Florence-2 integrates diverse vision and vision-language tasks through a novel prompt-based model.
- Florence-2 utilizes a unified, prompt-based approach for various vision and vision-language tasks.
- The model is trained on FLD-5B, a dataset with 5.4 billion annotations across 126 million images.
- Florence-2 exhibits strong zero-shot and fine-tuning capabilities across multiple vision tasks.
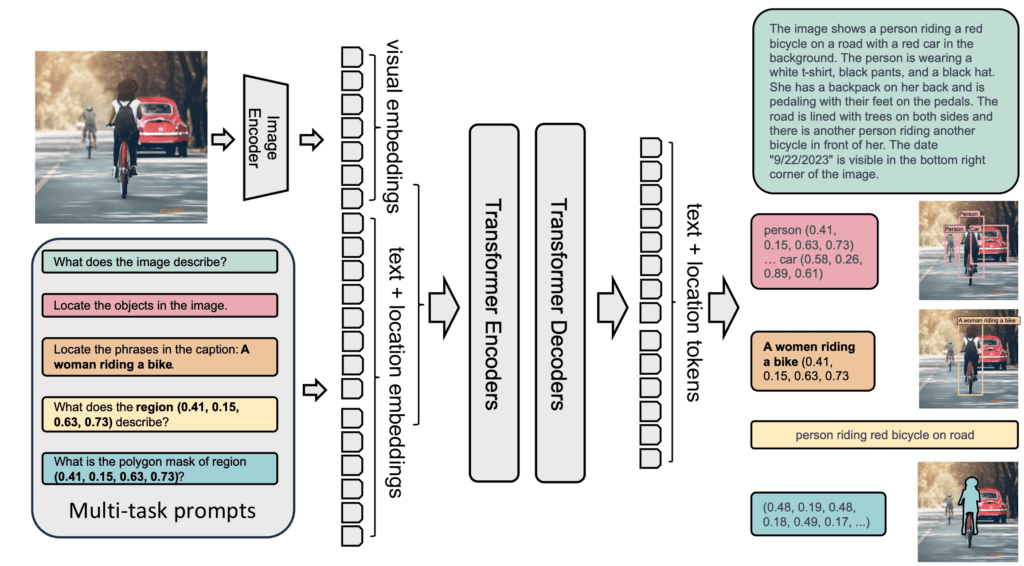
The field of computer vision has taken a significant leap forward with the introduction of Florence-2, a groundbreaking vision foundation model. Developed to tackle a wide range of vision and vision-language tasks, Florence-2 stands out by employing a unified, prompt-based approach. This new model addresses the limitations of existing large vision models, which, while strong in transfer learning, often struggle with diverse task instructions involving varying spatial hierarchies and semantic granularity.
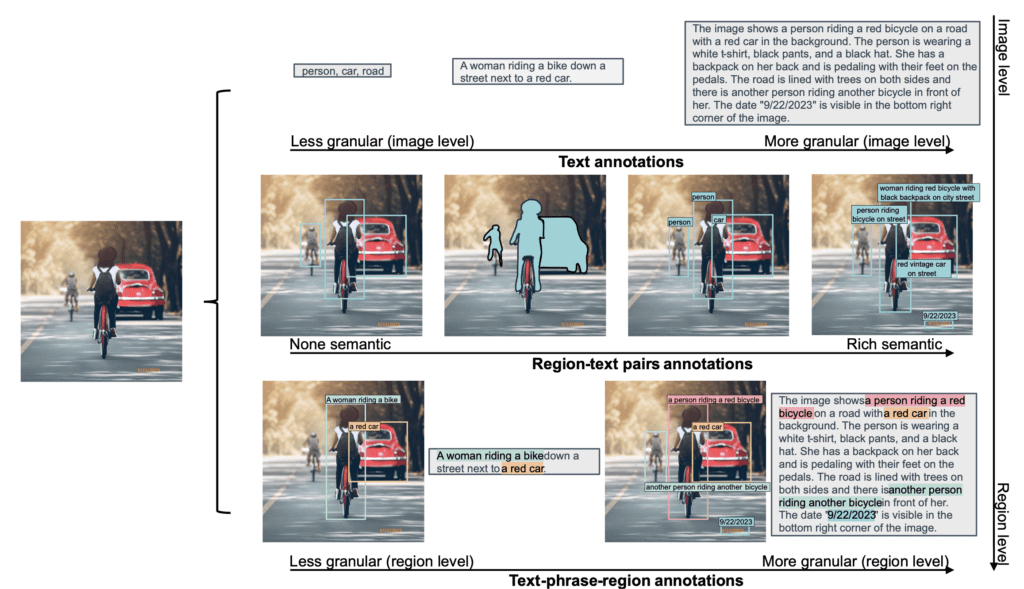
Unified Prompt-Based Vision Model
Florence-2 is designed to interpret text prompts as task instructions, generating appropriate text-based results. This could range from image captioning and object detection to visual grounding and segmentation. Such a multifaceted learning setup necessitates a vast amount of high-quality annotated data. To meet this need, the developers created FLD-5B, a massive dataset comprising 5.4 billion comprehensive visual annotations spread across 126 million images. This dataset was built using an iterative process combining automated image annotation and continuous model refinement.
Training and Capabilities
Florence-2 is trained using a sequence-to-sequence structure to handle the wide array of vision tasks. Extensive evaluations have demonstrated that Florence-2 excels in both zero-shot and fine-tuning scenarios. This makes it a formidable contender in the realm of vision foundation models, showcasing unprecedented versatility and performance across multiple tasks.
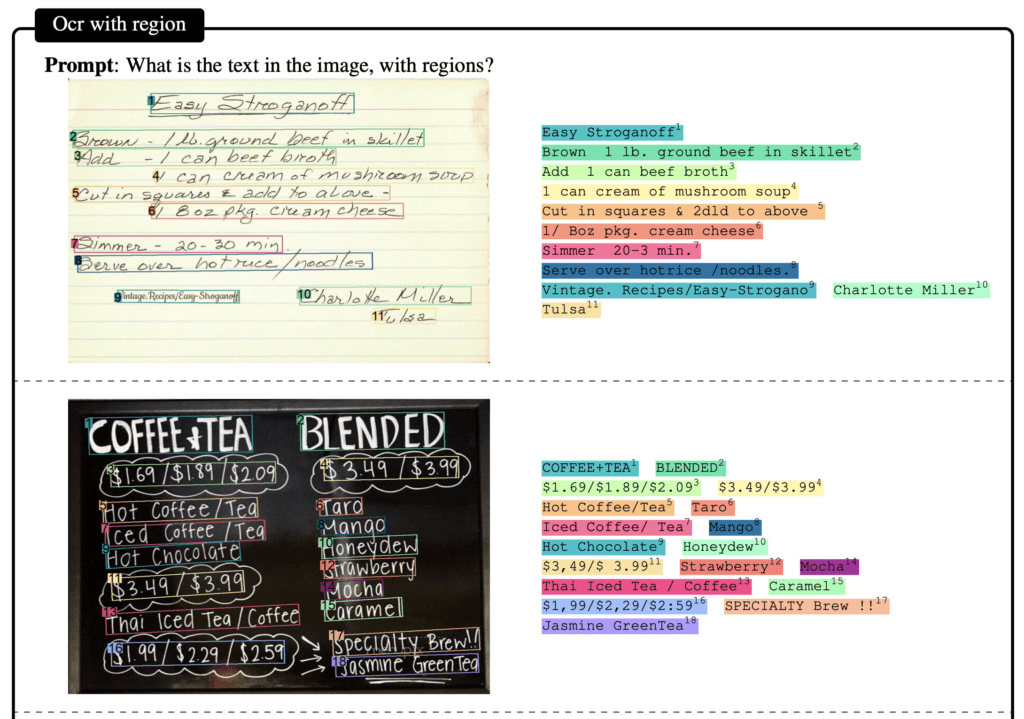
In the context of Artificial General Intelligence (AGI), there has been a noticeable trend towards utilizing pre-trained, versatile representations. This trend, prominent in natural language processing (NLP), has seen advanced models demonstrate adaptability with comprehensive knowledge across various domains and tasks through simple instructions. Inspired by these successes, the Florence Project aims to develop a foundational vision model endowed with diverse perceptual capabilities, handling both spatial hierarchy and semantic granularity.
The FLD-5B Dataset
The creation of FLD-5B was a monumental effort, involving 126 million images paired with 5 billion comprehensive annotations. These annotations were collected through the Florence data engine, which uses a sophisticated process of automated image annotation followed by model refinement. This dataset forms the backbone of Florence-2, enabling its comprehensive multitask learning in a unified manner.
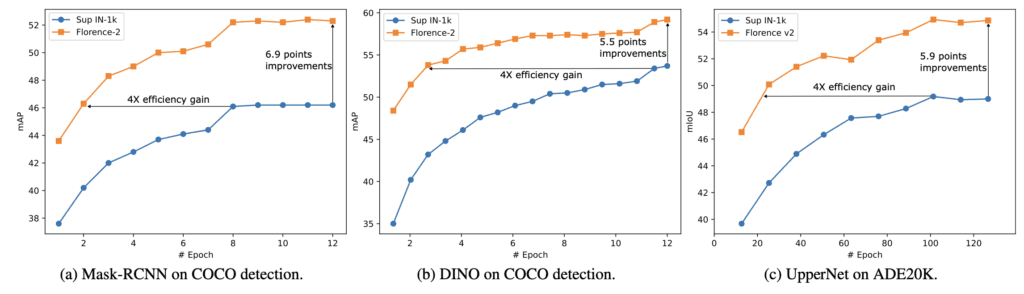
Performance and Applications
Florence-2’s capabilities extend across a wide spectrum of visual tasks, such as captioning, object detection, visual grounding, and referring segmentation. The model’s performance in these areas highlights the strength of its universal representation, which has been pre-trained on the extensive FLD-5B dataset. Experimental results underscore the model’s effectiveness, revealing substantial improvements in a multitude of downstream tasks.
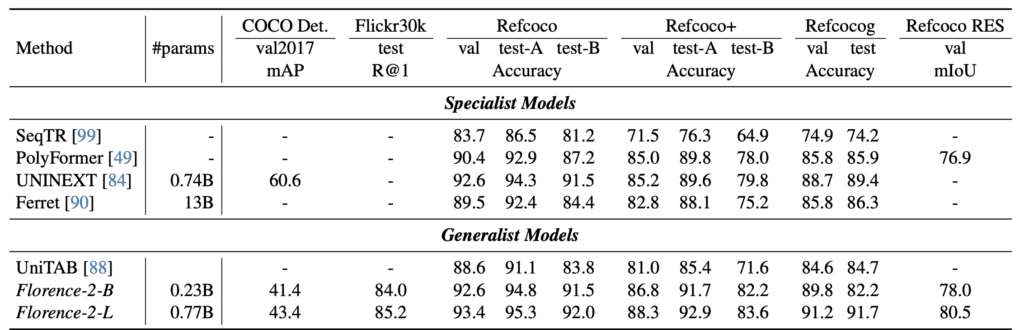
Florence-2 represents a significant advancement in computer vision, bridging the gap between task-specific models and a truly unified vision model. By leveraging a massive, high-quality dataset and an innovative training approach, Florence-2 sets a new standard for vision and vision-language tasks. This model not only demonstrates exceptional zero-shot and fine-tuning capabilities but also paves the way for future advancements in the field, potentially driving the next wave of innovation in AI-driven visual understanding.