Tailoring AI-Generated Images to Individual Tastes
- ViPer personalizes image generation by capturing and applying individual visual preferences.
- The system uses user comments to infer visual likes and dislikes, guiding generative models accordingly.
- User studies show ViPer’s effectiveness in producing images that align with personal preferences.
As generative models continue to revolutionize the creation of digital content, one significant challenge remains: personalizing these models to cater to individual tastes. Enter ViPer, a novel approach designed to tailor AI-generated images to reflect an individual’s unique visual preferences. This system marks a significant step forward in making generative AI more user-centric, enhancing applications from image editing to style transfer.
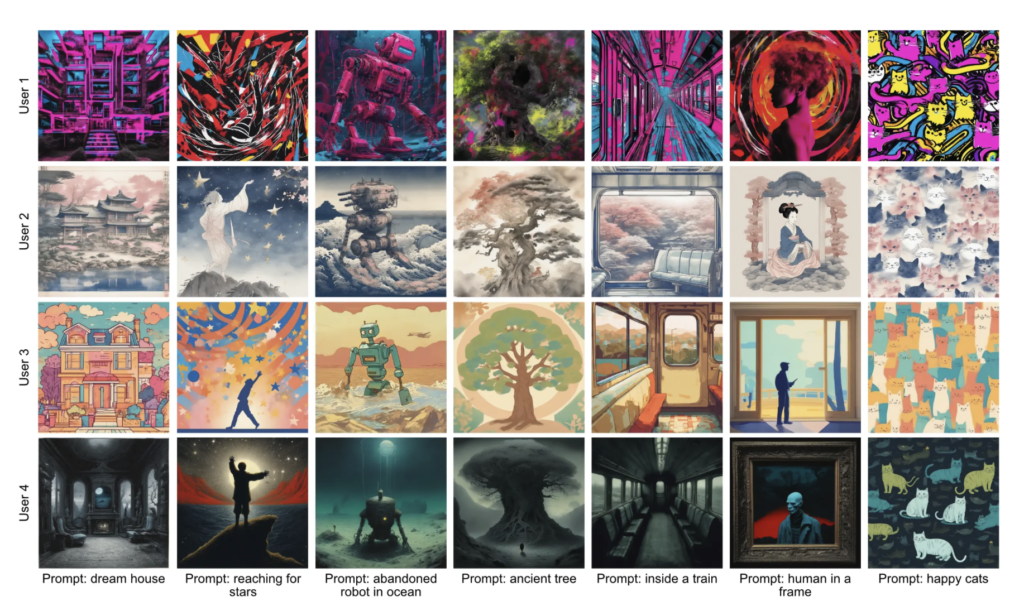
Understanding ViPer
Current generative models are primarily designed to appeal to a broad audience, often resulting in outputs that may not satisfy specific user preferences. Personalizing these models typically involves iterative manual prompt engineering, a time-consuming and often frustrating process. ViPer addresses this inefficiency by introducing a one-time, expressive feedback mechanism to capture a user’s visual preferences.
The process begins with users commenting on a curated selection of images, explaining their likes and dislikes. These comments are then analyzed using a large language model to extract structured visual attributes. These attributes, which can include preferences for surrealism, monochromatic schemes, or rough brushstrokes, are used to guide a text-to-image model like Stable Diffusion, creating images that better align with individual tastes.
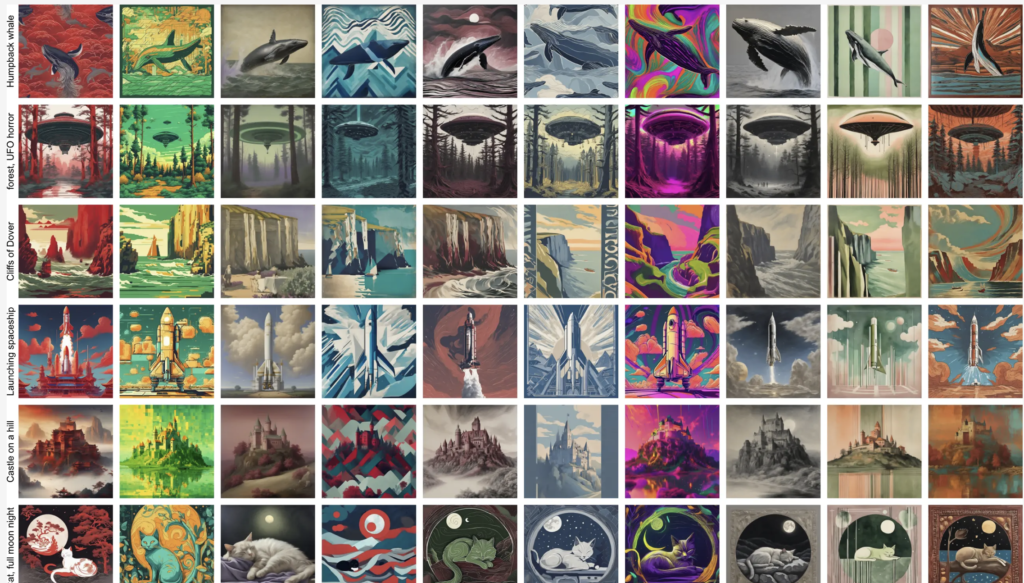
The ViPer Process
ViPer’s methodology involves several key steps:
- User Feedback Collection: Users provide comments on a set of images, detailing what they like or dislike about each.
- Preference Inference: A large language model processes these comments, extracting structured visual attributes that represent the user’s preferences.
- Model Conditioning: These attributes are used to condition a text-to-image model, guiding it to produce images that align with the identified preferences.
This process allows for a highly personalized image generation experience without the need for additional fine-tuning of the model.
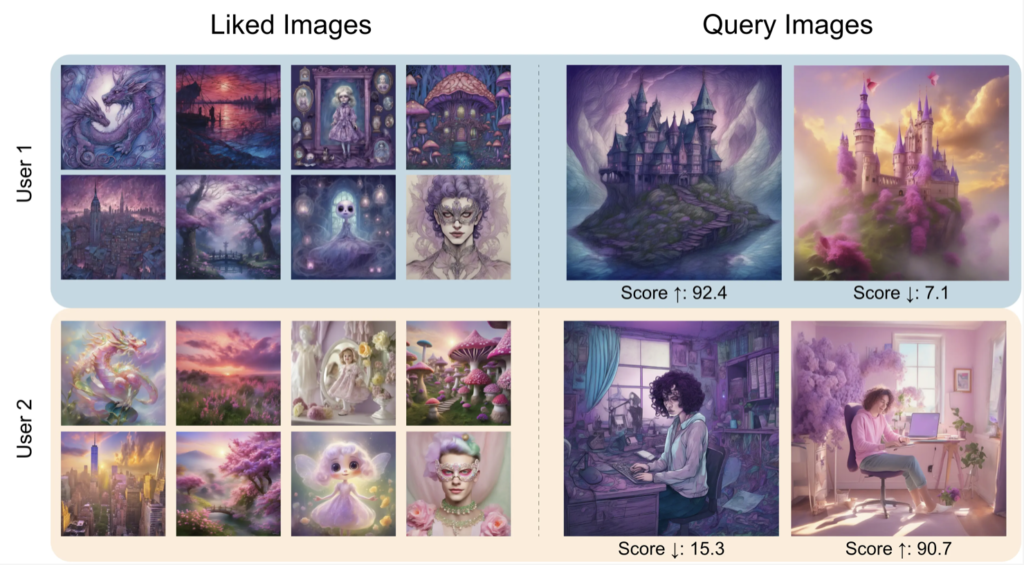
Benefits and Performance
ViPer has demonstrated significant advantages over traditional methods. User studies show that images generated using ViPer’s personalized approach are preferred over those produced by generic models or models personalized using less expressive feedback mechanisms like binary reactions or image rankings.
The approach is also scalable and flexible, capable of accommodating a wide range of visual preferences. ViPer introduces a new metric for automatically assessing personalization, reducing the dependency on costly human evaluations and streamlining the development process.
Challenges and Future Directions
While ViPer marks a significant advancement, there are areas for improvement:
- Expressiveness of User Feedback: While commenting on images is more expressive than ranking, some users may still find it challenging to articulate their preferences. Future work could explore more intuitive feedback mechanisms, such as interpreting brain signals or using more sophisticated proxies.
- Visual Attribute Set: The current implementation relies on a pre-defined set of visual attributes, which may not capture all user preferences. Leveraging more powerful language models like GPT-4 could offer more flexibility, though these models are not open-sourced and can be expensive.
- Limitations of Stable Diffusion: The text encoder in Stable Diffusion has constraints, such as a 77-token limit and sensitivity to word order, which can sometimes overlook preferred attributes. Future improvements could involve using encoders without these limitations to enhance the personalization accuracy.
Additionally, fine-tuning Stable Diffusion based on ViPer’s inferred preferences initially resulted in a decline in image quality. Exploring alternative tuning strategies remains an interesting avenue for future research.
ViPer represents a significant step forward in the personalization of generative models, offering a scalable and effective solution for tailoring AI-generated images to individual tastes. By leveraging user feedback to infer visual preferences and conditioning models accordingly, ViPer enhances the relevance and appeal of AI-generated content. As generative AI continues to evolve, approaches like ViPer will be crucial in ensuring these technologies meet the diverse and specific needs of their users.