How Context-Regularized Text Embedding is Setting New Standards in Image Synthesis.
In the rapidly evolving field of text-to-image personalization, a new player has emerged that promises to enhance the quality and relevance of generated images. Here’s a snapshot of what makes CoRe stand out:
- Balancing Act: CoRe addresses the persistent challenge of balancing identity preservation with text alignment in image generation. By fine-tuning how text embeddings interact with context tokens, CoRe ensures that generated images are both faithful to the original concept and closely aligned with the text prompt.
- Context Matters: The key to CoRe’s effectiveness lies in its innovative use of Context Regularization. This technique enhances the learning of new text embeddings by regularizing their interaction with surrounding context tokens, ensuring that each element in the prompt is accurately represented.
- Versatility and Performance: CoRe’s design allows it to be applied to arbitrary prompts without needing image generation for training, significantly improving its generalization capabilities. Experimental results show that CoRe outperforms existing methods in terms of both identity preservation and text alignment, offering a new level of personalization.
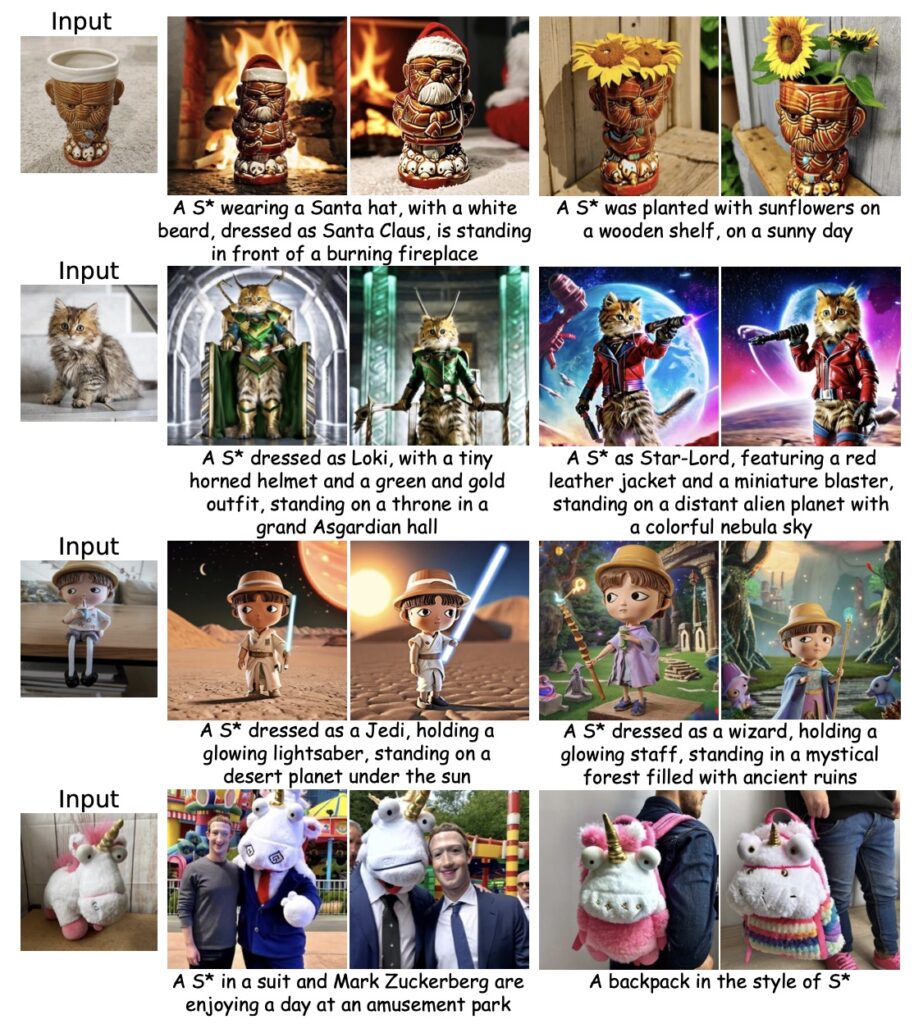
In recent years, text-to-image personalization has made significant strides, enabling high-quality and tailored image synthesis based on user-provided concepts and text prompts. However, despite these advancements, a crucial challenge remains: finding the right balance between preserving the identity of the concept and ensuring accurate text alignment.
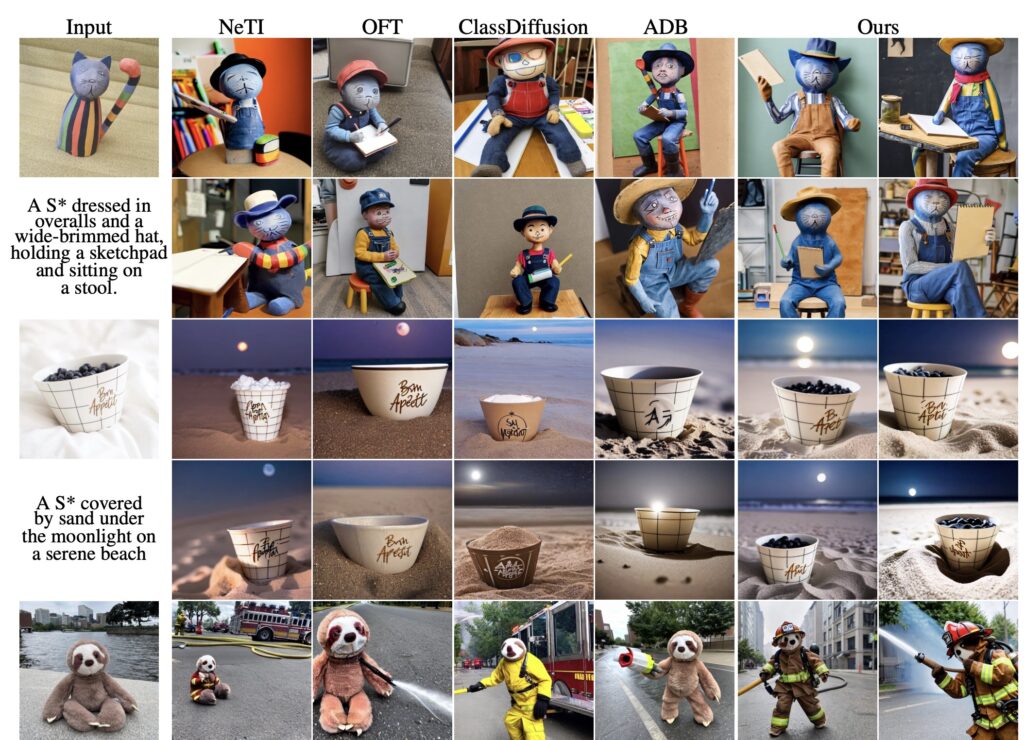
Introducing Context-Regularized Text Embedding Learning (CoRe), a novel approach designed to tackle this issue head-on. Traditional methods have struggled to achieve a seamless integration of new concepts into the pre-trained models, often resulting in either loss of identity or poor text alignment. CoRe seeks to bridge this gap by focusing on the semantic understanding of prompts and their context within the CLIP text encoder.
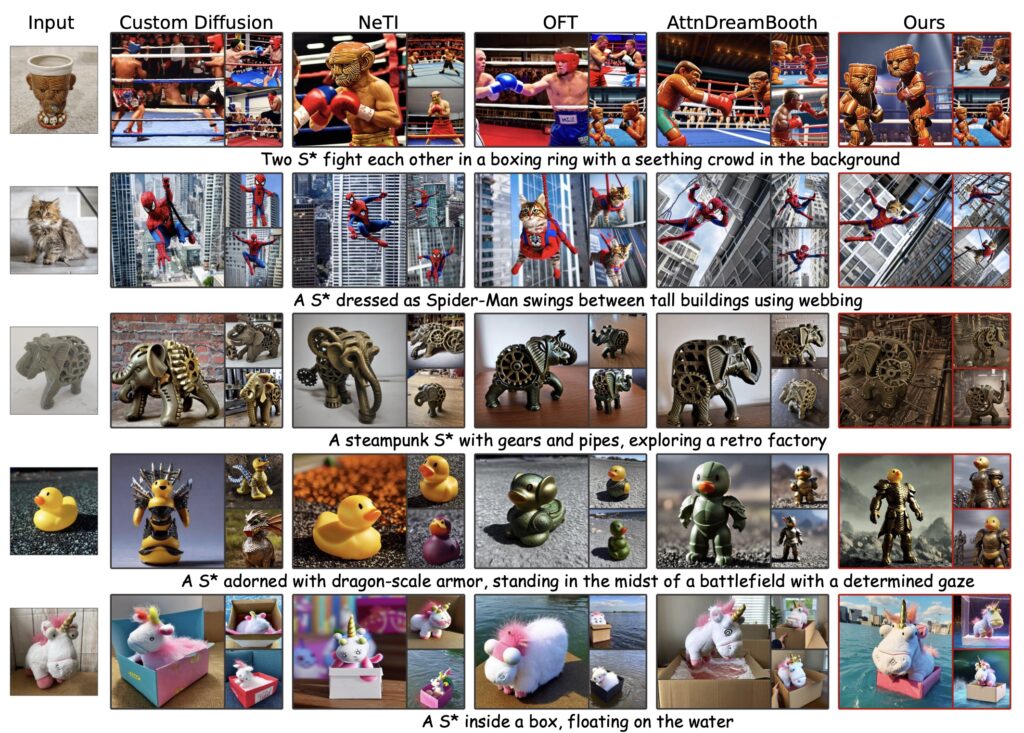
CoRe operates on the principle that effective text-to-image personalization requires a precise semantic representation of the prompt. This is managed by the CLIP text encoder, which processes the interactions between text tokens. CoRe enhances this process by embedding new concepts into the input space of the text encoder with a refined approach. By regularizing the context tokens in the prompt, CoRe ensures that the new concept’s text embedding is learned accurately and integrates smoothly with existing tokens.
One of the standout features of CoRe is its versatility. It can be applied to any prompt without the need to generate corresponding images for training. This aspect not only simplifies the training process but also enhances the generalization of the text embeddings. Furthermore, CoRe can be utilized as a test-time optimization technique, refining the generation of complex compositions and specific prompts.
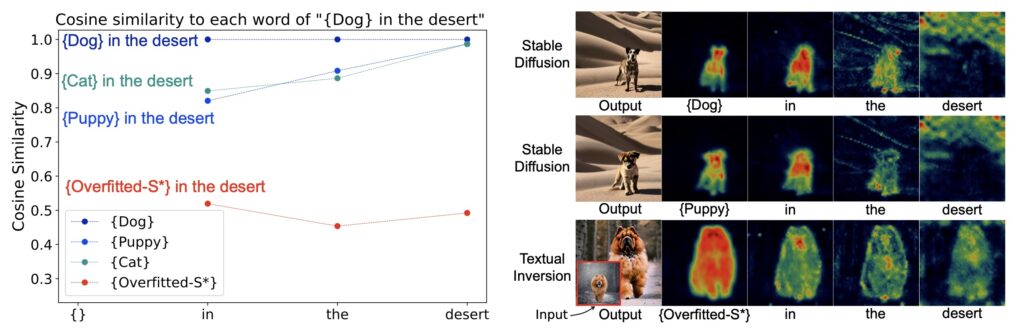
Our comprehensive experiments have demonstrated that CoRe significantly outperforms existing baseline methods, particularly in maintaining identity preservation and improving text alignment. However, as with any advanced technique, CoRe is not without its challenges. Difficult compositions involving intricate interactions between learned concepts and other objects can still pose difficulties, a challenge partly inherited from the pre-trained models.
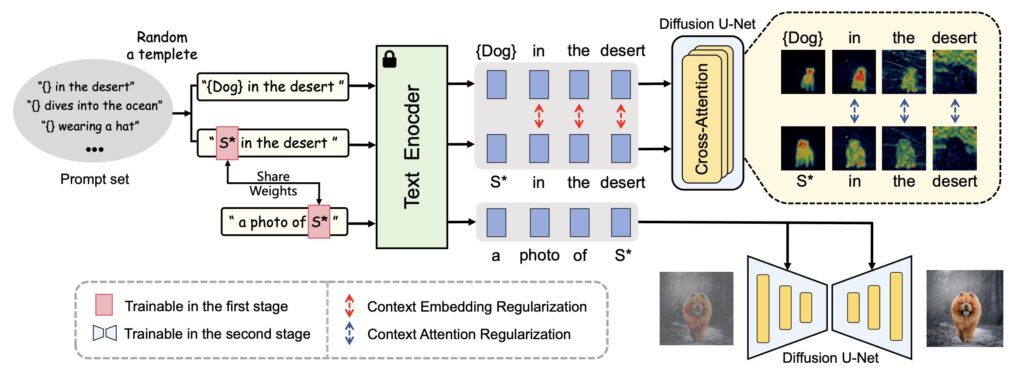
CoRe represents a major advancement in text-to-image personalization, offering improved performance and flexibility. By focusing on context regularization, CoRe sets a new standard in how text embeddings are learned and utilized, paving the way for more accurate and personalized image generation. For those interested in exploring this innovative approach, the code for CoRe will be made publicly available, inviting further research and development in this exciting field.