Probing the Depth and Multiview Consistency of AI-Driven Visual Perception
- 3D Structural Encoding: The study investigates whether visual foundation models not only manage 2D object recognition but also encapsulate the 3D structure of scenes within their representations.
- Viewpoint Consistency Challenges: Despite some ability to maintain surface details across similar viewpoints, the models exhibit limitations in multiview consistency, struggling with significant viewpoint changes.
- Future Directions and Limitations: The findings highlight the need for enhanced 3D understanding in visual models and suggest avenues for future research to achieve more sophisticated spatial awareness.
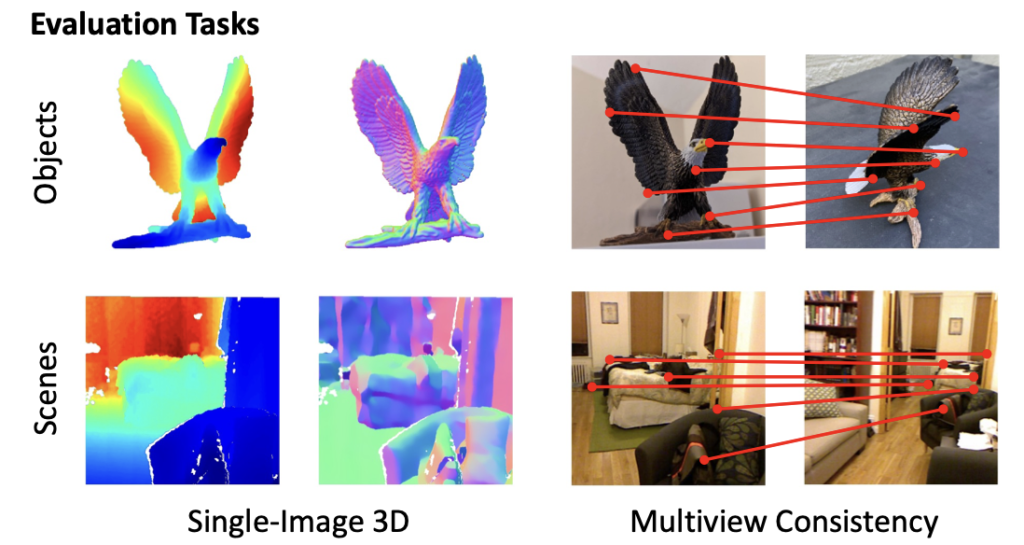
Google’s recent announcement, marks a significant step in understanding the capabilities and limitations of AI in visual processing. This research delves into whether these advanced models, known for their proficiency in various 2D tasks, can truly comprehend and represent the three-dimensional aspects of visual scenes.
Core Analysis
The study’s main objective is to determine the depth and consistency of 3D representations within visual foundation models. Researchers utilized task-specific probes and zero-shot inference methods to evaluate the models’ ability to encode and maintain the geometry of visible surfaces consistently across different views. The evaluation revealed that while the models capture some elements of depth and orientation effectively, they falter when faced with larger changes in viewpoint, indicating a lack of true 3D consistency.
Findings and Implications
- Depth and Orientation: The models generally encode the depth and orientation of objects well, suggesting a solid understanding of some 3D aspects within a limited range of viewpoints.
- Multiview Inconsistency: The struggle with large viewpoint shifts points to a potential over-reliance on view-dependent representations, which may limit the models’ utility in applications requiring robust 3D perceptions, such as augmented reality or robotics.
- Vision-Language Models: A notable exception in the study was vision-language models, which demonstrated superior capabilities in handling semantic correspondences across images, suggesting that integrating linguistic context could enhance spatial understanding.
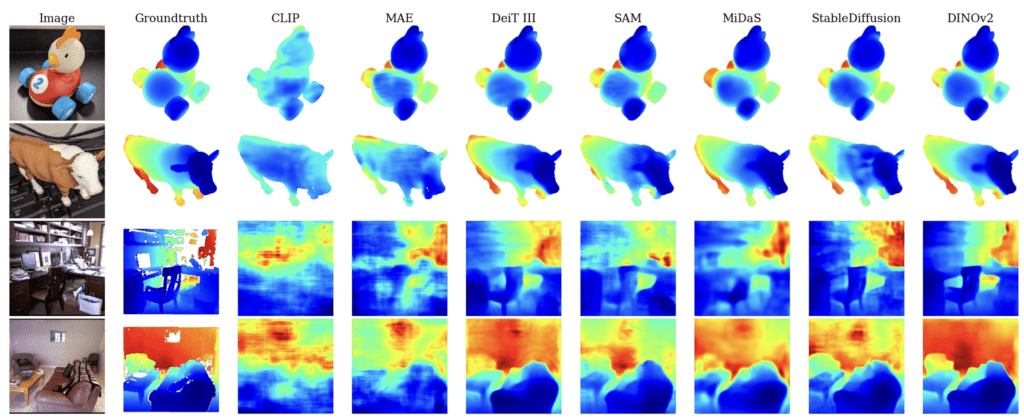
Challenges and Future Research
The study acknowledges several limitations, including the diversity of training datasets and computational resources that could affect the outcomes. Furthermore, the minimal probing approaches used may not fully uncover the complexities of learned representations. Future research is encouraged to employ more comprehensive probing techniques and explore more complex 3D tasks that involve shape perception, spatial reasoning, and dynamic predictions.
Google’s exploration into the 3D awareness of visual foundation models opens up new avenues for enhancing AI’s understanding of the physical world. While current models show promise in basic 3D tasks, significant advancements are needed to achieve true 3D consistency and understanding. This research not only sheds light on current capabilities but also sets the stage for future advancements that could revolutionize how AI perceives and interacts with its environment.