A Million-Scale Dataset Brings New Potential to Image-to-Video Generation Models
- Unprecedented Scale and Scope: TIP-I2V introduces over 1.7 million unique text and image prompts for image-to-video model research, offering insights into user-driven video creation.
- Enhanced Research Capabilities: By analyzing TIP-I2V, researchers can understand user preferences, build better benchmarks, and address key safety issues in AI video generation.
- Bridging Dataset Gaps: Unlike existing text-to-video or text-to-image datasets, TIP-I2V specifically supports the growing field of image-to-video generation, paving the way for innovative model development.
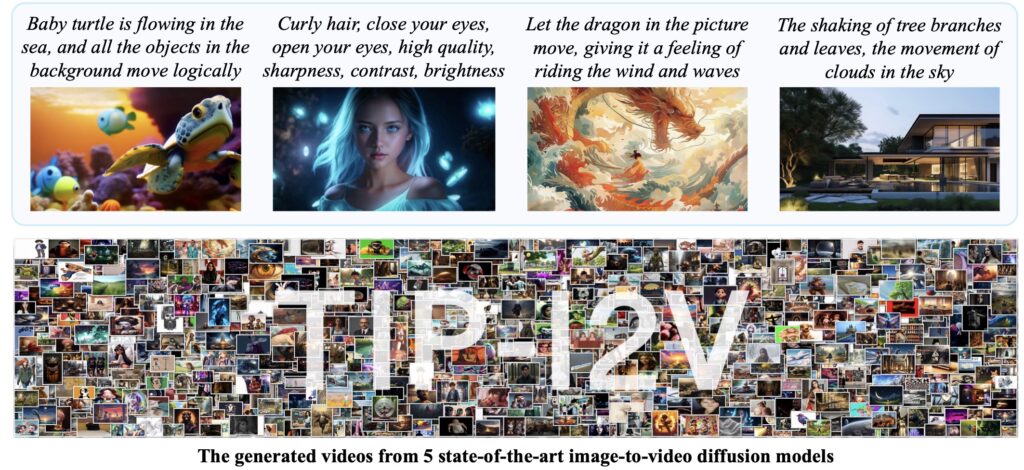
The field of AI-driven video generation is advancing rapidly, with image-to-video (I2V) models at the forefront due to their visual consistency and enhanced controllability. Despite their growing popularity, one significant gap remains: there has been no dedicated dataset for understanding the unique requirements of image-to-video prompts—until now. TIP-I2Vfills this void with over 1.7 million unique user-provided prompts, representing the first large-scale dataset tailored specifically for I2V models. This extensive resource is set to reshape research in the field, providing data essential for improving user-focused model design, safety, and evaluation.
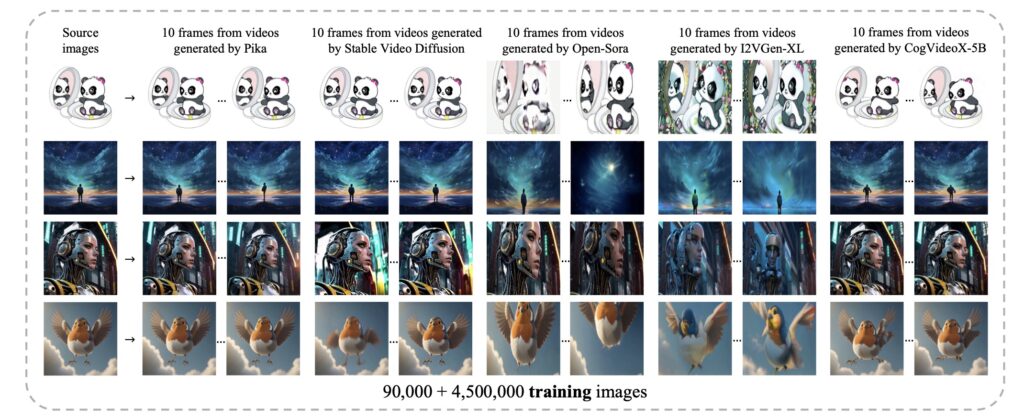
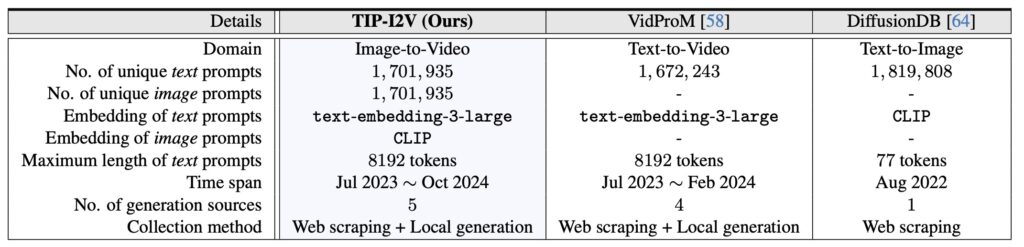
TIP-I2V: A New Dataset for New Possibilities
TIP-I2V includes text and image prompts sourced from a diverse user base, along with the corresponding generated videos from five leading image-to-video models. In comparing TIP-I2V to established datasets like VidProM (text-to-video) and DiffusionDB (text-to-image), the TIP-I2V team underscores the need for this specialized image-to-video prompt dataset. Not only does TIP-I2V offer broader prompt diversity, but it also provides insights into the semantic differences that affect video generation. These unique attributes enable TIP-I2V to serve as a foundation for improved model training and performance evaluation.
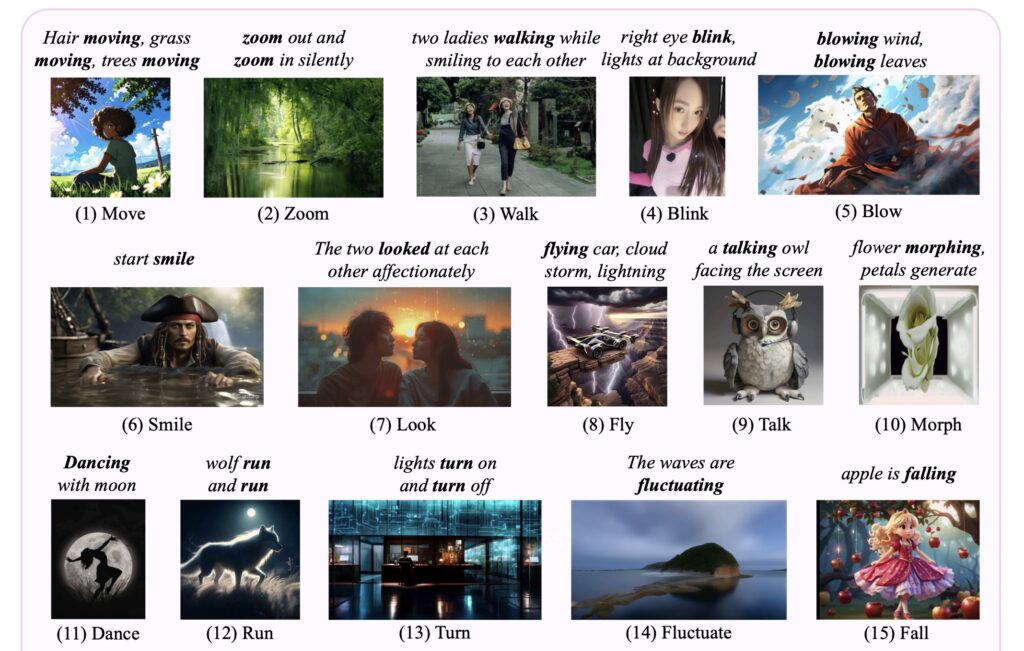
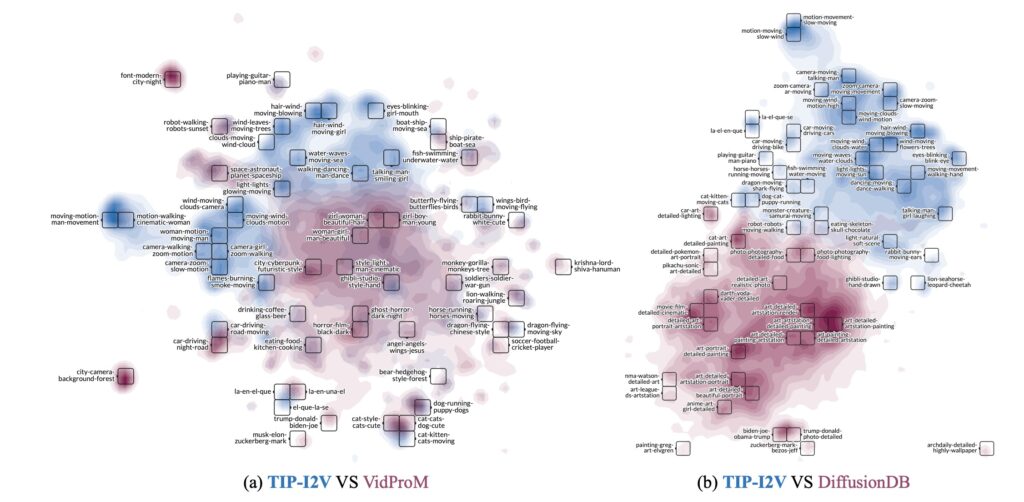
New Research Directions Powered by TIP-I2V
TIP-I2V opens up significant new avenues for research. For instance, by leveraging the dataset’s vast array of prompts, researchers can now analyze user preferences on a larger scale, adapting models to better meet these needs. Additionally, TIP-I2V supports the development of new benchmarks that more accurately evaluate model performance across multiple dimensions, such as visual quality, temporal consistency, and prompt adherence. The dataset also enables researchers to tackle emerging safety concerns, including misinformation detection by tracking generated videos back to their source images.
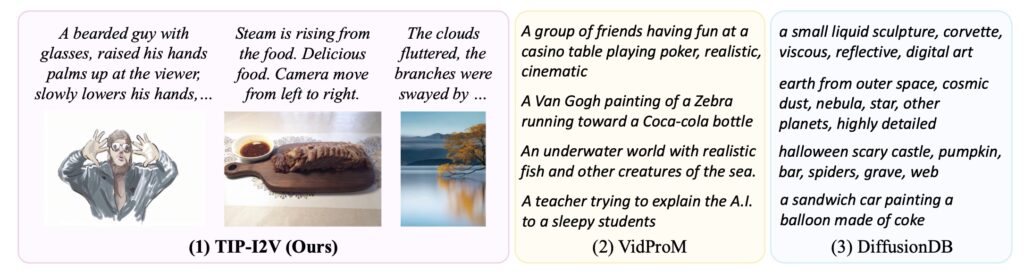
Advancing Safety and Reliability in AI-Generated Content
One of the key applications of TIP-I2V lies in improving model safety and reliability. With a specialized dataset like TIP-I2V, developers can better understand potential misinformation risks and design safeguards for accurate, trustworthy content generation. By tracing the lineage of generated videos back to their prompt sources, TIP-I2V provides a structured approach to ensuring transparency and accountability within the AI generation process, an increasingly critical consideration as AI becomes a more prominent content creation tool.
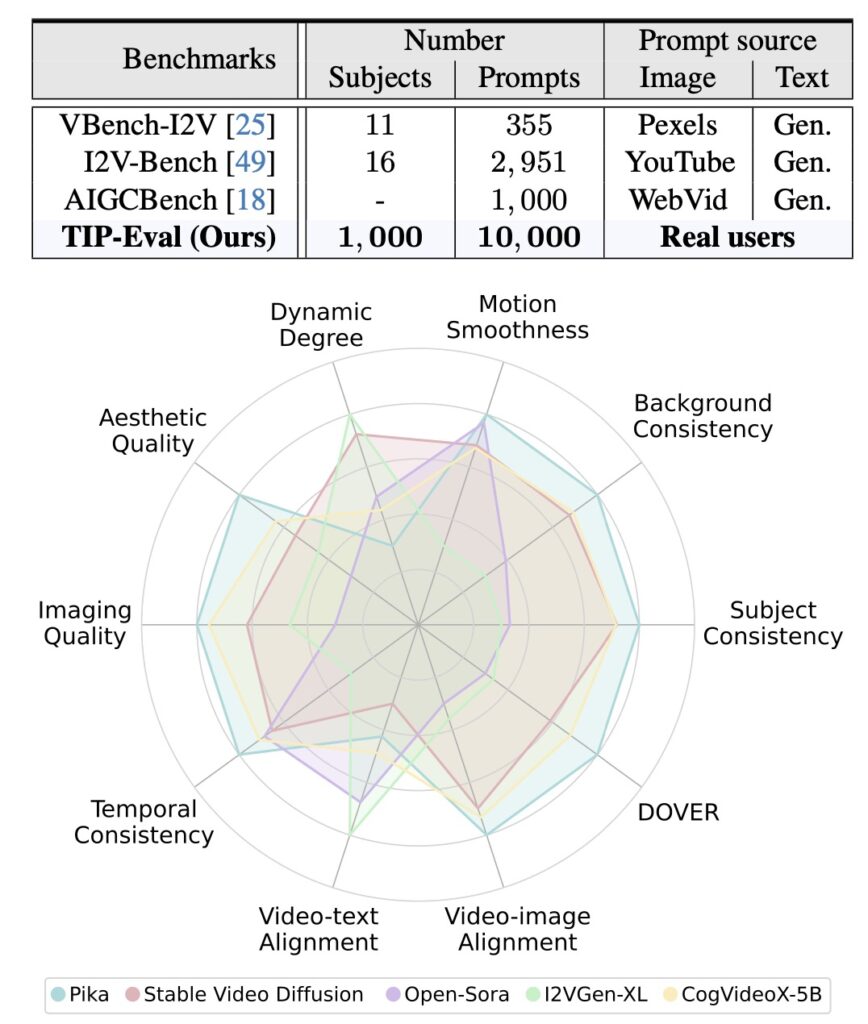
As the first dataset of its kind, TIP-I2V provides an essential resource for advancing image-to-video research and development. With its massive scale, detailed prompts, and alignment with real-world user needs, TIP-I2V is poised to drive innovation across the field, from improved user-centered models to better safety and benchmarking standards. By encouraging the research community to build upon TIP-I2V, this dataset is set to become a cornerstone in the evolution of image-to-video technology, supporting safer, more adaptable AI applications for the future.
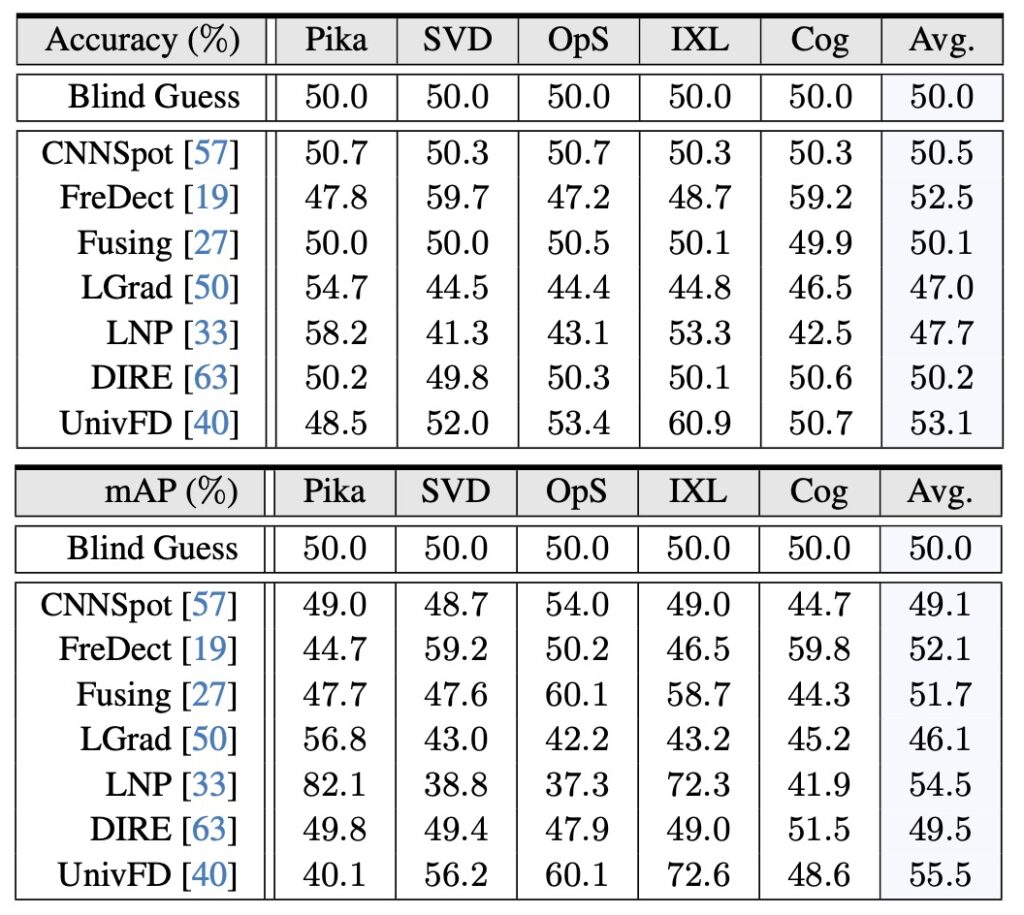
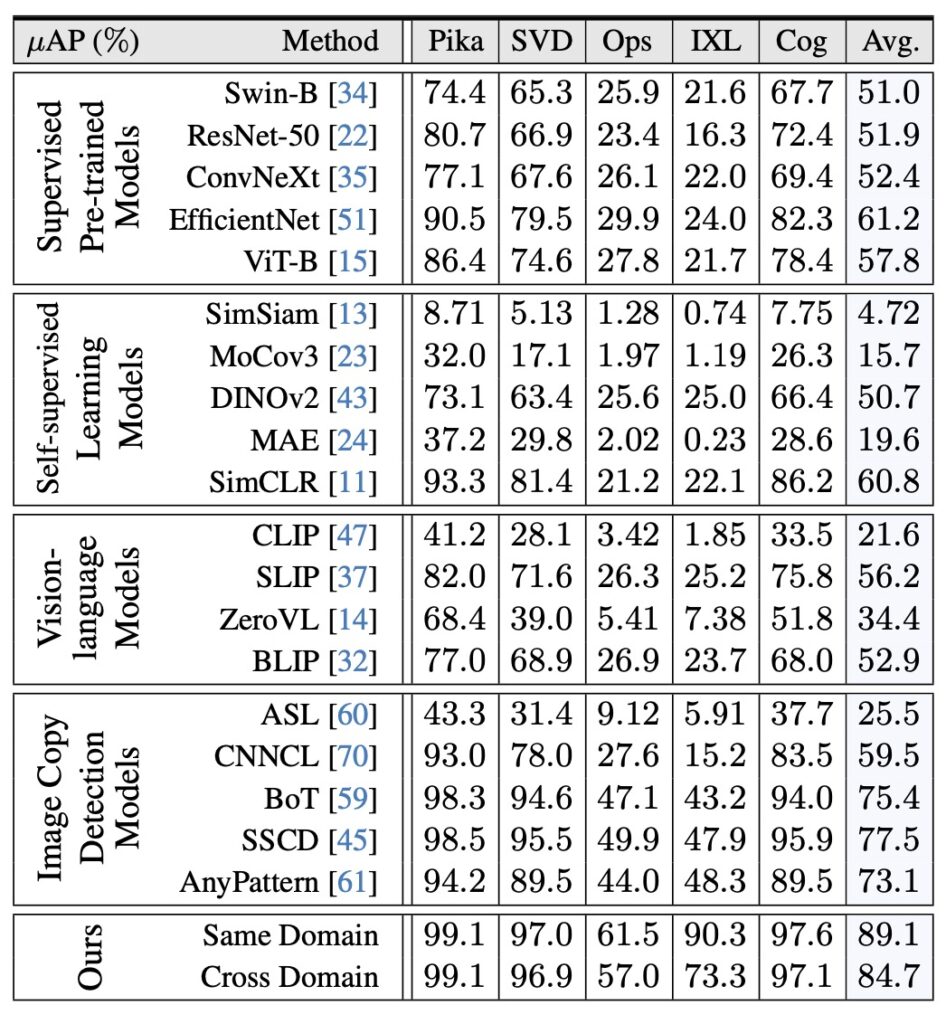