Novel approach leverages counterfactual datasets and bootstrap supervision to achieve groundbreaking results in object removal and insertion within images.
- Innovative Counterfactual Dataset: ObjectDrop utilizes a unique dataset composed of before-and-after images of scenes with a single object removed, enhancing the realism of object manipulation in images.
- Bootstrap Supervision for Expansion: To overcome the limitations of a small dataset for object insertion, ObjectDrop employs bootstrap supervision, synthetically expanding the dataset to improve model performance.
- Superior Performance in Object Manipulation: The approach significantly outperforms existing methods, adeptly handling complex image elements like shadows and reflections for both object removal and insertion.
The advent of diffusion models has marked a significant leap forward in image editing capabilities, yet these models often struggle with maintaining physical consistency, particularly when manipulating objects within a scene. Recognizing the limitations of self-supervised approaches in handling occlusions, shadows, and reflections accurately, a novel method named ObjectDrop has been introduced, which centers on the concept of a “counterfactual” dataset.
Counterfactual Dataset as a Foundation
ObjectDrop’s methodology hinges on the creation of a counterfactual dataset, which involves photographing scenes before and after the removal of a specific object, with minimal other changes. This dataset, comprising 2,500 images, serves as a crucial foundation for fine-tuning a diffusion model to not only remove objects from images but also effectively eliminate their associated effects, such as shadows and reflections.
Overcoming Dataset Limitations with Bootstrap Supervision
While ObjectDrop’s object removal capabilities are impressive, the challenge of photorealistic object insertion proved more daunting due to the need for a substantially larger dataset. To address this, ObjectDrop introduces a method of bootstrap supervision, where the object removal model is used to generate additional synthetic data from a large unsupervised dataset. This process expands the initial dataset to 350,000 images, facilitating the training of a robust object insertion model capable of synthesizing complex image elements.
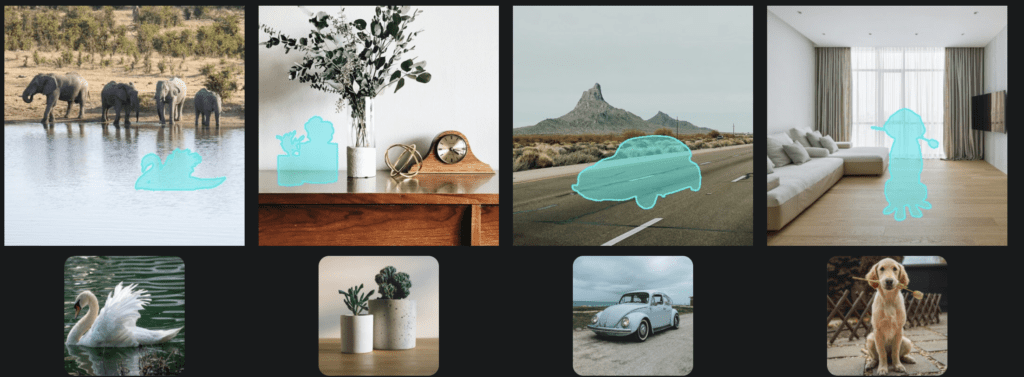
Achieving Unprecedented Realism in Image Editing
The integration of ObjectDrop’s object removal and insertion models allows for seamless manipulation of objects within images, setting a new standard in photorealistic image editing. The method demonstrates remarkable generalization capabilities, adeptly removing large objects from diverse scenes and synthesizing realistic shadows and reflections for inserted objects.
By leveraging a counterfactual dataset and bootstrap supervision, ObjectDrop offers a practical and effective solution to the challenges of photorealistic object manipulation in image editing. This approach not only advances the field of image editing but also opens up new possibilities for applications in various domains, from content creation to virtual staging and beyond. With ObjectDrop, the future of image editing looks more realistic and versatile than ever before.