Transforming Single RGB Images into Realistic 3D Environments with Component-Aligned Technology
- CAST (Component-Aligned 3D Scene Reconstruction) introduces a groundbreaking method to create high-quality 3D scenes from a single RGB image, overcoming limitations of traditional approaches by focusing on object-level segmentation, spatial relations, and physical consistency.
- By leveraging advanced techniques like occlusion-aware generation, physics-aware corrections, and Signed Distance Fields (SDF), CAST ensures accurate object placement and realistic interactions, enhancing visual and functional realism.
- With applications in gaming, film production, robotics, and immersive content creation, CAST paves the way for innovative virtual environments, though it faces challenges in object detail, lighting, and complex scene handling that future work aims to address.
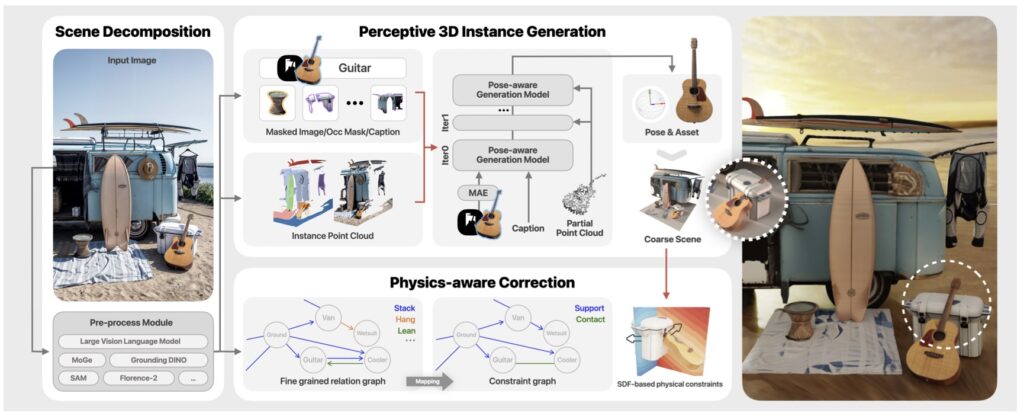
In the realm of computer graphics, reconstructing a detailed 3D scene from a single RGB image has long been a formidable challenge. Traditional methods often falter due to domain-specific constraints or the inability to generate high-quality objects with accurate spatial relationships. Enter CAST—Component-Aligned 3D Scene Reconstruction—a pioneering approach that promises to redefine how we create virtual environments. By dissecting a scene into its fundamental components and reassembling them with precision, CAST offers a glimpse into a future where digital worlds mirror the complexity and coherence of reality. This article explores the intricacies of CAST, its transformative potential, and the hurdles it must overcome to fully realize its promise.
At its core, CAST begins by extracting critical information from a single RGB image, such as object-level 2D segmentation and relative depth data. This initial step lays the groundwork for understanding the scene’s structure. What sets CAST apart is its use of a GPT-based model to analyze inter-object spatial relations, akin to how humans navigate networks of relationships in their social lives. Just as a chair supports a table or a lamp casts shadows on nearby surfaces, objects in a space are interconnected through physical constraints and functional roles. CAST captures these subtle dynamics, ensuring that the reconstructed scene feels cohesive and intuitive, rather than a disjointed collection of items.
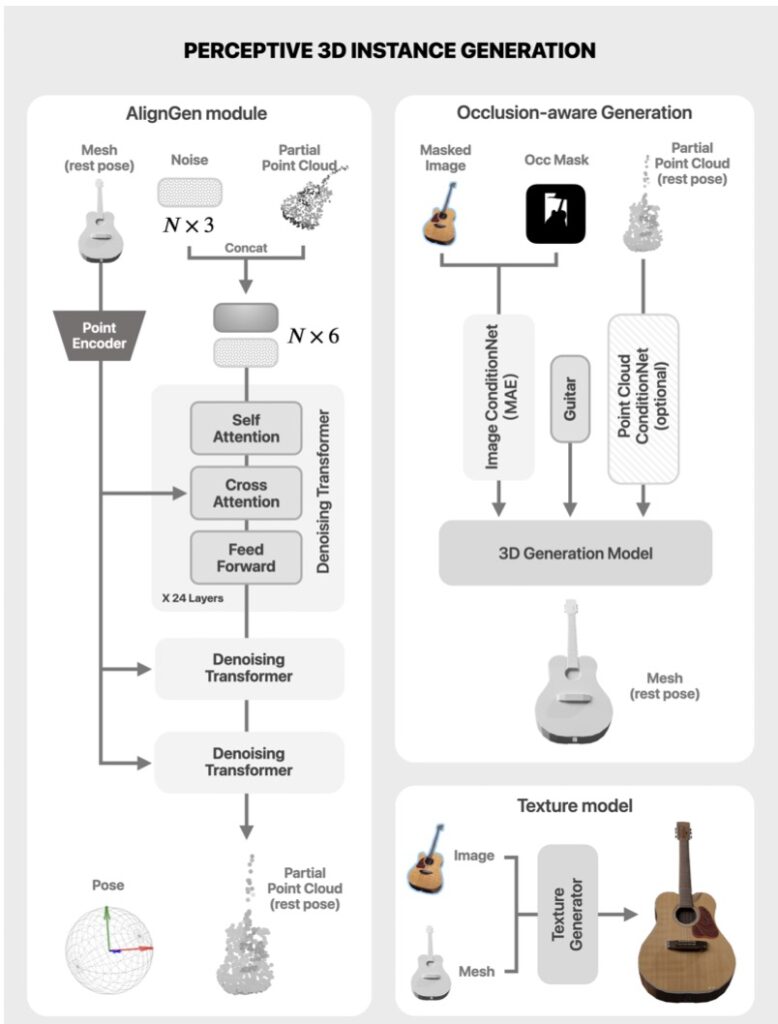
The next phase of CAST’s pipeline is where technical innovation truly shines. Using an occlusion-aware large-scale 3D generation model, CAST independently generates the full geometry of each object. Techniques like Masked Auto Encoder (MAE) and point cloud conditioning help mitigate issues arising from partial occlusions or incomplete data, aligning the generated objects with the source image’s geometry and texture. Furthermore, an alignment generation model calculates the necessary transformations to position each object accurately within the scene’s point cloud. This meticulous process ensures that every element fits seamlessly, avoiding common pitfalls like misaligned poses or unnatural orientations that plague other methods.
Beyond mere placement, CAST incorporates a physics-aware correction mechanism to guarantee physical consistency. By constructing a fine-grained relation graph and a corresponding constraint graph, the system optimizes object poses to reflect real-world interactions. Issues such as object penetration, floating elements, or improper occlusions are addressed through the use of Signed Distance Fields (SDF), resulting in scenes that not only look realistic but also behave as expected under physical laws. Experimental results underscore CAST’s superiority, demonstrating marked improvements in visual quality and spatial coherence compared to state-of-the-art methods. This balance of geometric fidelity and physical plausibility positions CAST as a significant leap forward in single-image 3D reconstruction.
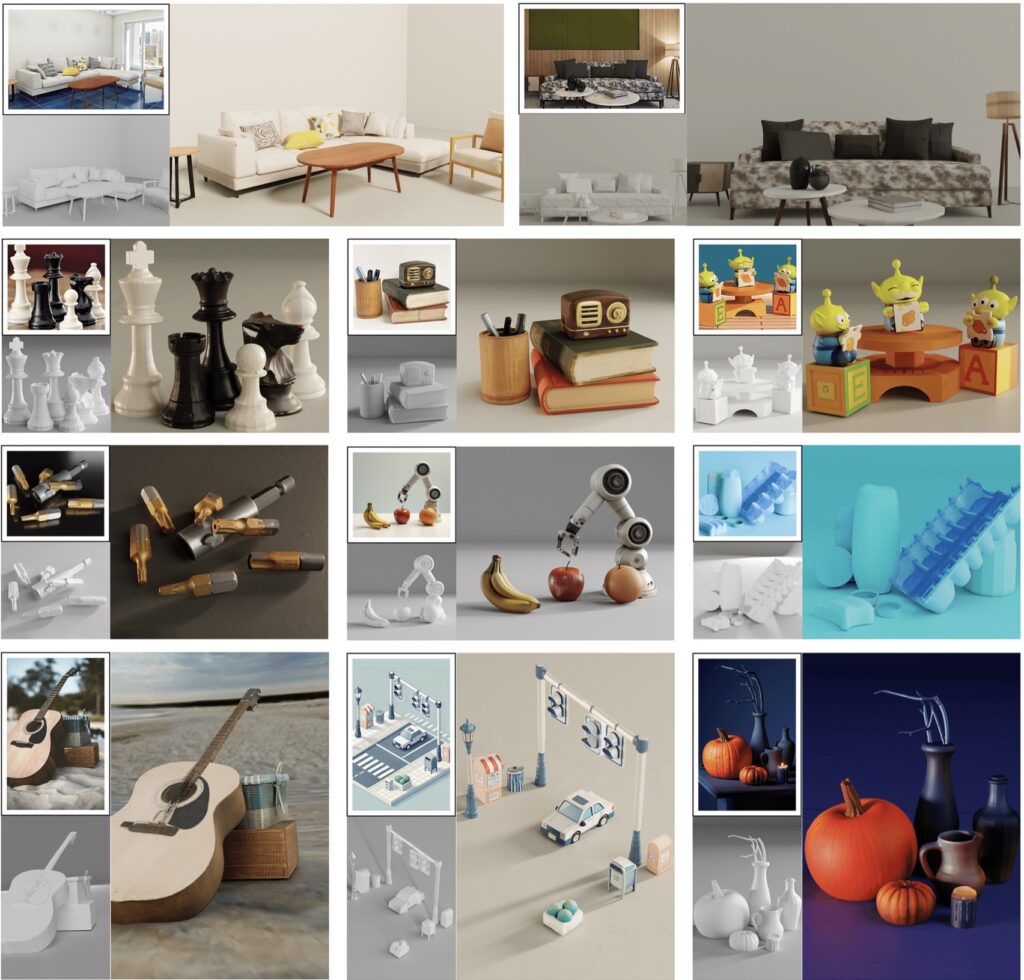
The implications of CAST extend far beyond academic research, offering practical applications across diverse fields. In virtual content creation, such as immersive game environments and film production, CAST enables creators to integrate real-world setups into digital landscapes with unprecedented ease and realism. Similarly, in robotics, CAST facilitates efficient real-to-simulation workflows, providing scalable, realistic environments for testing and training robotic systems. These use cases highlight how CAST can bridge the gap between physical and virtual realms, transforming how we interact with and design digital spaces.
Despite its remarkable achievements, CAST is not without limitations. The quality of its scene generation heavily depends on the underlying object generation model, which currently lacks the detail and precision needed for flawless results. This can lead to inconsistencies in object alignment and spatial relations. Additionally, the mesh representation struggles with materials like textiles, glass, or fabrics, often rendering them unnaturally, and fails to accurately depict transparent surfaces. While supplementary modules have been added to enhance object robustness, the need for more advanced generation models remains a pressing concern.
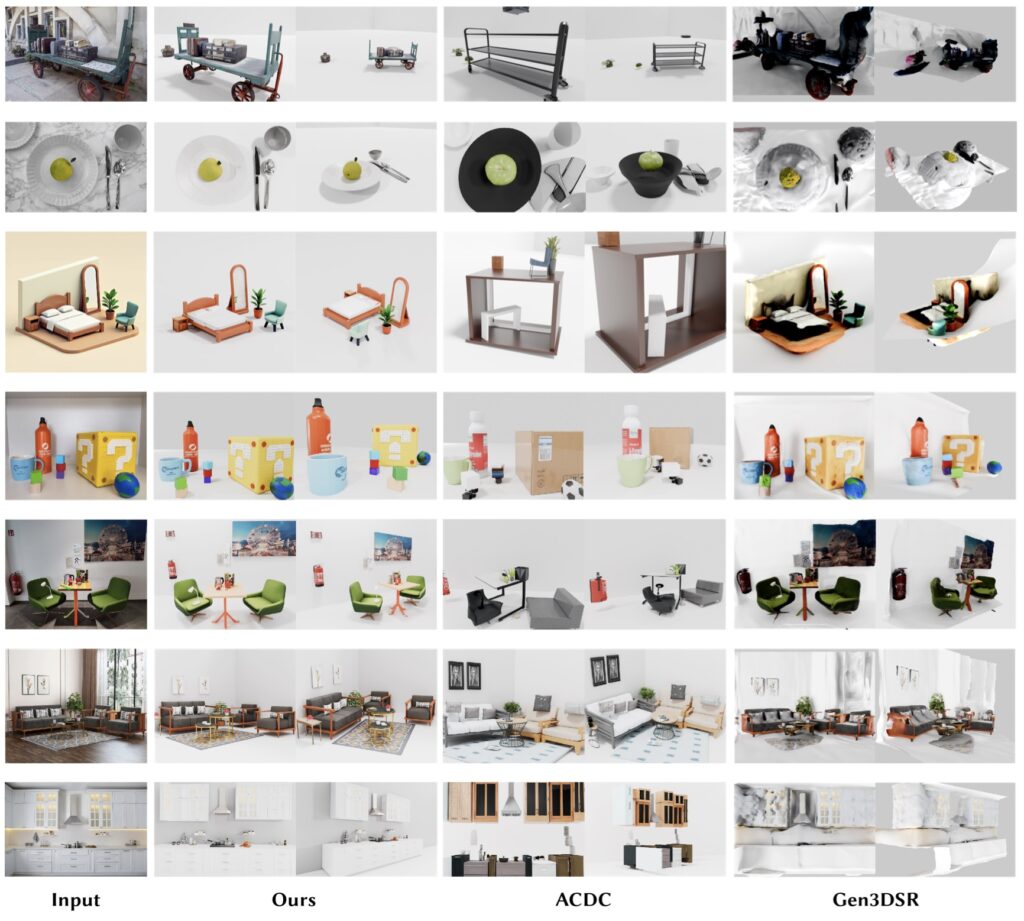
Another notable gap in CAST’s current framework is the absence of lighting estimation and background modeling. Without realistic lighting, the interplay between objects and their surroundings lacks natural shading and illumination, diminishing the overall immersion of the generated environments. To partially address this, CAST employs off-the-shelf tools like panoramic HDR generation combined with preset lighting conditions in software like Blender. Yet, integrating advanced lighting estimation and background modeling in future iterations could significantly elevate the contextual depth and visual fidelity of the scenes.
Complex scenes with intricate spatial layouts or dense object configurations also pose challenges, occasionally leading to slight performance degradation. While CAST excels at reconstructing individual scenes, there is untapped potential in using its outputs to build large-scale datasets for advanced research in fully learned scene or video generation pipelines. Expanding the variety and realism of generated scenes could further bolster the robustness of 3D generative models, opening new avenues in film production, simulation, and immersive media.
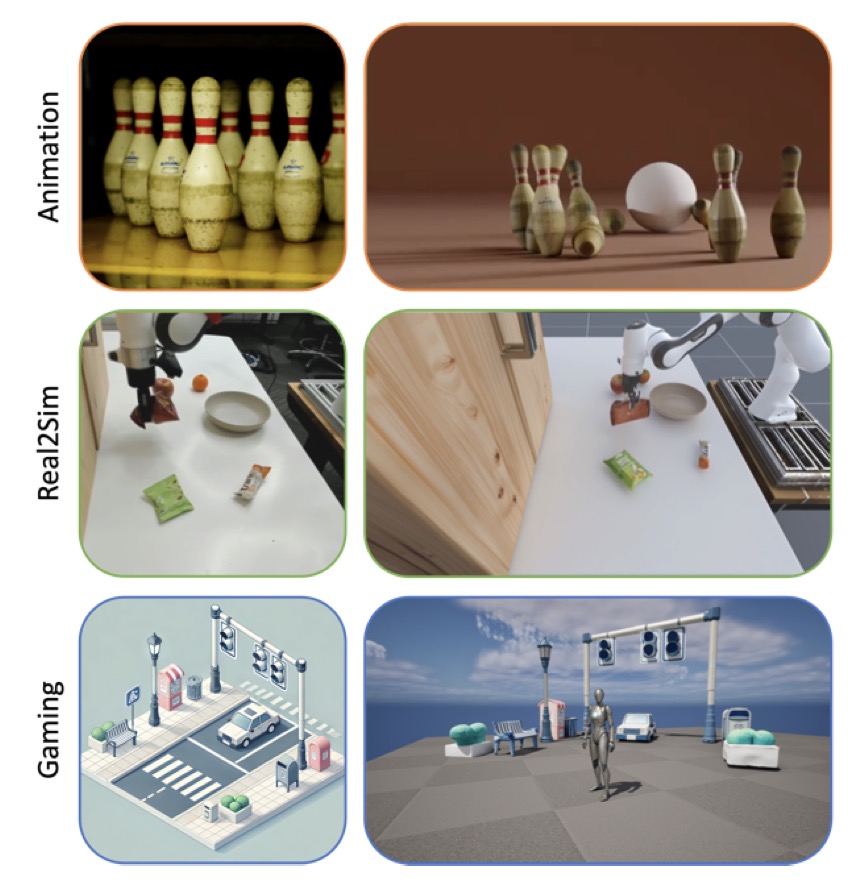
CAST stands as a strong foundation for the future of 3D generation and scene reconstruction. Its structured pipeline, which integrates scene decomposition, perceptive 3D instance generation, and physical correction techniques, addresses longstanding challenges in the field. Through extensive experiments and user studies, CAST has proven its ability to outperform existing methods, setting a new benchmark for visual quality and physical plausibility. As researchers and developers build upon this framework, addressing its current limitations in object detail, lighting, and scalability, CAST could unlock even greater possibilities for creating virtual worlds that are indistinguishable from reality. In a world increasingly reliant on digital environments, CAST is not just a tool—it’s a visionary step toward a more immersive future.