Introducing the First Comprehensive Benchmark for Complex Video Generation from Text Prompts
- T2V-CompBench offers the first benchmark tailored for compositional text-to-video generation.
- The benchmark includes diverse evaluation metrics across seven categories with 700 text prompts.
- Initial findings highlight the challenges current models face in achieving high-quality compositional text-to-video generation.
Text-to-video (T2V) generation technology has progressed rapidly, enabling the creation of videos from textual descriptions. However, a critical aspect that remains underexplored is the ability to compose various objects, attributes, actions, and motions into coherent and dynamic video sequences. Addressing this gap, T2V-CompBench has been introduced as the first comprehensive benchmark specifically designed for compositional text-to-video generation.
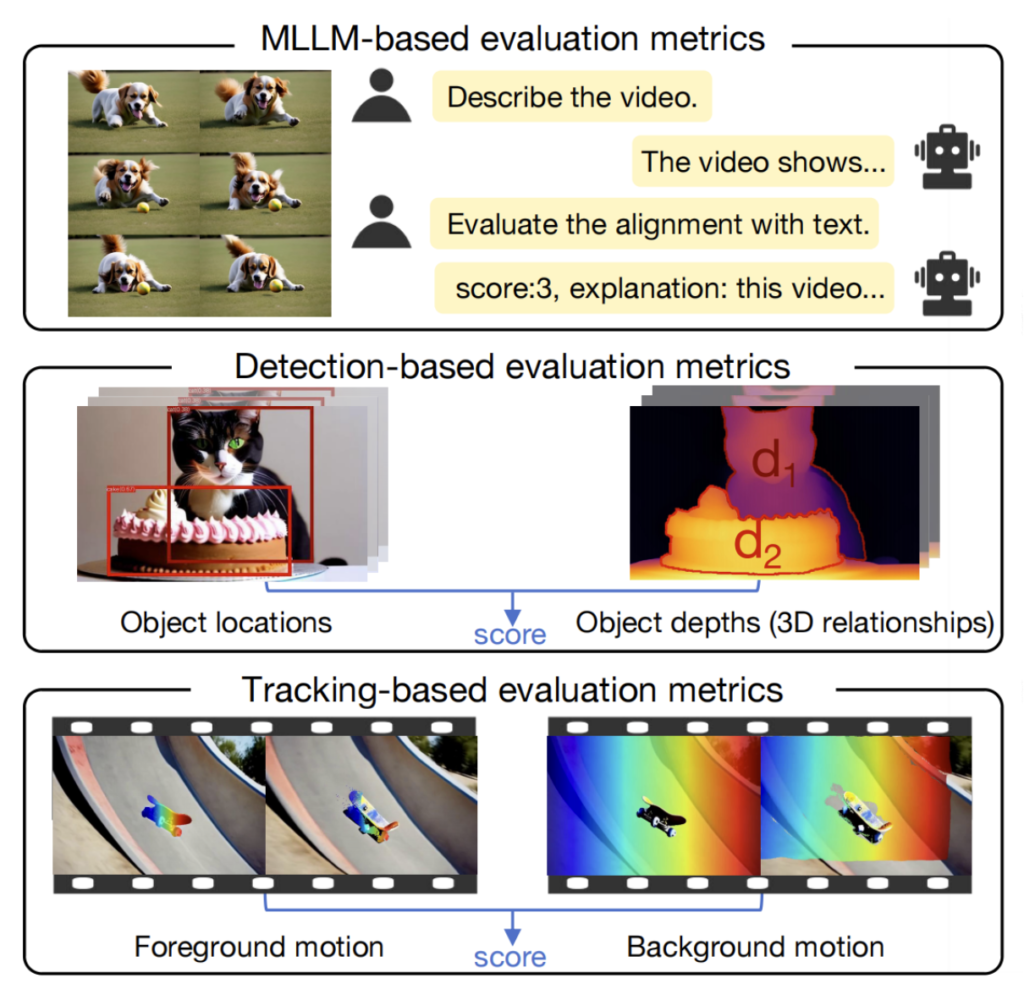
Understanding T2V-CompBench
T2V-CompBench aims to push the boundaries of T2V generation by focusing on compositionality. This benchmark encompasses various aspects, such as consistent attribute binding, dynamic attribute binding, spatial relationships, motion binding, action binding, object interactions, and generative numeracy. By doing so, it provides a robust framework to evaluate the ability of T2V models to generate complex and dynamic scenes from detailed text descriptions.
The benchmark includes 700 text prompts spread across seven categories, ensuring a wide range of scenarios and challenges for T2V models. This extensive dataset is designed to test the limits of current T2V technologies and highlight areas needing improvement.
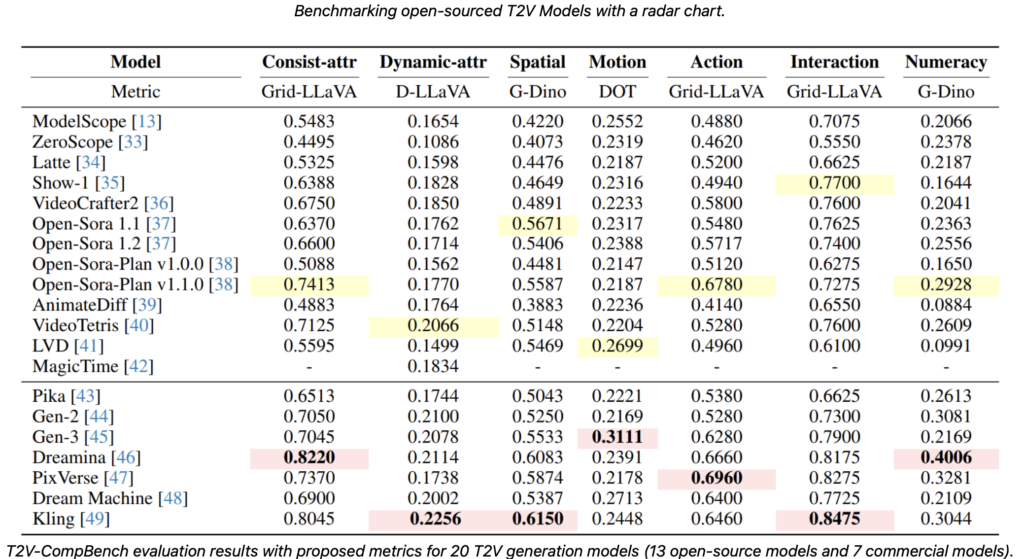
Evaluating Compositionally in T2V Generation
T2V-CompBench introduces a suite of evaluation metrics tailored to assess the compositionality of T2V models. These metrics include:
- MLLM-based Metrics: Focus on measuring the semantic alignment between the generated video and the text prompt.
- Detection-based Metrics: Evaluate the accuracy of object and attribute detection within the generated videos.
- Tracking-based Metrics: Assess the consistency and coherence of objects and actions across frames in the video.
The effectiveness of these metrics has been validated through correlation with human evaluations, ensuring that they accurately reflect the quality of compositional T2V generation.
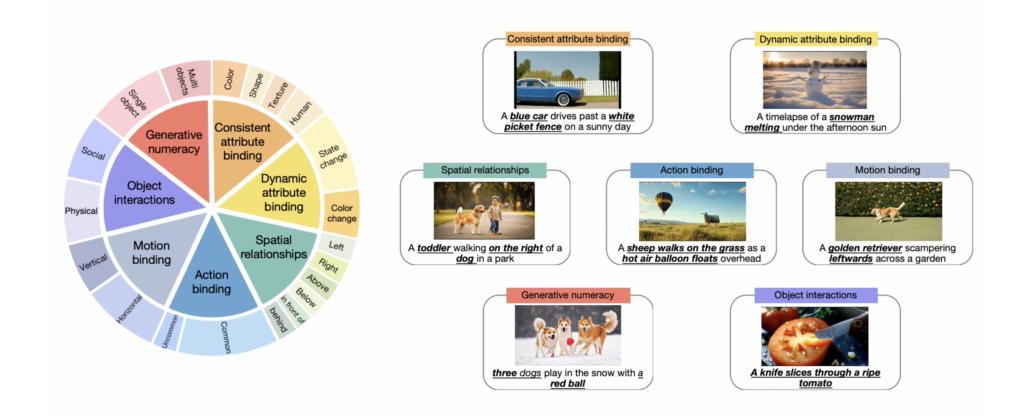
Benchmarking Current T2V Models
The introduction of T2V-CompBench also includes a benchmarking study of nine different T2V generative models. The analysis reveals that while there has been significant progress in T2V technology, achieving high-quality compositional text-to-video generation remains a formidable challenge. Current models struggle with accurately depicting multiple objects, attributes, and motions in complex scenes based on fine-grained text descriptions.
This benchmarking effort provides valuable insights into the strengths and weaknesses of existing models, offering a clear direction for future research. The goal is to inspire advancements that improve the compositionality and overall quality of T2V models.
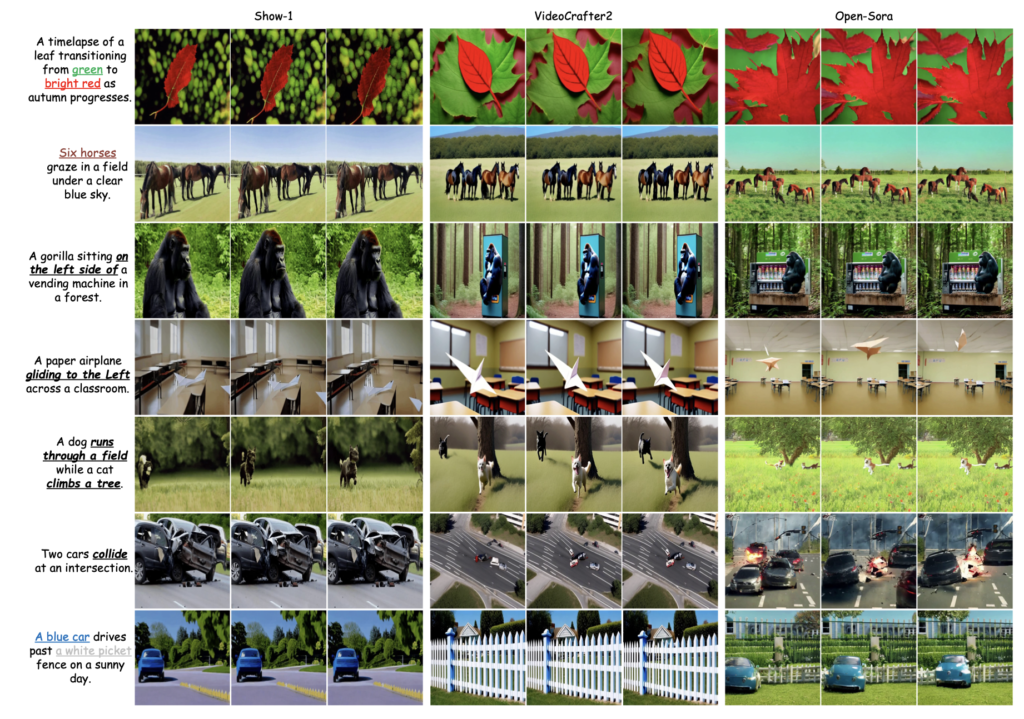
Addressing Challenges and Future Directions
One notable limitation of T2V-CompBench is the absence of a unified evaluation metric applicable across all categories. This gap highlights the need for further research into developing comprehensive evaluation frameworks for multimodal large language models (LLMs) and video understanding models. Additionally, the potential negative social impact of video generation technologies, such as the creation of misleading or fake videos, underscores the importance of ethical considerations in this field.
Despite these challenges, T2V-CompBench represents a significant step forward in the development of T2V technology. By providing a rigorous and comprehensive benchmark, it sets a new standard for evaluating and improving compositional text-to-video generation models.
T2V-CompBench is a groundbreaking benchmark that addresses a critical gap in the evaluation of text-to-video generation models. By focusing on compositionality and providing robust evaluation metrics, it offers a valuable tool for researchers and developers aiming to push the boundaries of T2V technology. As the field continues to evolve, T2V-CompBench will play a crucial role in guiding future advancements and ensuring that T2V models can generate complex, coherent, and high-quality videos from text prompts.