Transforming Text into Meshes in Seconds with Stable Diffusion
- PRD enables the adaptation of SD into a native 3D generator, eliminating the need for 3D ground-truths and allowing for scalable training data.
- The proposed TriplaneTurbo model, built upon PRD and Parameter-Efficient Triplane Adaptation (PETA), achieves high-quality 3D mesh generation in just 1.2 seconds.
- The PRD approach has the potential to be extended to various 3D generation tasks and can be applied to other pre-trained models beyond SD.
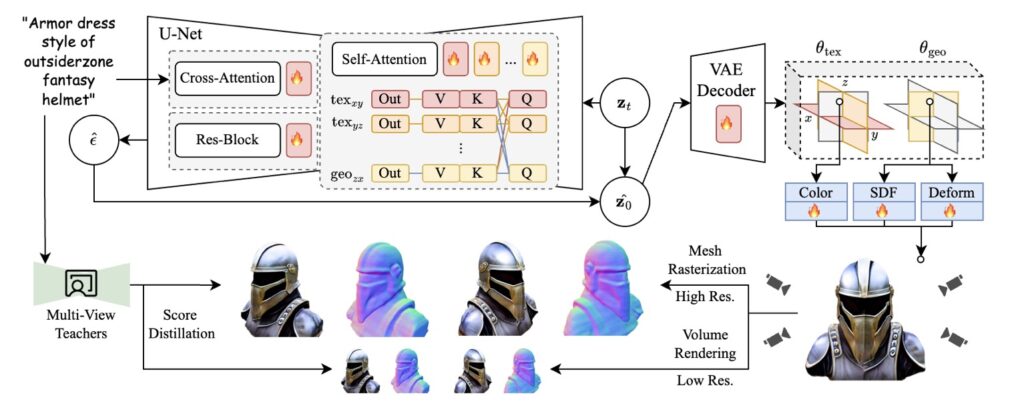
The field of 3D generation has long been hindered by the scarcity of high-quality 3D training data. Traditional approaches to adapting pre-trained text-to-image diffusion models, such as Stable Diffusion, into 3D generators often result in poor quality due to this data shortage. However, the introduction of Progressive Rendering Distillation (PRD) offers a novel solution to this challenge.
PRD revolutionizes the training process by eliminating the need for 3D ground-truths. Instead, it leverages multi-view diffusion models, such as MVDream and RichDreamer, in conjunction with Stable Diffusion to distill text-consistent textures and geometries into the 3D outputs through score distillation. This innovative approach allows for the easy scaling of training data and the improvement of generation quality, even for challenging text prompts with creative concepts.
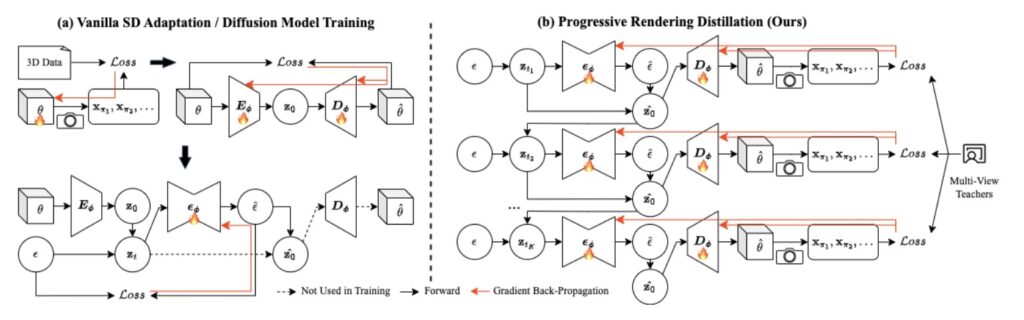
At the core of PRD lies the modification of the Markov chain used in traditional diffusion models. By transforming Stable Diffusion’s U-Net and decoder into 3D generators, PRD enables the decoding of 3D representations from the estimated latent vectors at each step of the chain. The 3D outputs are then rendered from different camera views and receive supervision from multi-view teachers via score distillation. This specialized gradient detachment strategy ensures good convergence while optimizing GPU memory usage and preventing gradient explosion.
To demonstrate the effectiveness of PRD, we introduce TriplaneTurbo, a model that adapts Stable Diffusion for Triplane generation. TriplaneTurbo utilizes two Triplanes: a geometry Triplane storing Signed Distance Function (SDF) and deformation values for mesh extraction, and a texture Triplane containing RGB attributes for painting texture on the mesh. By employing Parameter-Efficient Triplane Adaptation (PETA), which adds only 2.5% trainable parameters to the SD model, TriplaneTurbo achieves remarkable efficiency and quality in 3D mesh generation.
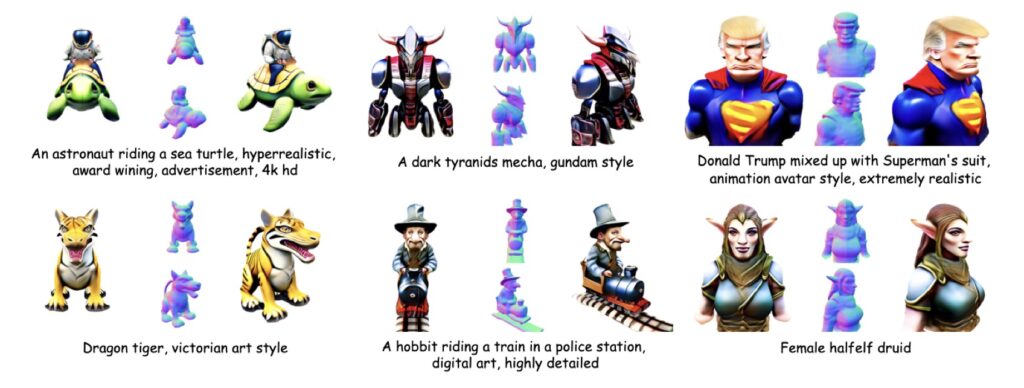
The power of TriplaneTurbo lies in its ability to produce high-quality 3D meshes in just 1.2 seconds, while also generalizing well for challenging text input. This breakthrough in instant text-to-mesh generation opens up new possibilities for various applications, from 3D modeling and animation to virtual reality and gaming.
Moreover, the PRD approach has the potential to extend beyond text-to-mesh generation. It can be applied to other 3D generation tasks, such as 3D scene generation and image-to-3D conversion. While currently implemented with Stable Diffusion, PRD can also be adapted to other pre-trained models, such as DiT, further expanding its impact on the field of 3D generation.
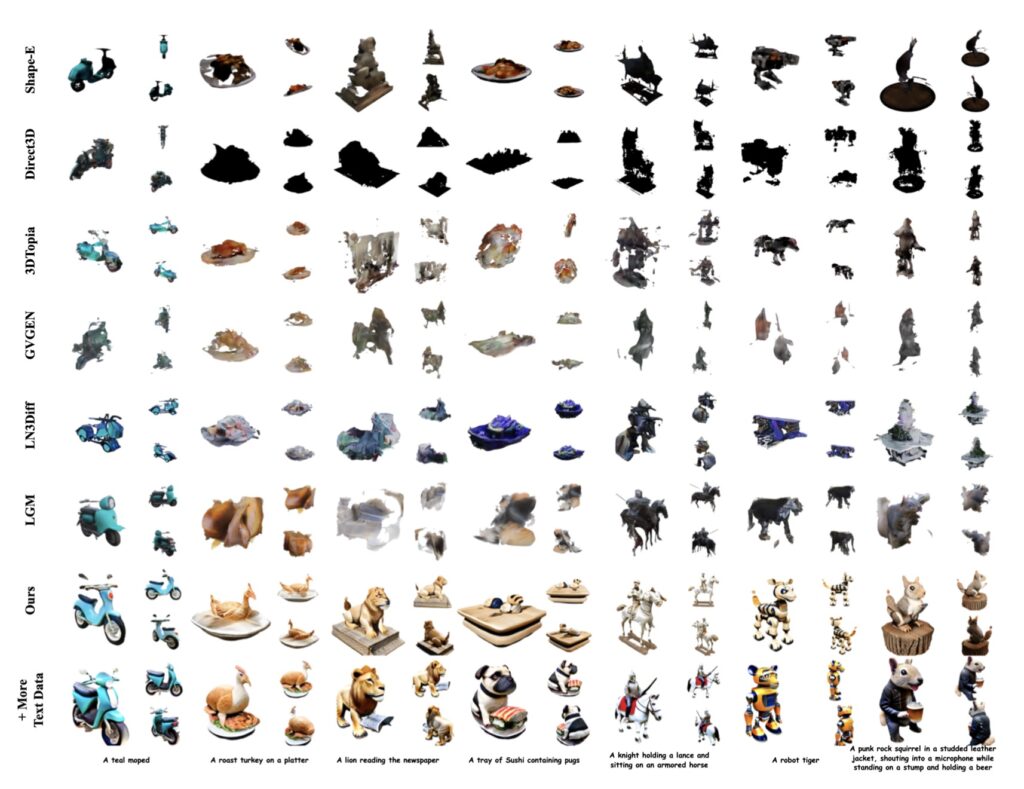
It is important to acknowledge the limitations of the proposed method. The generation of precise numbers of multiple 3D objects may require more sophisticated multi-view teachers, potentially enhanced with layout guidance. Additionally, the results for full-body humans might exhibit limited facial and hand details, which could be improved by extending the adaptation of Stable Diffusion to more advanced 3D structures beyond Triplanes.
Progressive Rendering Distillation represents a significant leap forward in the field of 3D generation. By eliminating the dependency on 3D data and enabling the adaptation of pre-trained models like Stable Diffusion, PRD paves the way for more efficient, scalable, and high-quality 3D content creation. As we continue to explore and refine this approach, we can look forward to a future where the power of text-to-3D generation is truly unleashed, revolutionizing industries and inspiring new creative possibilities.