Bridging Speech and Motion for Naturalistic Digital Avatars
- Full-Body Control: Unlike traditional models that focus solely on upper body gestures, SynTalker enables nuanced control of full-body motions based on both speech and user-defined text prompts.
- Addressing Data Limitations: By leveraging existing text-to-motion datasets, SynTalker overcomes the challenge of limited full-body motion data, ensuring a wider range of human activities can be accurately represented.
- Advanced Technical Framework: Utilizing a multi-stage training process and diffusion-based conditional inference, SynTalker achieves unprecedented precision in generating realistic motions that align seamlessly with speech.
In the evolving landscape of artificial intelligence, the ability to create lifelike digital avatars has become increasingly important, particularly in applications like virtual assistants, gaming, and interactive environments. Traditional co-speech motion generation techniques have focused primarily on upper body gestures, leaving a significant gap in the ability to produce comprehensive, full-body motions that reflect the nuances of human interaction. SynTalker seeks to bridge this gap by allowing digital avatars to perform a range of movements, such as “talking while walking,” effectively mimicking real-life conversations.
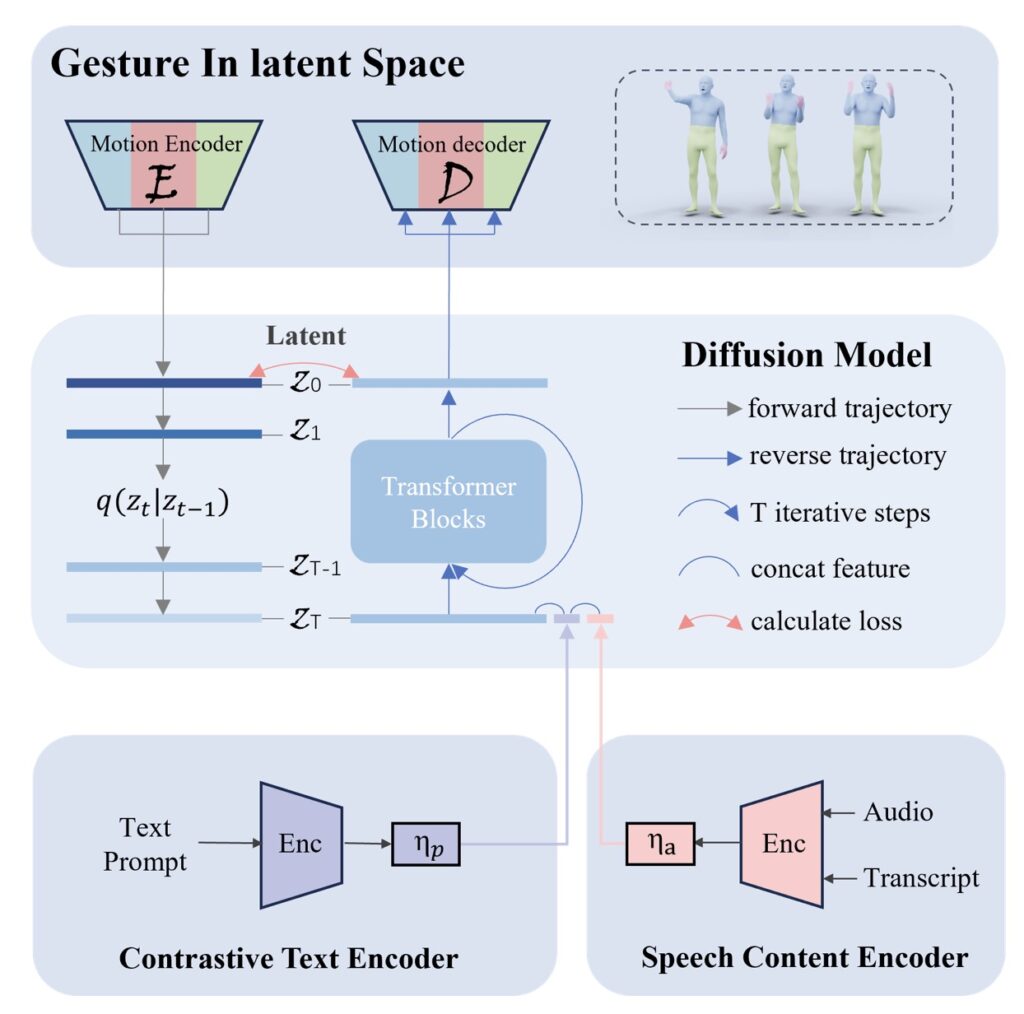
One of the main challenges in co-speech motion generation is the limited availability of datasets that capture the variety of full-body motions. Existing speech-to-motion datasets often include only basic gestures, leading to a lack of training data for more complex actions. SynTalker addresses this by integrating off-the-shelf text-to-motion datasets, thereby enhancing the model’s understanding of a broader range of human activities. This approach allows for more realistic and engaging representations of avatars, expanding their usability in diverse applications.
The technical foundation of SynTalker is equally impressive. The model employs a multi-stage training process that synchronizes the comprehension of co-speech audio signals with textual prompts. This allows the system to interpret both speech and text effectively, facilitating the generation of sophisticated full-body motions that align with the emotional and contextual nuances of the dialogue. Furthermore, the introduction of a separate-then-combine strategy during inference enables fine-grained control over individual body parts, providing users with the flexibility to direct specific motions based on context.
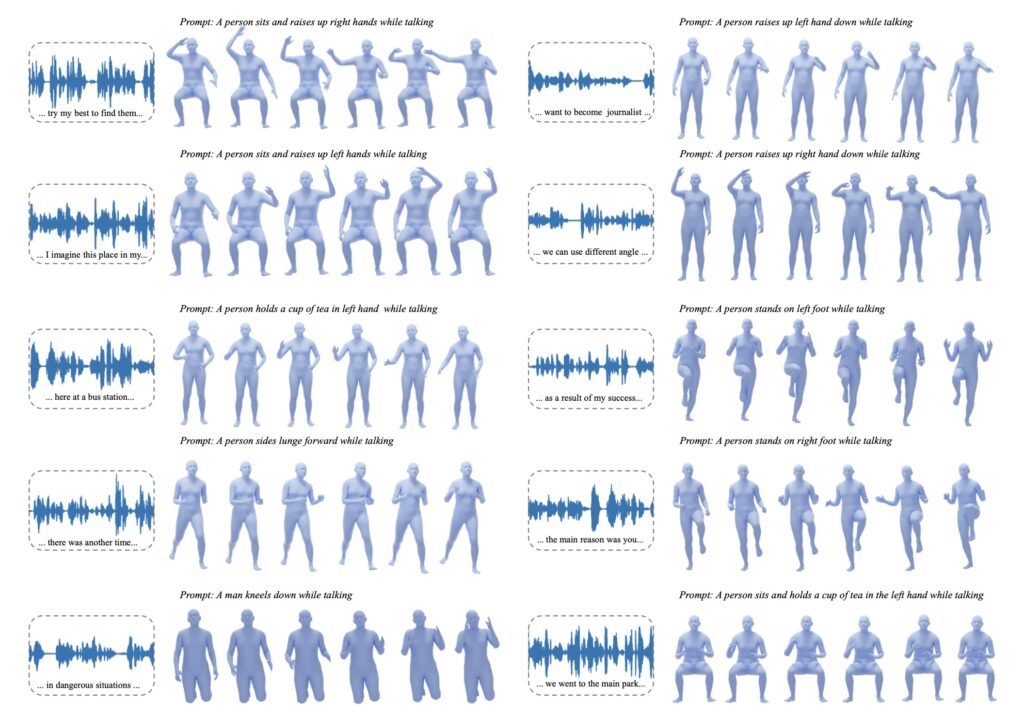
Extensive experiments have demonstrated SynTalker’s capability to produce highly accurate and synchronized full-body movements in response to both speech and prompts. This marks a significant leap forward from existing co-speech generation methods, which often lack the precision and flexibility required for natural interactions. By harnessing the power of AI, SynTalker not only enhances the realism of digital avatars but also enriches the user experience across various platforms, from virtual meetings to interactive gaming environments.
SynTalker represents a pivotal advancement in the field of co-speech motion generation. By overcoming limitations in existing datasets and leveraging innovative technical approaches, this model enables the creation of fully expressive digital avatars that can respond dynamically to human speech and contextual cues. As the demand for immersive digital experiences continues to grow, technologies like SynTalker will play a crucial role in shaping the future of human-computer interaction, making digital communication more natural and engaging than ever before.