Streamlining Map Query Datasets with Unparalleled Efficiency
- Purpose of MAPQATOR: A cutting-edge system designed to efficiently annotate and create high-quality geospatial QA datasets by leveraging map APIs.
- Key Benefits: The platform offers a plug-and-play architecture, caching for consistent results, and a centralized data workflow, enhancing both speed and reliability.
- Impact and Limitations: MAPQATOR significantly reduces manual annotation time but requires familiarity with API costs and external platform stability.
Mapping and navigation services like Google Maps and Apple Maps have transformed how we interact with location-based information. While these platforms excel at offering features like route planning and contextual POI data, they falter when handling natural language geospatial queries. Addressing this challenge, recent advancements in large language models (LLMs) hint at promising capabilities for geospatial question answering (QA). However, creating reliable datasets for this purpose has remained a bottleneck due to the complexities of manual annotation and data inconsistencies.
Enter MAPQATOR—a web-based application that revolutionizes the process of developing geospatial QA datasets. By automating data collection and annotation, it bridges the gap between map services and LLMs, unlocking the potential for accurate, efficient, and reproducible geospatial datasets.
Bridging the Gap Between Maps and LLMs
The need for a system like MAPQATOR arises from the limitations of current geospatial data annotation methods. Manual approaches are time-consuming and prone to inconsistencies, making them ill-suited for training state-of-the-art language models. MAPQATOR addresses this issue with its seamless integration of various map APIs, enabling users to gather and visualize data with minimal effort. Its plug-and-play design ensures adaptability across different platforms, making it a versatile tool for researchers and developers alike.
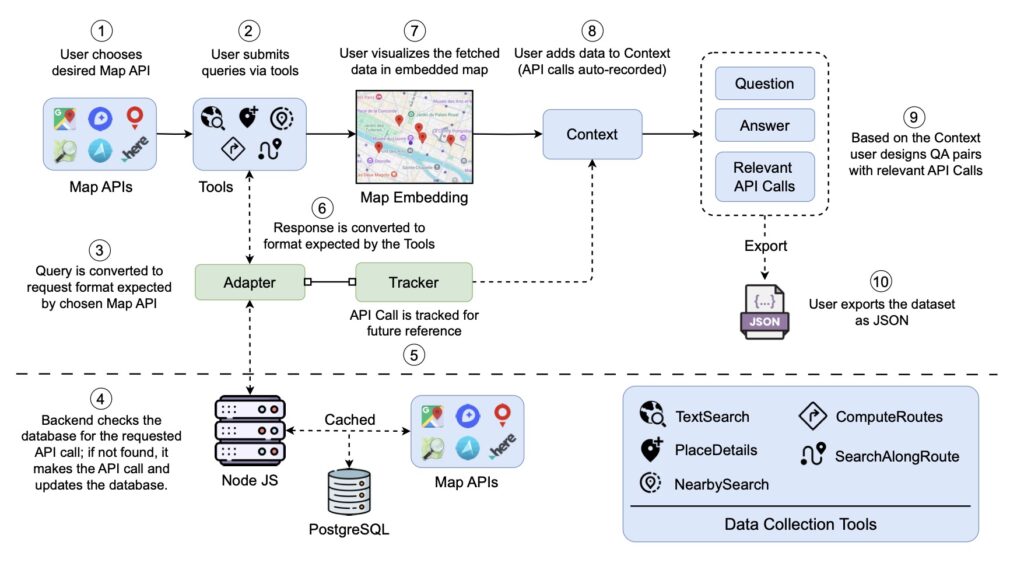
Key Features of MAPQATOR
One of the standout features of MAPQATOR is its caching mechanism, which stores API responses to provide consistent ground truths. This feature is critical as real-world map data evolves over time, ensuring that datasets remain reliable for training and evaluation. Additionally, MAPQATOR offers tools for creating geospatial questionnaires, simplifying the process of generating complex QA datasets.
The platform’s centralized architecture combines data retrieval, annotation, and visualization in one place. This not only enhances workflow efficiency but also ensures traceability—a critical factor for reproducible research.
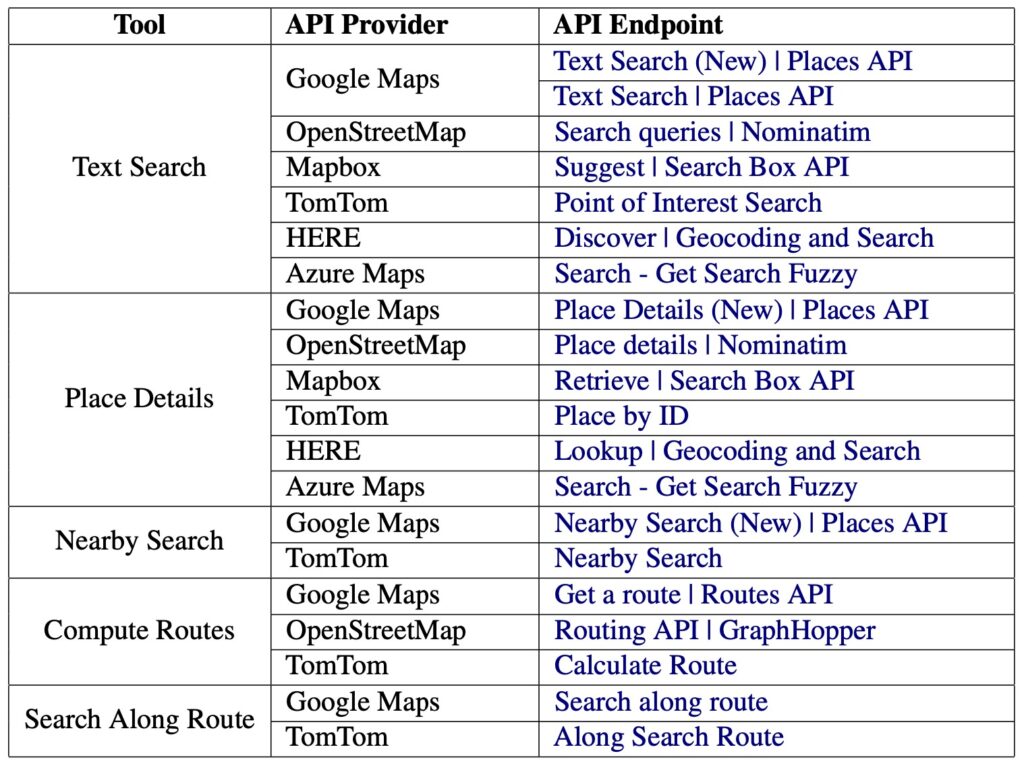
Performance and Efficiency
Experimental evaluations highlight the efficiency of MAPQATOR, showing that it speeds up the annotation process by at least 30 times compared to manual methods. For instance, while manual data retrieval for a single task could take up to 487 seconds, MAPQATOR accomplishes the same in just over 10 seconds. These metrics underscore the platform’s potential to transform how we develop geospatial QA datasets, paving the way for more sophisticated applications in spatial reasoning and navigation.
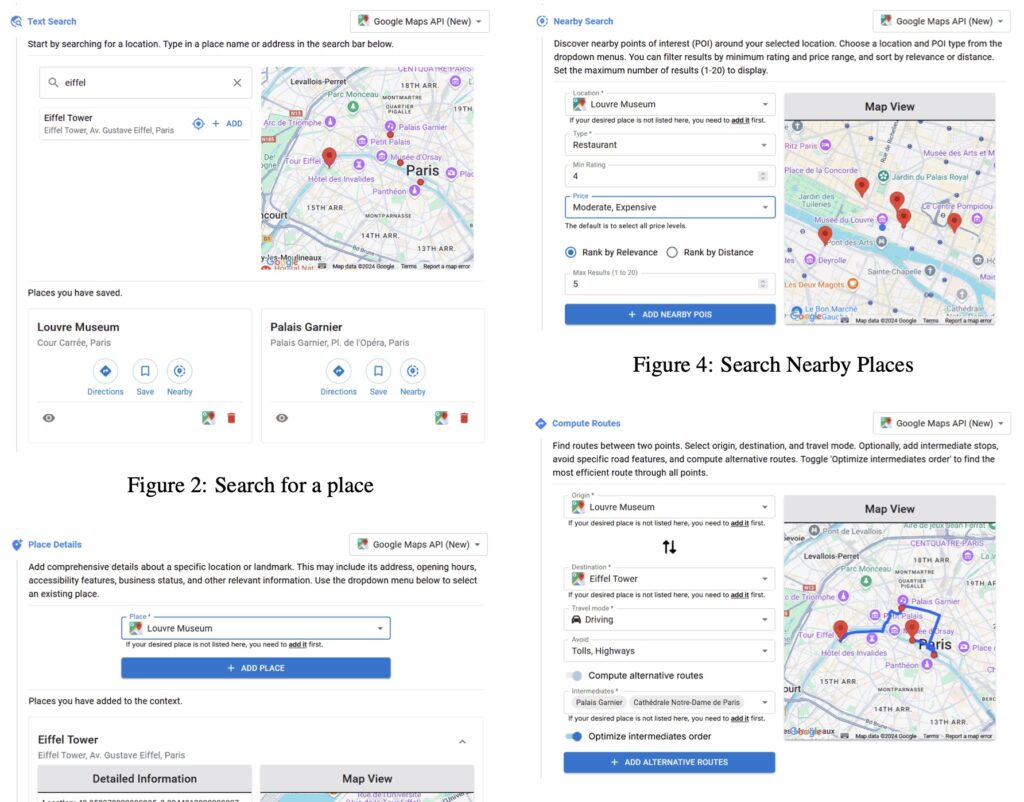
Challenges and Future Directions
Despite its advantages, MAPQATOR is not without limitations. It relies on several paid APIs, which could pose accessibility challenges for users unfamiliar with pricing structures. Moreover, the platform’s performance is tied to the availability and stability of external APIs, making it susceptible to disruptions from changes in third-party services.
Nonetheless, MAPQATOR’s modular framework offers opportunities for adaptation beyond geospatial QA, potentially extending into other domains requiring annotated datasets. Future improvements could focus on incorporating qualitative user feedback and expanding support for additional contextual data sources, such as TripAdvisor, to enrich QA datasets further.
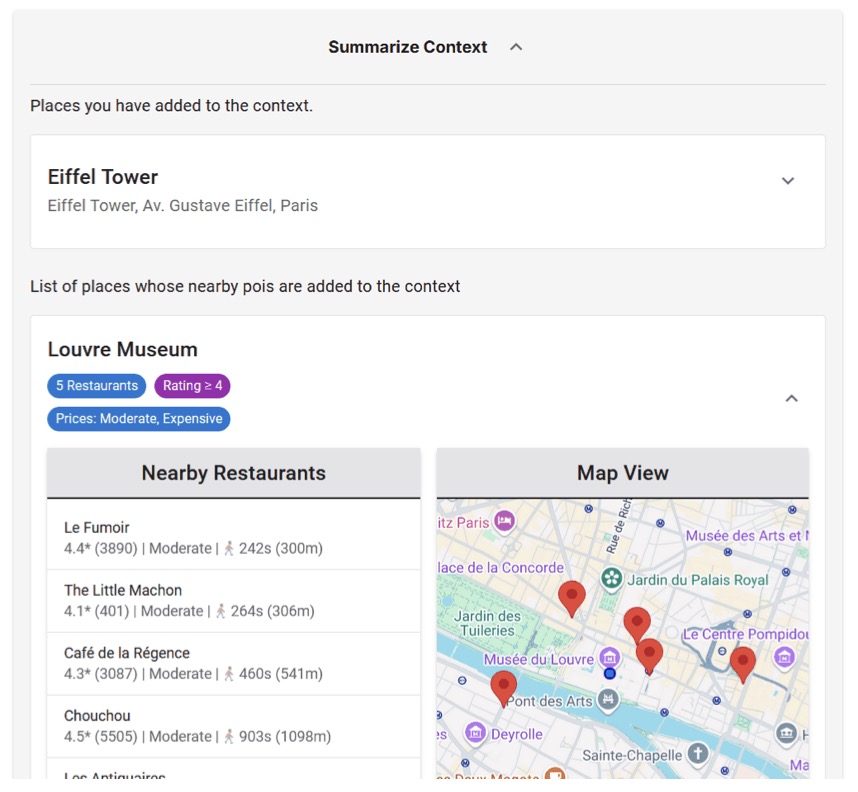
MAPQATOR represents a significant leap forward in geospatial QA dataset creation, addressing long-standing challenges of efficiency and reliability. Its innovative features and robust architecture make it an indispensable tool for advancing the capabilities of LLMs in geospatial reasoning. As the platform evolves, it holds the potential to redefine the intersection of language models and spatial understanding, unlocking new possibilities for research and application.