A New Approach to Extract and Identify Multiple Intents in Complex User Queries
- Qualcomm’s research introduces a Pointer Network-based system designed to handle multiple intents within a single query in task-oriented dialogue systems.
- This study offers a groundbreaking multi-intent dataset, overcoming limitations in existing multilingual, multi-intent conversational data.
- Qualcomm’s solution shows superior performance in detecting and classifying intent spans, paving the way for more nuanced conversational AI.
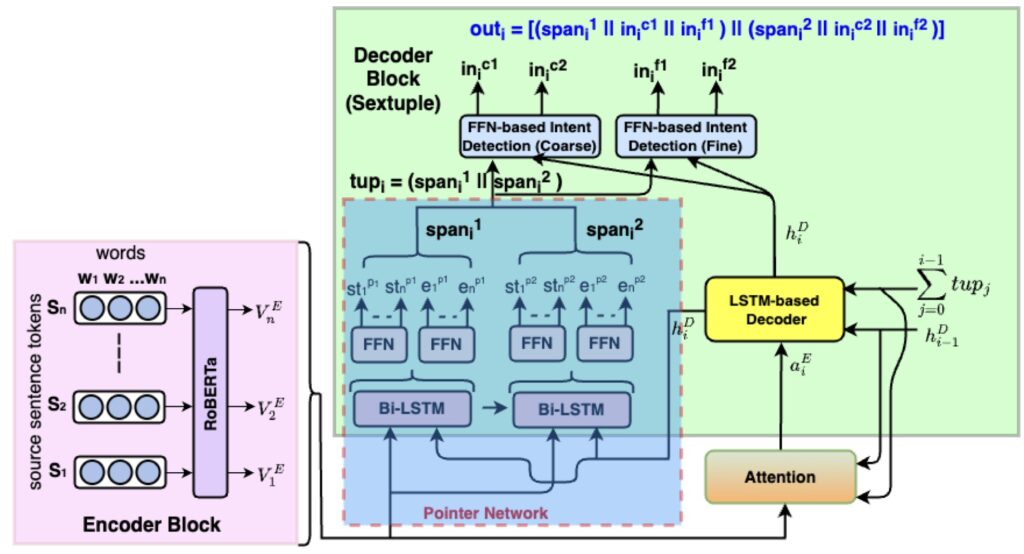
Task-oriented dialogue systems are at the forefront of natural language understanding (NLU), as they decipher user queries and provide relevant responses. While these systems are effective in managing single intents, real-world conversations often involve complex queries with multiple intents. Qualcomm’s latest research tackles this challenge by introducing a Pointer Network-based approach to detect and extract multiple intent spans within a single sentence, marking a significant leap in conversational AI. Alongside this approach, Qualcomm has developed a new multilingual, multi-intent dataset that enables accurate classification and spans extraction, even across multiple languages.
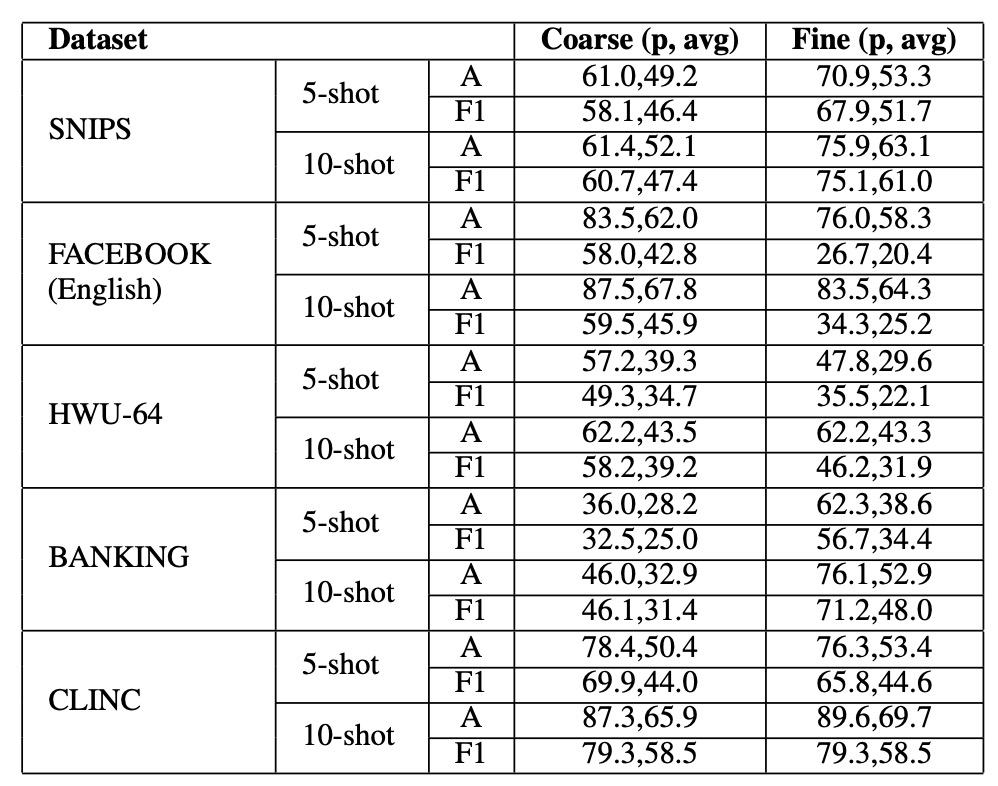
The Role of Intent Detection in Dialogue Systems
In task-oriented dialogue systems, intent detection is crucial for recognizing the user’s needs. Intent represents the purpose behind a user’s query, such as setting reminders, finding locations, or checking the weather. For example, the query, “Remind me to buy groceries tomorrow and set an alarm for 7 AM,” contains two intents—setting a reminder and setting an alarm. Qualcomm’s system not only detects these individual intents but also classifies them into primary (main focus) and non-primary (secondary) intents, enhancing the AI’s understanding of complex queries. This advancement enables AI systems to deliver responses that are more accurate and contextually aware.
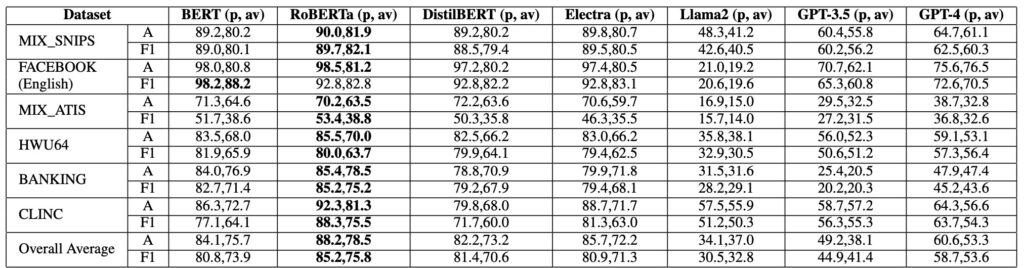
Qualcomm’s Solution: Pointer Network-Based Model for Multi-Intent Detection
To address the lack of systems capable of handling multi-intent, Qualcomm developed a Pointer Network-based modelcalled MLMCID (Multi-Label Multi-Class Intent Detection). This model uses RoBERTa for English data and XLM-R for non-English data, creating a robust framework to detect intents across languages. The Pointer Network architecture extracts intent spans (e.g., “remind me to buy groceries” and “set an alarm for 7 AM”) and identifies both coarse-grained and fine-grained intent labels. This innovation marks a significant improvement over traditional intent detection methods, as it provides a structured and detailed understanding of each query.
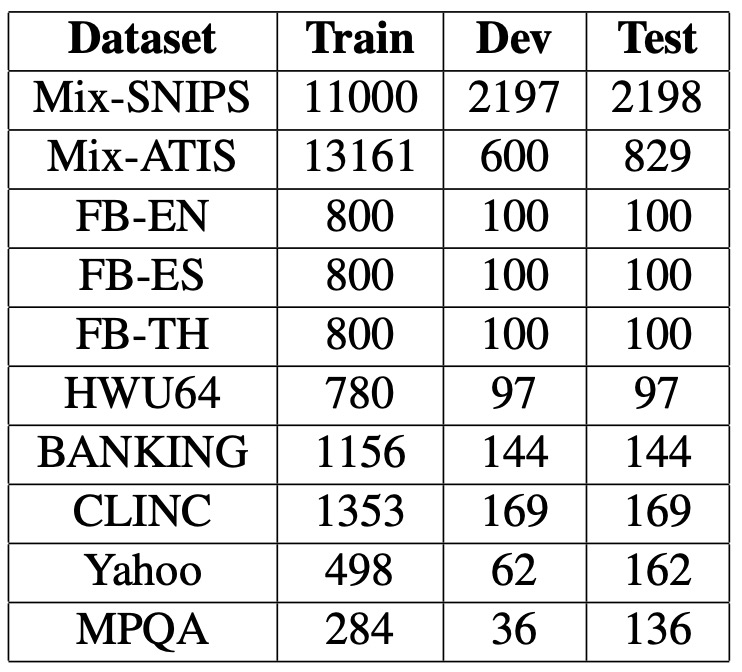
Building a New Multi-Intent Dataset for Real-World Applications
Qualcomm’s team also developed the MLMCID dataset, a multi-label, multi-class intent dataset created by integrating data from state-of-the-art benchmarks. This new dataset incorporates diverse intents across languages, making it one of the first multilingual datasets tailored for multi-intent detection. By training their Pointer Network model on this dataset, Qualcomm achieved high accuracy and F1 scores across various test sets, underscoring the dataset’s robustness. This dataset enables AI to handle more realistic conversation scenarios, including complex multi-intent queries that traditional single-intent systems cannot manage.

Future Implications for Conversational AI and NLU
Qualcomm’s pointer network-based approach represents a major step forward for multi-intent modeling in conversational AI. By demonstrating its efficacy even in few-shot scenarios, Qualcomm’s solution shows potential for rapid deployment and adaptation across new applications and languages. This work not only sets a foundation for more advanced AI dialogue systems but also provides a benchmark for future research in multilingual and multi-intent NLU.
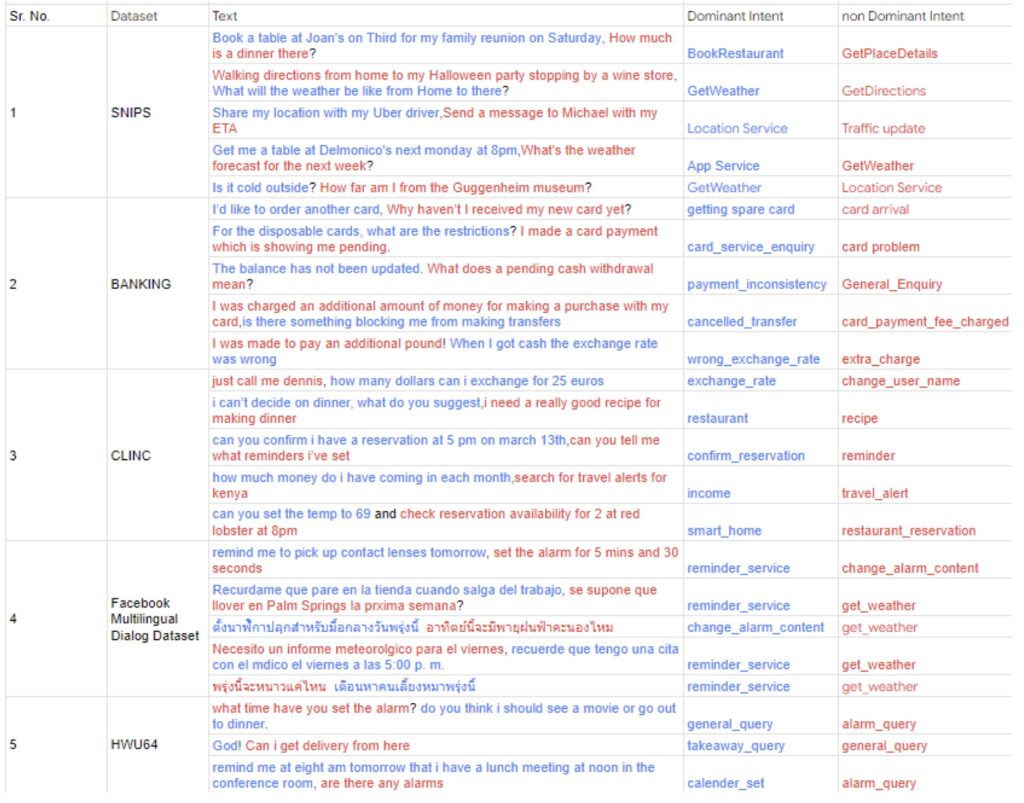
Qualcomm’s innovation in intent detection for task-oriented dialogue systems opens new horizons for conversational AI, where real-world queries often involve layered requests. With its Pointer Network architecture and comprehensive dataset, Qualcomm has laid the groundwork for future advancements that bring conversational systems closer to understanding the true complexity of human queries.