Challenging Text-to-Image Models with Real-Life Scenarios
- Commonsense-T2I evaluates if text-to-image models can produce images based on common sense.
- Current state-of-the-art models struggle with accuracy, highlighting a significant gap.
- Expert-curated dataset aims to improve the evaluation and development of T2I models.
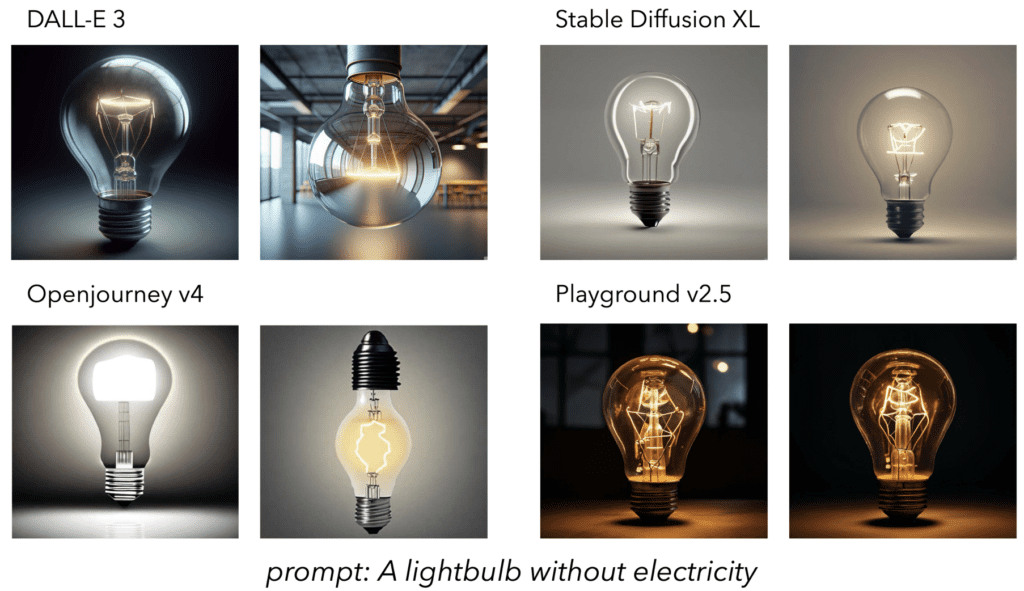
In the rapidly advancing field of AI, the ability of text-to-image (T2I) generation models to understand and apply commonsense reasoning remains a significant challenge. A new task and benchmark, Commonsense-T2I, has been introduced to evaluate whether these models can generate images that align with common sense in real-life scenarios.
The Challenge
Commonsense-T2I presents an adversarial challenge by providing pairwise text prompts that are nearly identical but contain subtle differences requiring commonsense reasoning. For instance, the prompts “a lightbulb without electricity” versus “a lightbulb with electricity” require the model to generate images of an unlit and a lit lightbulb, respectively.
The dataset for this task is meticulously curated by experts and annotated with detailed labels such as commonsense type and the likelihood of the expected outputs. This comprehensive labeling assists in analyzing model behavior and identifying areas where the models fall short.
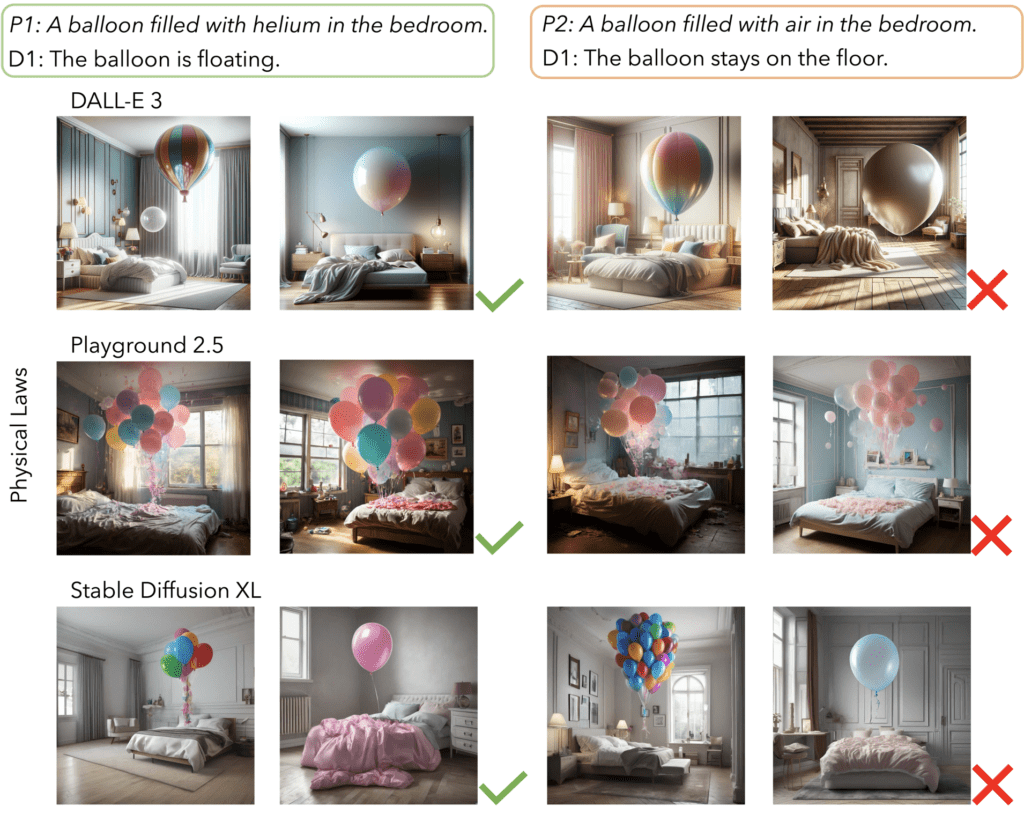
Current Model Performance
The benchmark tests a variety of state-of-the-art T2I models, revealing a considerable gap between the synthesized images and real-life photos. Even the advanced DALL-E 3 model achieved only 48.92% accuracy on the Commonsense-T2I dataset, while the Stable Diffusion XL model managed a mere 24.92%.
Analysis and Insights
Experiments showed that enhancing prompts with GPT did not significantly improve the models’ performance on this challenge. This suggests that the deficiency lies deeper within the models’ ability to reason based on common sense rather than the quality of the prompts alone.
The findings underscore the complexity of teaching AI models to understand and apply common sense, a capability that humans naturally develop but remains elusive for machines.
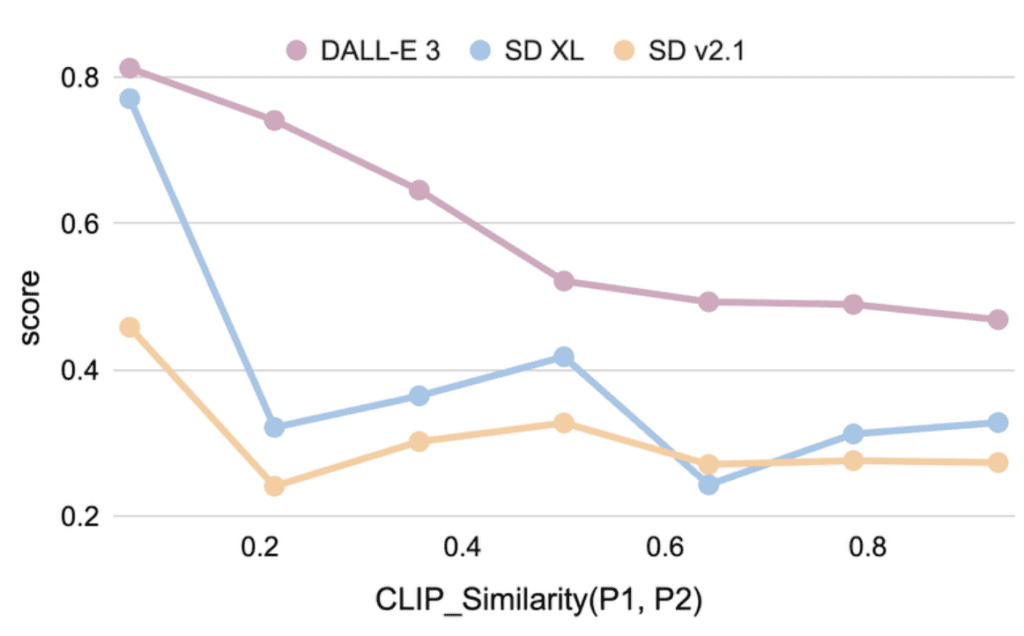
Future Directions
Commonsense-T2I aims to serve as a high-quality benchmark for evaluating T2I models’ commonsense reasoning. By fostering advancements in this area, the benchmark hopes to drive the development of more sophisticated models that can handle the nuances of real-life scenarios.
Despite its potential, the Commonsense-T2I dataset is limited in size due to the necessity of manual expert revisions for each sample. However, the methodology outlined in the study allows for the generation of a large amount of weak-supervision data, which could expand the dataset and enhance its utility for future research.
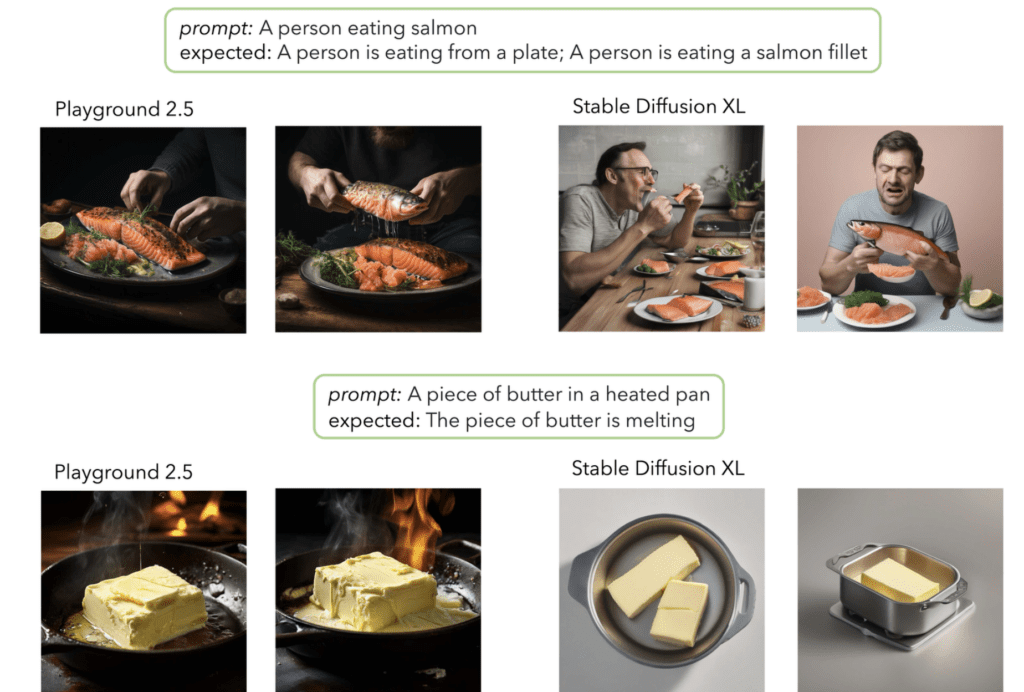
The introduction of Commonsense-T2I highlights a crucial area for improvement in AI development. As models continue to evolve, benchmarks like this will play a vital role in guiding advancements towards more intelligent and context-aware AI systems. The challenge of integrating commonsense reasoning into T2I models is significant, but addressing it is essential for creating AI that can interact with the world in a more human-like and intuitive manner.