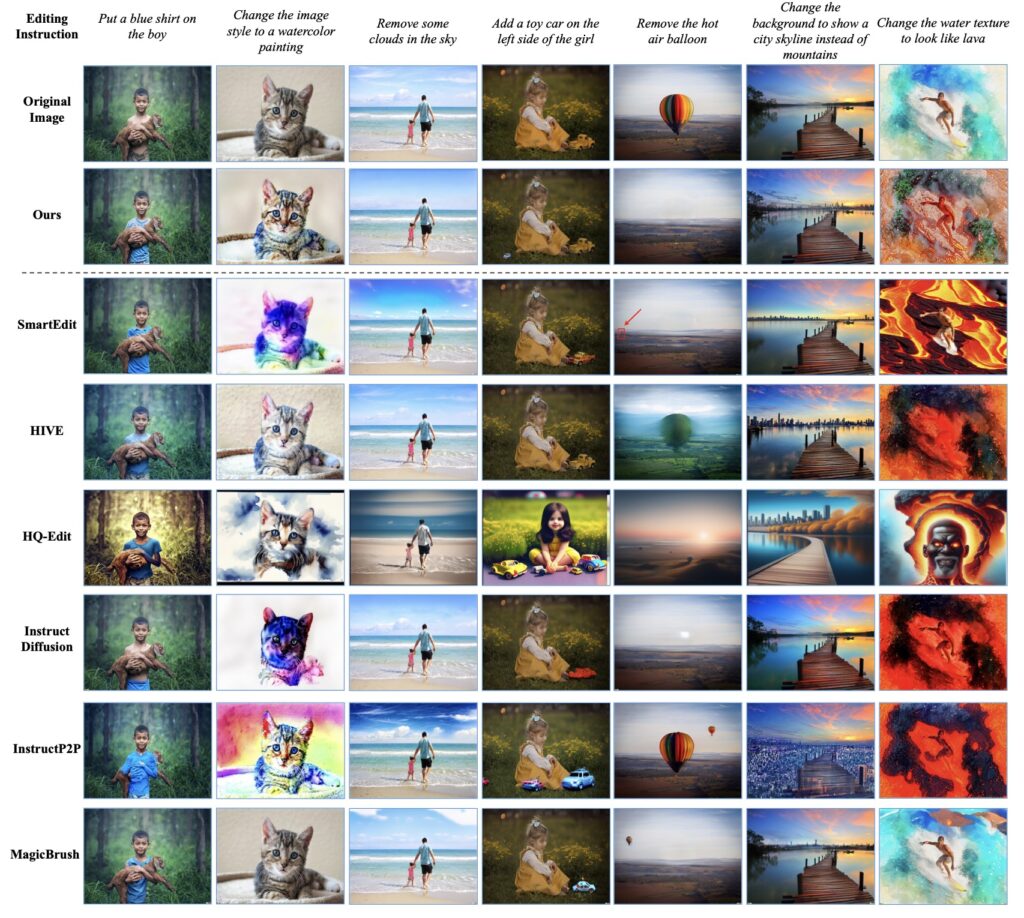
However, rectification alone cannot resolve every challenge in image editing, particularly in complex scenarios where instructions remain ambiguous or insufficient. To overcome this, SuperEdit goes a step further by constructing contrastive supervision signals. This involves creating both positive and negative editing instructions for a given image pair and incorporating them into model training using triplet loss. Positive instructions guide the model toward the desired edit, while negative instructions teach it what to avoid, sharpening its ability to discern and execute precise edits. This dual-signal approach enhances the model’s learning process, enabling it to handle a wider range of editing tasks with greater accuracy. Unlike previous methods that scale up datasets with noisy data or rely on architectural complexity, SuperEdit’s focus on contrastive learning offers a data-oriented solution that maximizes supervision effectiveness with minimal structural overhead.
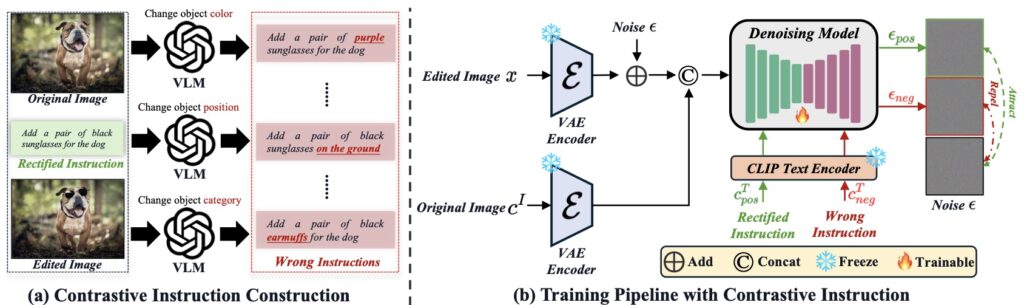
The results of SuperEdit speak for themselves. On multiple benchmarks, including the rigorous Real-Edit dataset, this method has demonstrated significant improvements over existing state-of-the-art approaches like SmartEdit. Specifically, SuperEdit achieves a 9.19% performance boost on Real-Edit while using 30 times less training data and a model that is 13 times smaller in size. This efficiency is a testament to the power of high-quality supervision signals, proving that substantial gains can be made without resorting to massive datasets or intricate architectures. Furthermore, evaluations under both GPT-4o and human scrutiny confirm that SuperEdit consistently outperforms competitors, highlighting its robustness and practical applicability in real-world editing tasks.
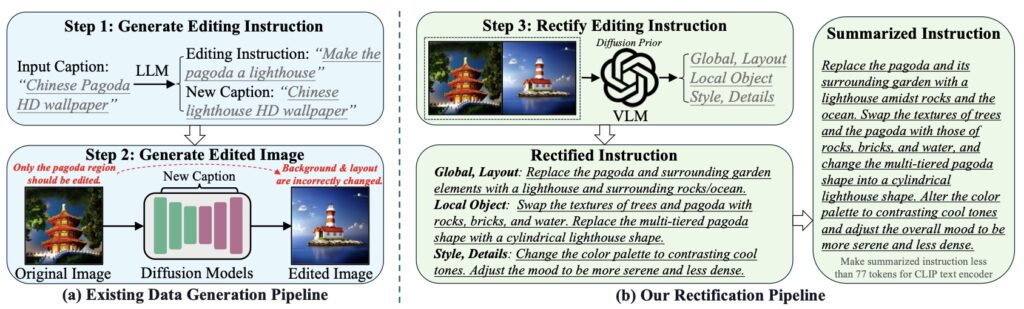
What sets SuperEdit apart is its broader perspective on the field of image editing. Rather than chasing incremental improvements through larger models or more data, it poses a critical research question: What level of performance can be achieved by focusing primarily on supervision quality and optimization, with minimal architectural modifications? The answer is striking. SuperEdit shows that high-quality supervision can effectively compensate for architectural simplicity, challenging the prevailing trend of scaling up resources and complexity. This insight opens new avenues for research, suggesting that the future of image editing may lie not in bigger models, but in smarter, more precise guidance mechanisms.
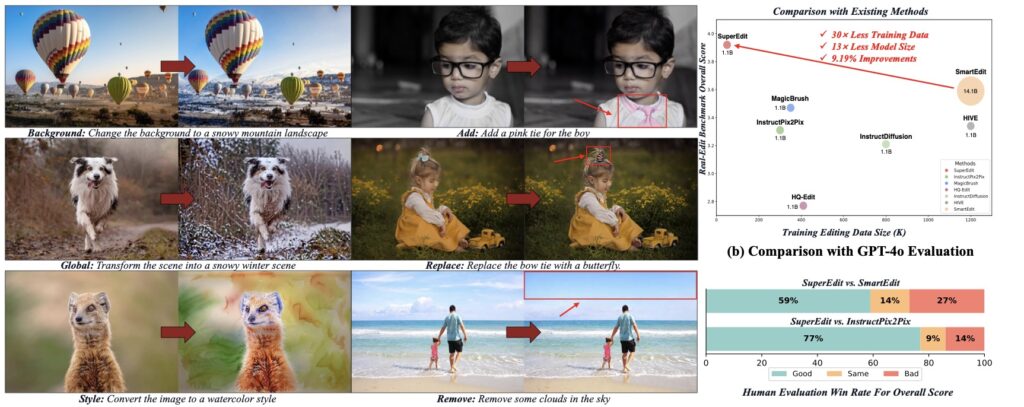
In a landscape where many efforts have been directed toward scaling edited images with noisy supervision, integrating massive VLMs, or performing additional pre-training, SuperEdit stands out by addressing the fundamental issue head-on. It offers a direct, efficient, and elegant solution that prioritizes the quality of supervision over the quantity of resources. By rectifying editing instructions and leveraging contrastive learning, SuperEdit not only achieves superior performance but also redefines how we think about instruction-based image editing. As the field continues to evolve, this approach serves as a powerful reminder that sometimes, the most transformative innovations come from revisiting and refining the basics. SuperEdit is not just a tool; it’s a paradigm shift, paving the way for more accessible, effective, and intelligent image editing solutions.
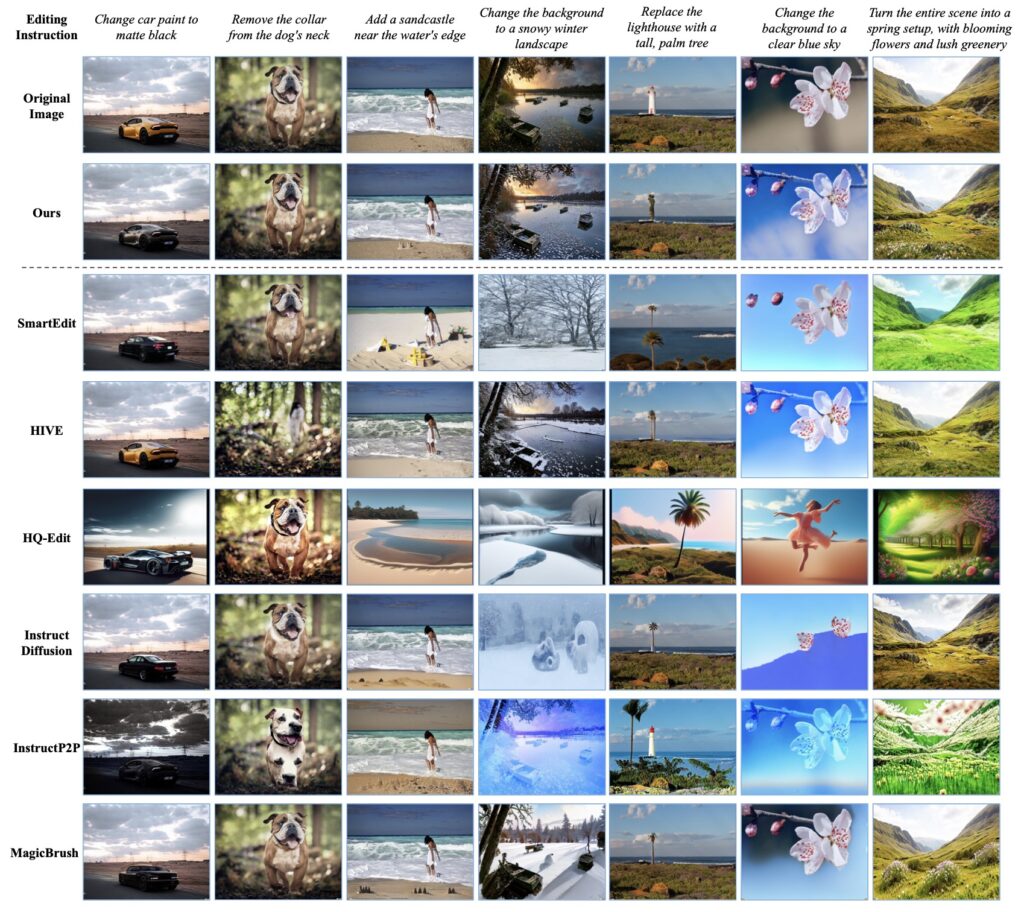