A New Approach to Ensure Originality in AI-Generated Images
- Challenge of Content Replication: While diffusion models can create stunning images, they may inadvertently replicate existing content, complicating the landscape of digital copyright and originality.
- Introducing ICDiff: To address this challenge, researchers have developed ICDiff, the first specialized Image Copy Detection (ICD) tool for diffusion models, which includes a novel dataset and deep embedding method.
- Proven Effectiveness: Experimental results demonstrate that ICDiff outperforms existing methods, successfully identifying replication in generated images and shedding light on the scale of content copying in popular diffusion models.
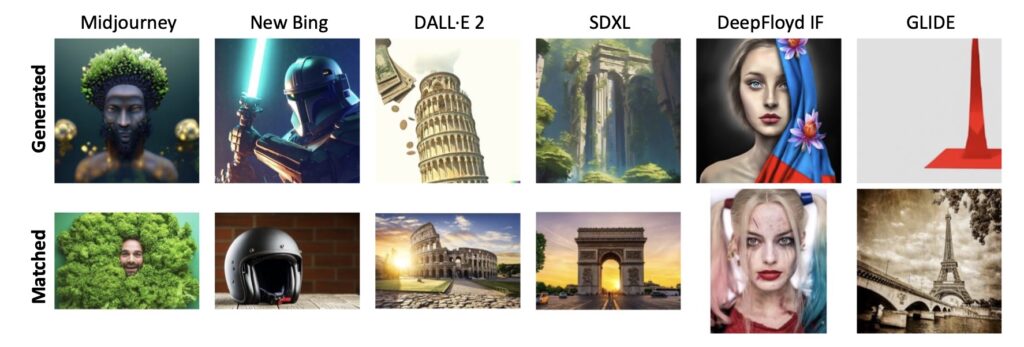
As the popularity of diffusion models continues to soar, so does the concern over the originality of the images they produce. These models, capable of generating high-quality visuals, can sometimes unintentionally replicate existing artwork, posing significant challenges in copyright and content ownership. Recognizing this pressing issue, researchers have taken the initiative to develop a specialized tool called ICDiff, aimed at detecting image copying specifically within the realm of diffusion models. This innovative approach not only enhances the understanding of content replication but also serves as a resource for maintaining originality in digital art.
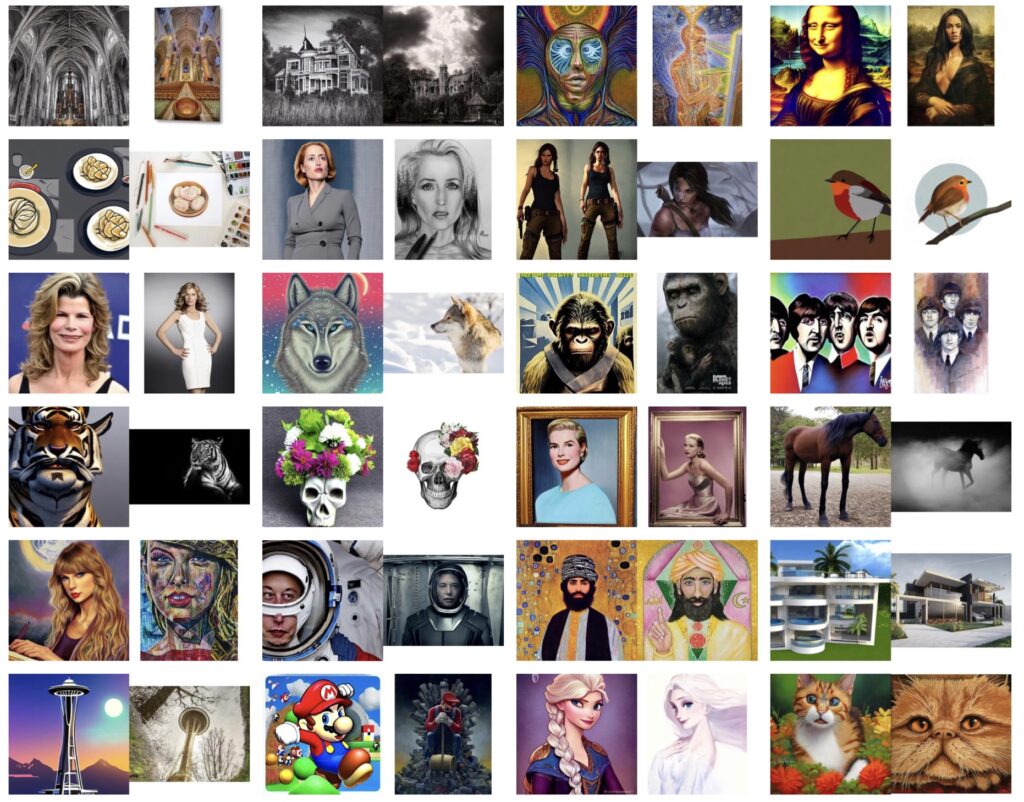
At the heart of the ICDiff project lies the creation of the Diffusion-Replication (D-Rep) dataset. This dataset consists of 40,000 image-replica pairs generated using the advanced Stable Diffusion V1.5 model. Each pair is meticulously annotated into six distinct replication levels, ranging from level 0 (no replication) to level 5 (total replication). This detailed classification allows for a nuanced understanding of how diffusion models might replicate existing content. Unlike traditional Image Copy Detection methods, which primarily focus on hand-crafted transformations, ICDiff provides a more comprehensive framework to assess the uniqueness of AI-generated images.
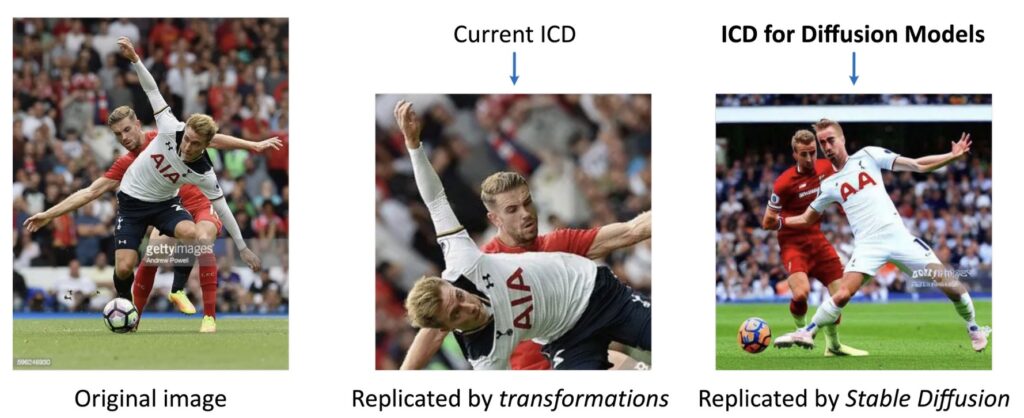
Central to ICDiff’s methodology is a novel deep embedding technique called PDF-Embedding. This approach transforms the replication levels of image-replica pairs into probability density functions (PDFs) that serve as supervision signals during the detection process. The underlying intuition is that the probabilities of neighboring replication levels should exhibit continuity and smoothness. By adopting this innovative technique, ICDiff outperforms existing protocol-driven methods and demonstrates a greater ability to accurately identify content replication across various diffusion models.
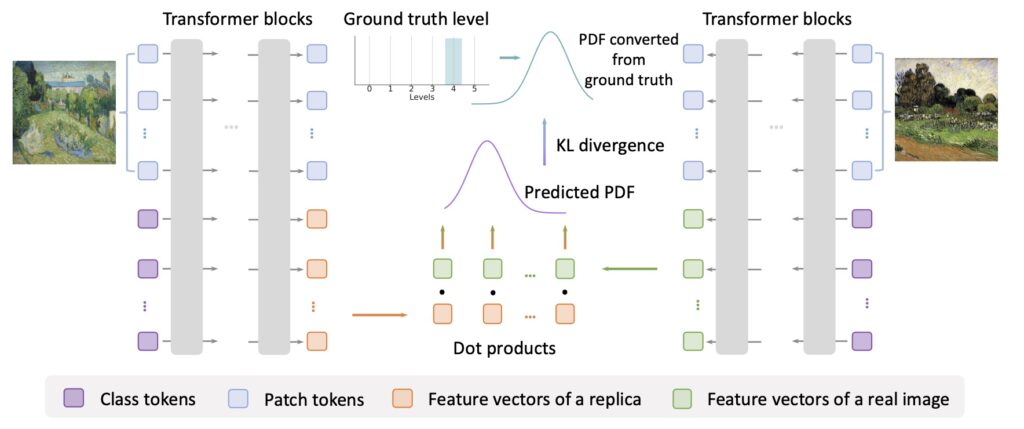
Experimental results reveal alarming statistics regarding content replication: the replication ratios of popular diffusion models against an open-source gallery range from 10% to 20%. This finding highlights the pressing need for tools like ICDiff, as it sheds light on the extent of copying occurring in the digital art space. With these insights, artists, developers, and legal professionals can better navigate the complexities surrounding copyright infringement and fair use.
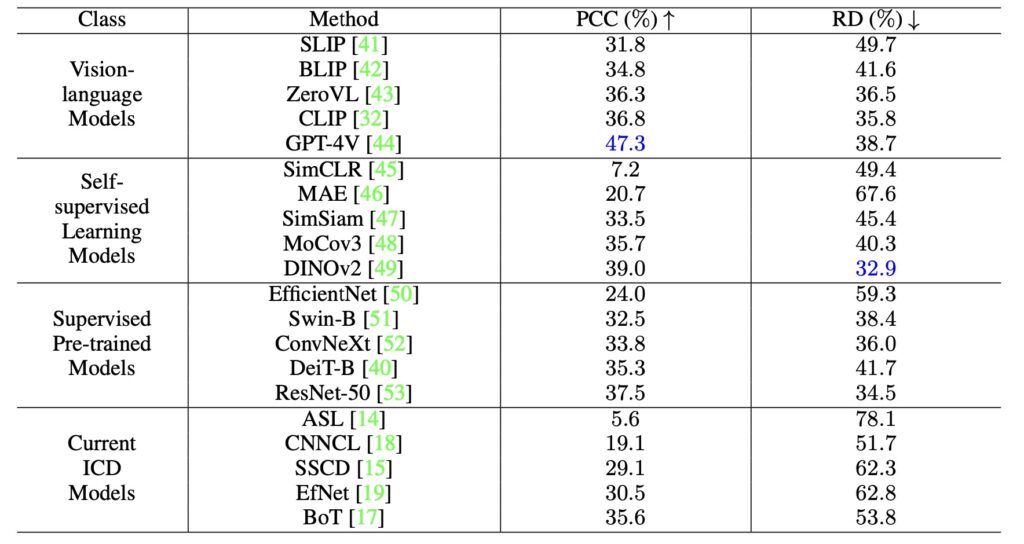
The introduction of ICDiff marks a significant advancement in the field of image copy detection. By addressing the unique challenges posed by diffusion models, this research paves the way for improved originality and accountability in AI-generated content. As the line between inspiration and imitation becomes increasingly blurred, the need for effective detection tools has never been more critical. The ICDiff project not only serves as a foundation for future research but also provides a valuable resource for maintaining the integrity of digital artistry in an era defined by rapid technological advancement.
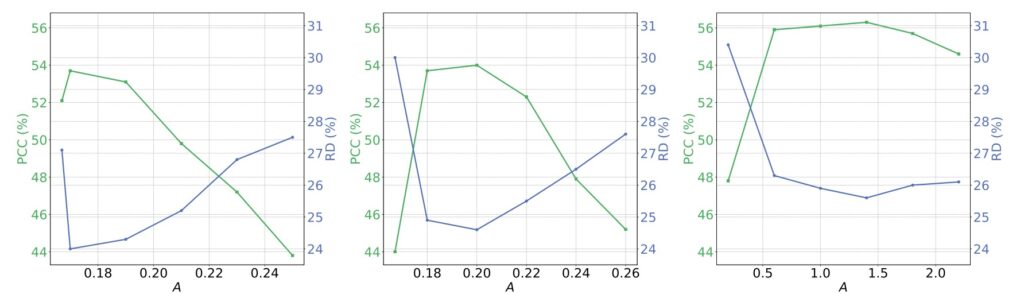
The development of ICDiff represents a crucial step toward understanding and addressing the challenges of content replication in the realm of diffusion models. By equipping researchers and artists with powerful detection tools, ICDiff aims to foster a more transparent and equitable digital art landscape, ensuring that creativity thrives while respecting the boundaries of originality. As we move forward, continued innovation in this field will be essential to uphold the rights of creators and promote a culture of responsible artistic expression.
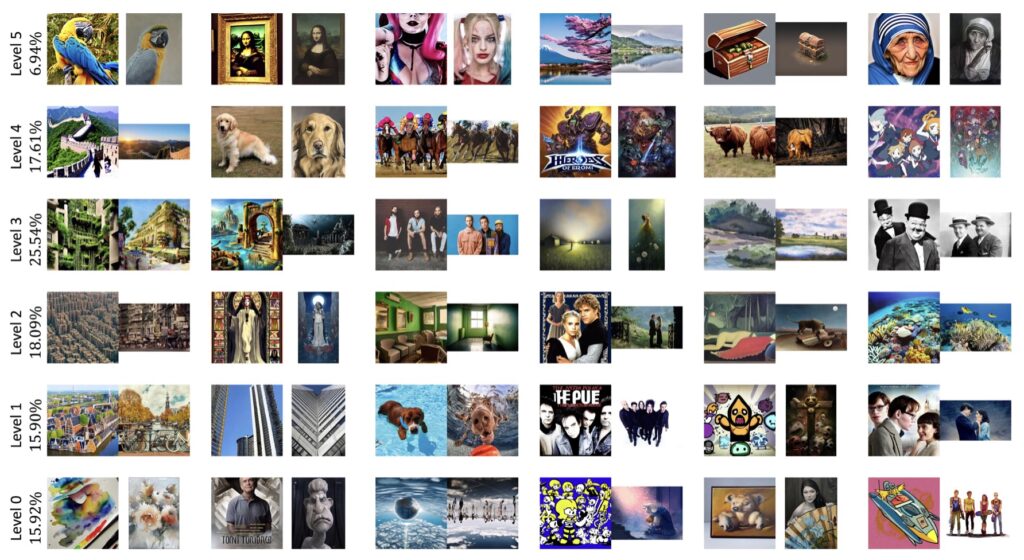