Real-Time, Fine-Grained 3D Scene Manipulation Made Possible
- Click-Gaussian enables rapid and accurate segmentation of 3D Gaussians.
- The Global Feature-guided Learning (GFL) method enhances segmentation accuracy.
- The method operates significantly faster than previous approaches, running in 10 ms per click.
Interactive segmentation of 3D Gaussians has long been hampered by slow processing times and imprecise results, limiting its utility in real-time 3D scene manipulation. Enter Click-Gaussian, a novel approach that promises to transform the landscape by offering rapid and precise segmentation. This method leverages the powerful rendering capabilities of 3D Gaussian Splatting while addressing the traditional challenges of noisy outputs and inconsistent feature fields.
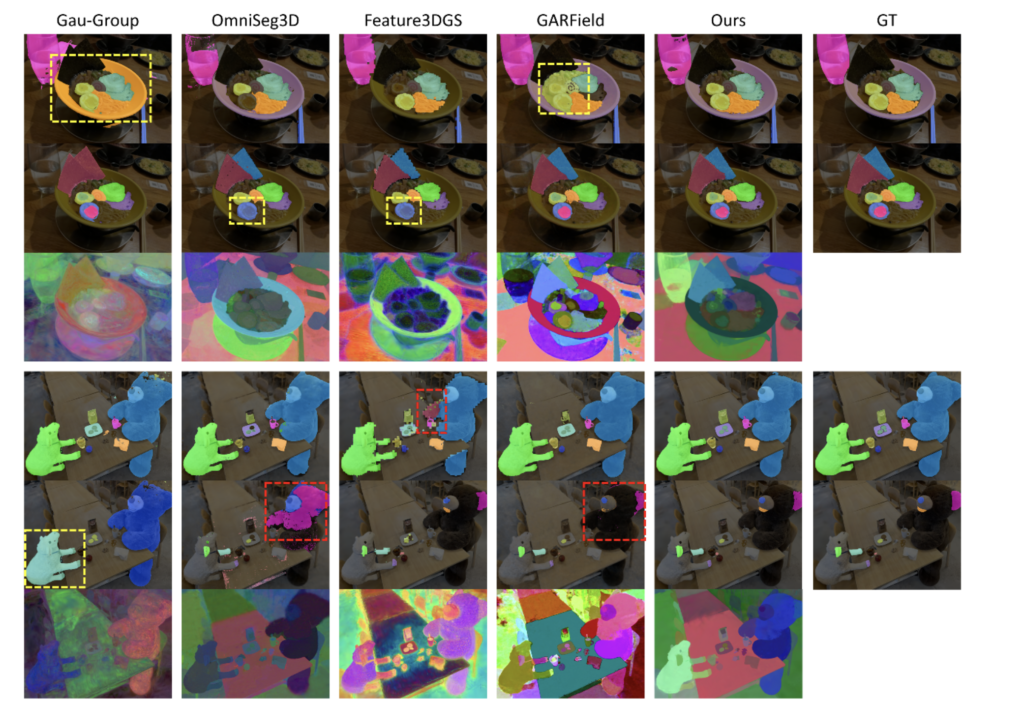
Overcoming Traditional Challenges
Traditional methods for 3D Gaussian segmentation often suffer from time-consuming post-processing steps necessary to clean up noisy outputs. Additionally, they struggle to achieve detailed segmentation required for fine-grained manipulation of 3D scenes. Click-Gaussian tackles these issues head-on by introducing a two-level granularity approach to feature fields, which facilitates more precise segmentation without the need for extensive post-processing.
Global Feature-guided Learning (GFL)
A core innovation of Click-Gaussian is the Global Feature-guided Learning (GFL) method. This technique constructs clusters of global feature candidates from noisy 2D segments across various views, smoothing out inconsistencies during training. By addressing the intrinsic dependency between coarse and fine levels of granularity in real-world objects, GFL ensures that the segmentation process is both accurate and efficient.
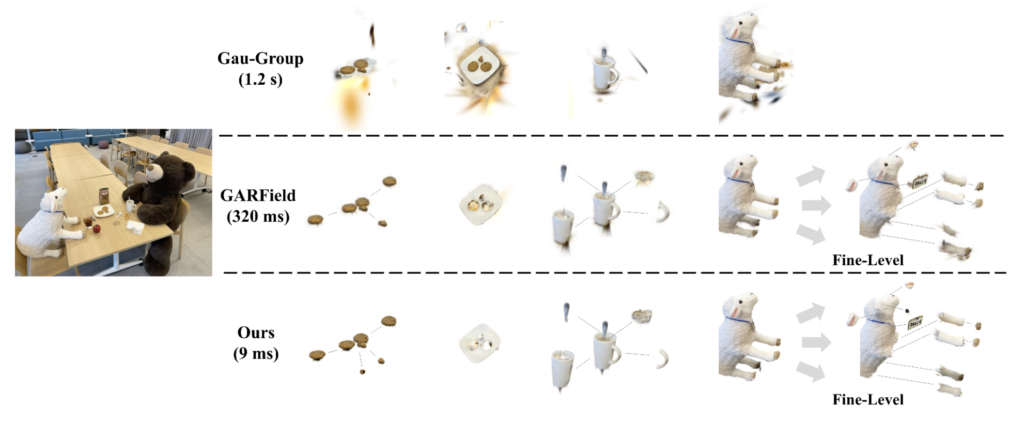
This approach significantly mitigates the problem of cross-view inconsistency in 2D segmentation masks, which has traditionally led to deteriorated 3D segmentation accuracy. With GFL, Click-Gaussian can lift 2D segmentation masks into 3D feature fields more reliably, resulting in improved overall performance.
Performance and Efficiency
Click-Gaussian stands out for its speed and accuracy. The method operates at an impressive rate of 10 milliseconds per click, making it 15 to 130 times faster than previous methods. This speed enables real-time interaction and manipulation of 3D scenes, a crucial requirement for applications in fields such as virtual reality, gaming, and 3D modeling.
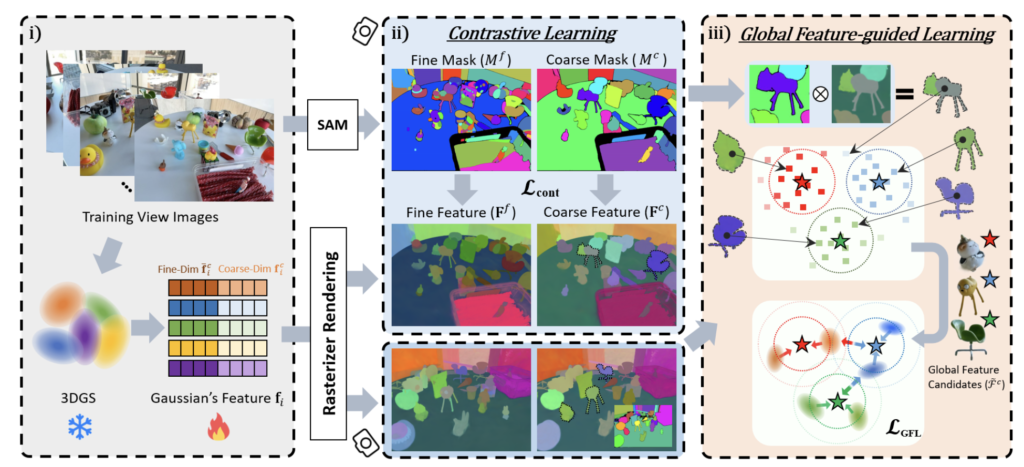
Users can select desired objects at both coarse and fine levels of detail more swiftly and accurately, enhancing their ability to modify 3D environments with precision. This efficiency is achieved without sacrificing the quality of segmentation, thanks to the robust feature learning facilitated by GFL.
Applications and Potential
The enhanced capabilities of Click-Gaussian have significant implications for various applications. In virtual reality, for example, developers can use this method to create more immersive and interactive environments by allowing real-time adjustments to scene elements. In the realm of gaming, designers can benefit from faster and more precise tools for modifying game worlds. Additionally, in 3D modeling and computer-aided design (CAD), Click-Gaussian can streamline workflows by reducing the time and effort required for detailed segmentation tasks.
Limitations and Future Directions
Despite its advantages, Click-Gaussian does have limitations. The method relies on pre-trained 3D Gaussian Splatting (3DGS) and the assumption of a two-level granularity, which might not be suitable for all scenarios. For instance, if a single Gaussian represents multiple objects that are semantically distinct but chromatically similar, feature learning can be hindered. Furthermore, the absence of intermediate levels of granularity might reduce efficiency in handling complex structures, potentially necessitating multiple interactions to achieve the desired segmentation.
Future developments could focus on addressing these limitations by incorporating more flexible granularity levels and improving the adaptability of the method to various object types and scenes. Enhancing the robustness of Click-Gaussian in diverse and challenging environments will further cement its position as a groundbreaking tool for 3D scene manipulation.
Click-Gaussian represents a significant advancement in the field of 3D segmentation, offering unprecedented speed and accuracy. By overcoming the traditional challenges associated with noisy outputs and inconsistent feature fields, this method paves the way for more efficient and precise manipulation of 3D scenes. With its potential applications spanning virtual reality, gaming, and 3D modeling, Click-Gaussian is set to become an invaluable tool for developers and designers looking to push the boundaries of what’s possible in interactive 3D environments.