New AI model VideoTetris tackles the challenge of generating complex, long-form videos from text prompts, offering improved spatial and temporal composition.
- Enhanced Video Generation: VideoTetris introduces spatio-temporal compositional diffusion to create detailed and coherent videos from complex text prompts.
- Advanced Data Preprocessing: The model uses enhanced video data preprocessing to improve motion dynamics and prompt understanding, ensuring consistency and quality.
- Improved Long-Form Video Creation: VideoTetris excels in generating long videos, maintaining accurate and consistent object placement and interactions.
Recent advancements in diffusion models have significantly improved text-to-video (T2V) generation. However, these models often struggle with complex scenarios involving multiple objects or dynamic changes. To address these challenges, a new framework called VideoTetris has emerged, promising to revolutionize the way we generate videos from textual descriptions.
Tackling Complex Video Generation
Traditional T2V models face limitations when dealing with compositional prompts and long-form videos. For instance, generating a scene described as “A man on the left walking his dog on the right” can be challenging for existing models, which may fail to accurately position and animate multiple objects. VideoTetris overcomes these hurdles with a novel approach called spatio-temporal compositional diffusion.
This technique manipulates the cross-attention values of denoising networks both spatially and temporally, allowing for precise and consistent composition of objects and actions throughout the video. By enhancing the model’s ability to understand and execute complex prompts, VideoTetris achieves remarkable fidelity and coherence in video generation.
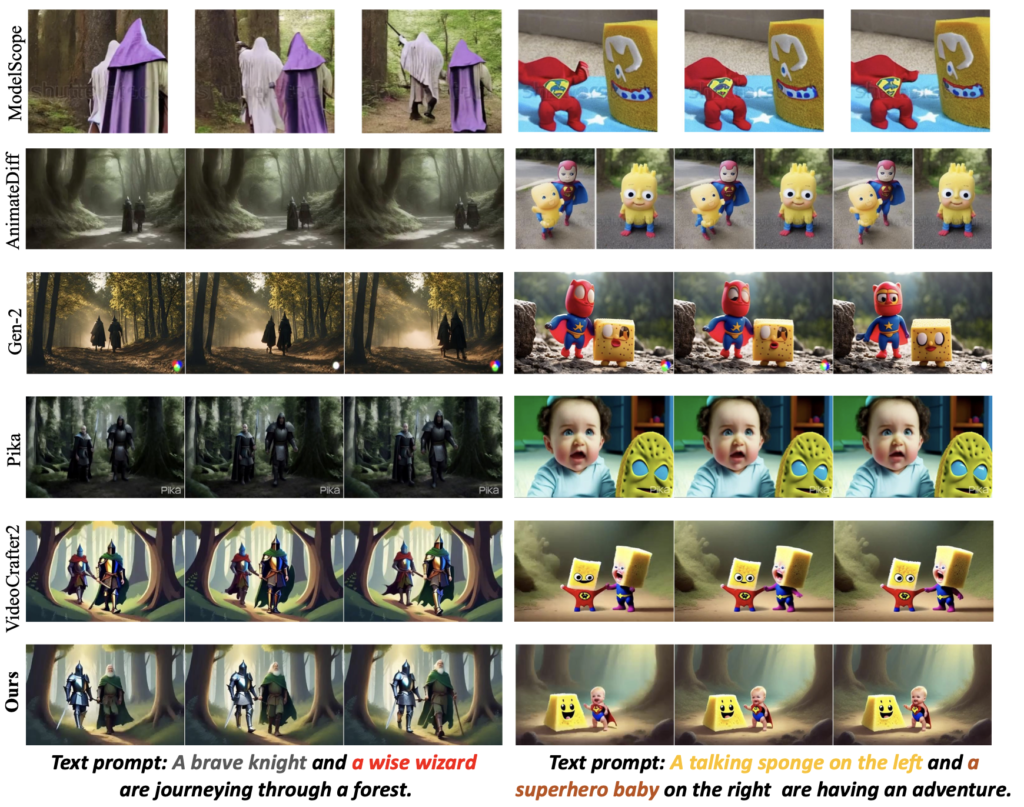
Enhanced Data Preprocessing and Consistency
One of the key innovations of VideoTetris is its enhanced video data preprocessing pipeline. This process enriches the training data with improved motion dynamics and semantic understanding, enabling the model to generate intricate scenes with various attributes and relationships. Additionally, VideoTetris introduces a Reference Frame Attention mechanism, which maintains content consistency across different frames and positions, ensuring a seamless viewing experience.
The model’s ability to handle long videos with progressive prompts is particularly noteworthy. Unlike other models that struggle with abrupt transformations and color distortions, VideoTetris excels in integrating new characters and maintaining consistent object positions and quantities. This makes it ideal for generating narrative-driven content that requires detailed and dynamic scene changes.
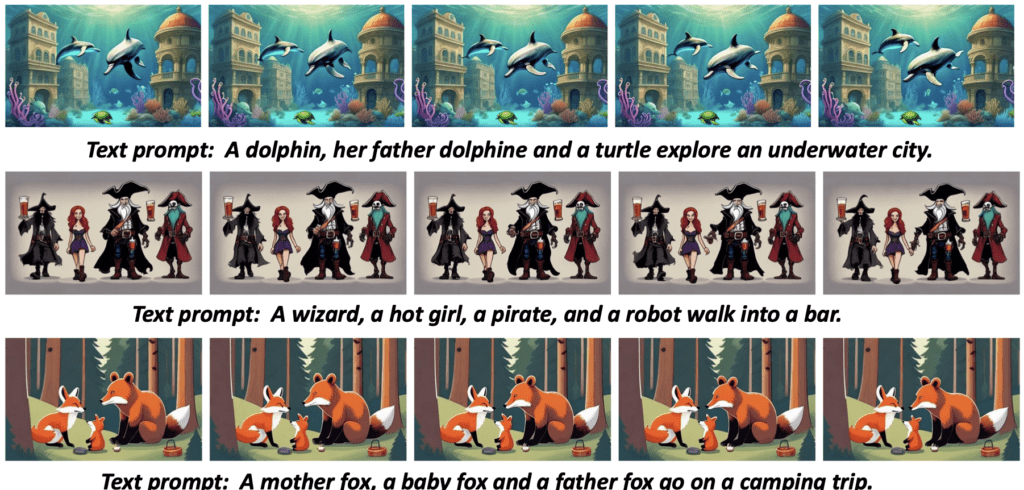
Superior Performance in Compositional Video Generation
VideoTetris sets itself apart from other models through its impressive performance in both short and long video generation. In experiments, VideoTetris accurately composed objects with their respective attributes, maintaining their positions and interactions as described in the text prompts. This contrasts with models like ModelScope and AnimateDiff, which often fail to interpret positional information correctly and merge attributes inappropriately.
In long video generation, VideoTetris demonstrates significantly higher motion dynamics and narrative coherence compared to models like FreeNoise and StreamingT2V. By avoiding the pitfalls of abrupt transformations and maintaining consistent object interactions, VideoTetris produces videos that are more visually appealing and semantically accurate.
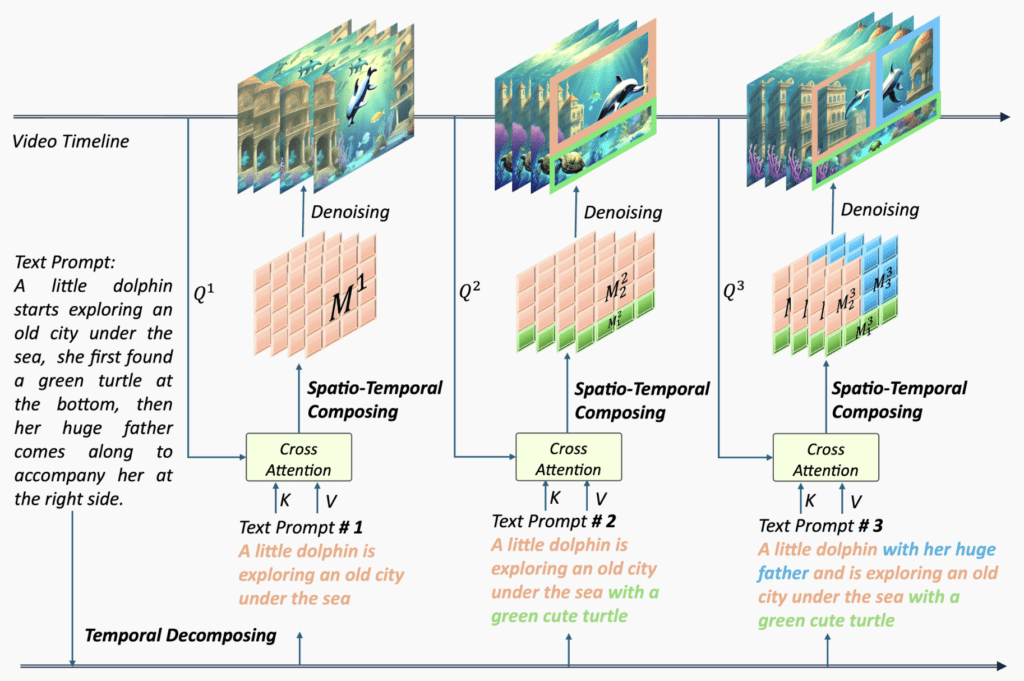
Future Directions and Broader Impact
Despite its strengths, VideoTetris still faces challenges, particularly in generating very long videos with consistent object transitions. The current reliance on ControlNet for auto-regressive generation can lead to excessive computation costs and control issues. Future work aims to explore more efficient training techniques and expand the capabilities of VideoTetris using larger, high-quality video datasets.
The advancements brought by VideoTetris open new possibilities in creative design, autonomous media, and other fields. However, the dual-use nature of this technology raises concerns about potential misuse, such as deepfakes and impersonation. It is crucial to emphasize that VideoTetris is designed to enhance legitimate image generation and does not support malicious applications.
VideoTetris represents a significant leap forward in text-to-video generation, offering a robust solution for creating complex, long-form videos from detailed text prompts. With its innovative spatio-temporal compositional diffusion and enhanced data preprocessing, VideoTetris sets a new standard for video generation models, paving the way for more advanced and versatile applications in the future.